Weakly Supervised Hand Pose Recovery With Domain Adaptation By Low-Rank Alignment
IEEE Conference Proceedings(2016)
摘要
Human hand pose recovery (HPR) in depth images is usually conducted by constructing mappings between 2D depth images and 3D hand poses. It is a challenging task since the feature spaces of 2D images and 3D poses are different. Therefore, a large number of labeled data is required for training, especially for popular frameworks such as deep learning. In this paper, we propose an HPR method with weak supervision. It is based on neural network and domain adaptation is introduced to enhance the trained model. To achieve domain adaptation, we propose low-rank alignment, which aligns the testing samples to the distribution of labeled samples. In this process, autoencoders are used to extract 2D image features and low- rank representation is used to describe this feature space. Therefore, the proposed method is named as Domain Adaptation with Low-Rank Alignment (DALA). In this way, we obtain a robust and non-linear mapping from 2D images to 3D poses. Experiments are conducted on two challenging benchmark datasets MSRA and ICVL. Both the results on a single dataset and across datasets show the outstanding performance of DALA.
更多查看译文
关键词
Human hand pose recovery,neural network,domain adaptation,low-rank representation
AI 理解论文
溯源树
样例
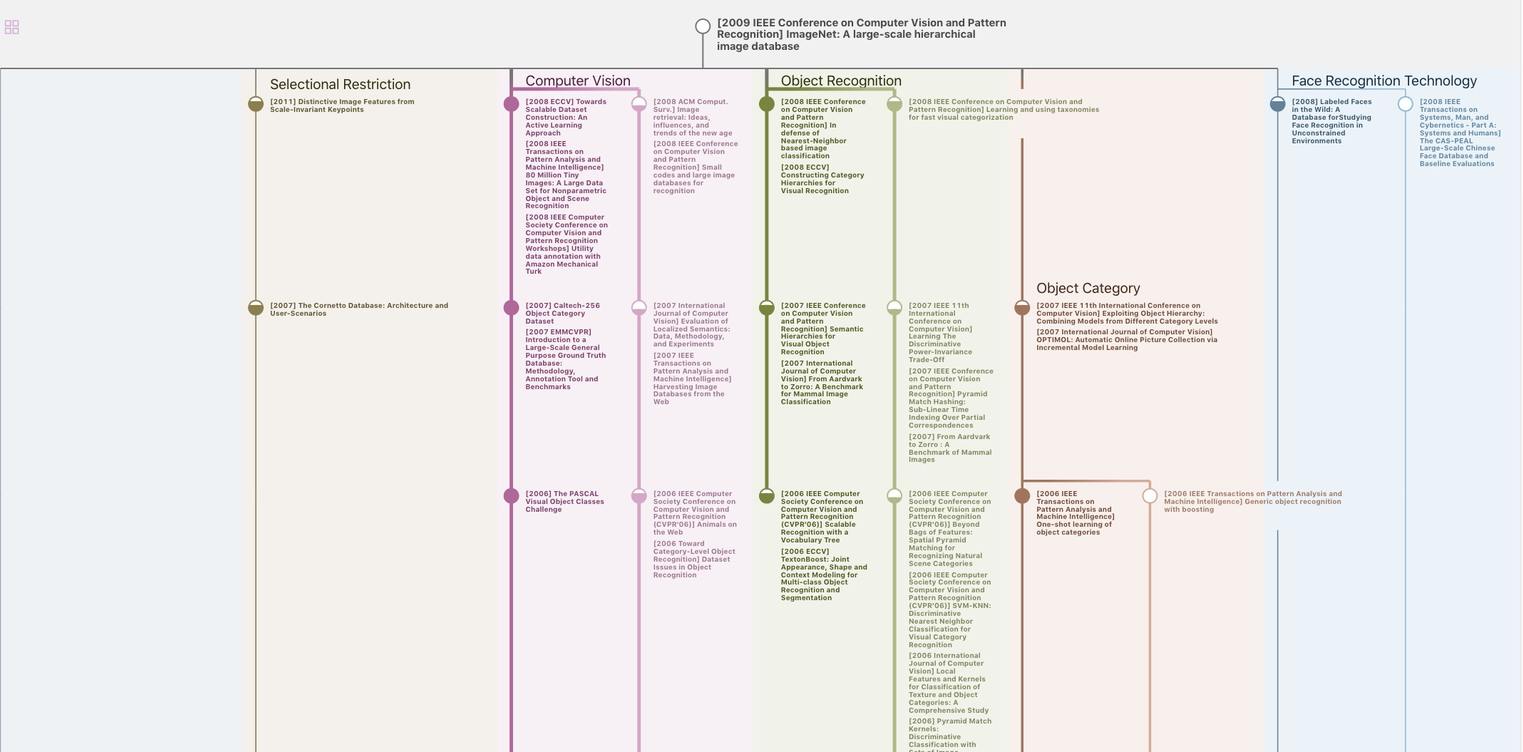
生成溯源树,研究论文发展脉络
Chat Paper
正在生成论文摘要