Data-Driven Methods Towards Learning The Highly Nonlinear Inverse Kinematics Of Tendon-Driven Surgical Manipulators
INTERNATIONAL JOURNAL OF MEDICAL ROBOTICS AND COMPUTER ASSISTED SURGERY(2017)
摘要
BackgroundAccurate motion control of flexible surgical manipulators is crucial in tissue manipulation tasks. The tendon-driven serpentine manipulator (TSM) is one of the most widely adopted flexible mechanisms in minimally invasive surgery because of its enhanced maneuverability in torturous environments. TSM, however, exhibits high nonlinearities and conventional analytical kinematics model is insufficient to achieve high accuracy.MethodsTo account for the system nonlinearities, we applied a data driven approach to encode the system inverse kinematics. Three regression methods: extreme learning machine (ELM), Gaussian mixture regression (GMR) and K-nearest neighbors regression (KNNR) were implemented to learn a nonlinear mapping from the robot 3D position states to the control inputs.ResultsThe performance of the three algorithms was evaluated both in simulation and physical trajectory tracking experiments. KNNR performed the best in the tracking experiments, with the lowest RMSE of 2.1275mm.ConclusionsThe proposed inverse kinematics learning methods provide an alternative and efficient way to accurately model the tendon driven flexible manipulator.
更多查看译文
关键词
data-driven methods, inverse kinematics, surgical robotics, tendon-driven serpentine manipulator
AI 理解论文
溯源树
样例
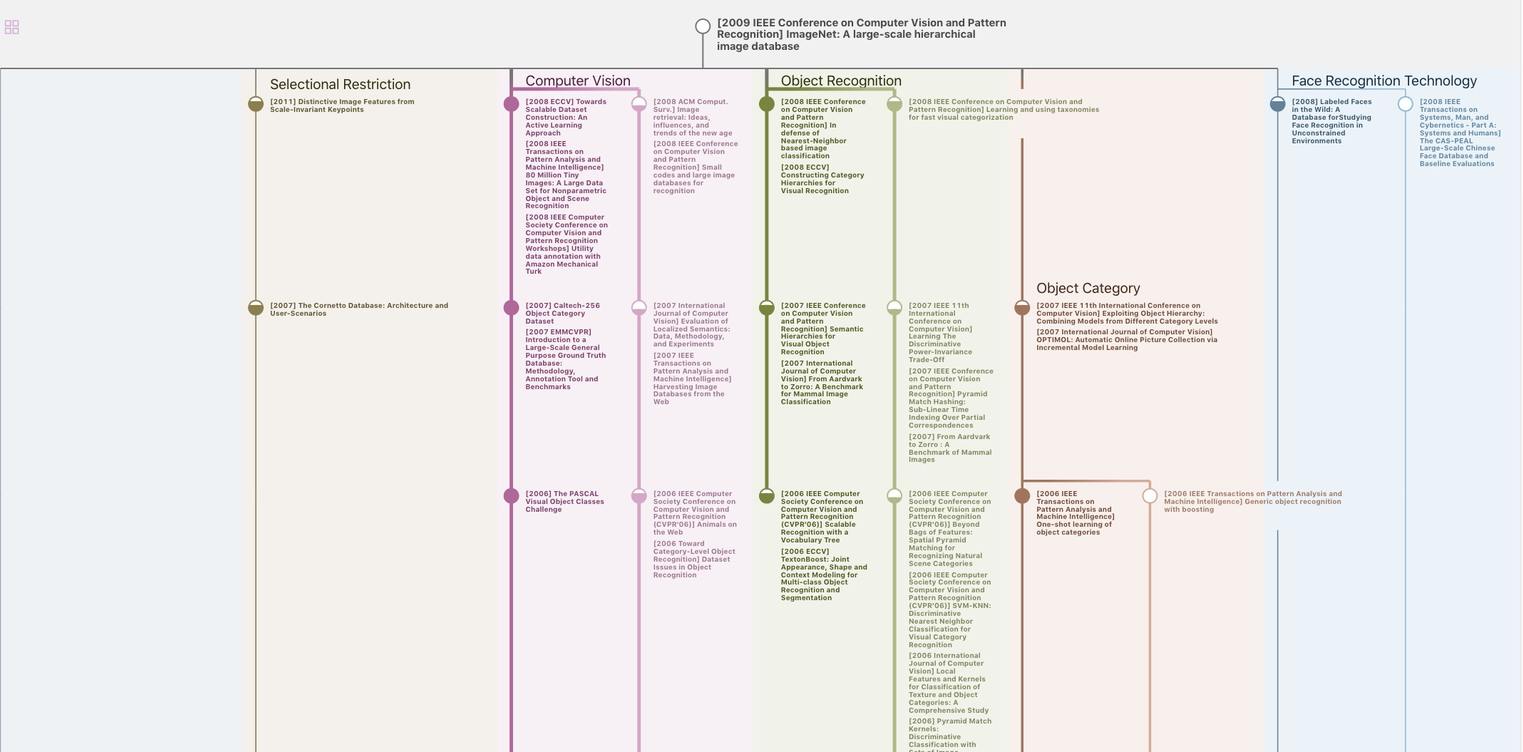
生成溯源树,研究论文发展脉络
Chat Paper
正在生成论文摘要