An Analysis of Value Function Learning with Piecewise Linear Control.
Journal of experimental and theoretical artificial intelligence(2015)
摘要
Reinforcement learning (RL) algorithms attempt to learn optimal control actions by iteratively estimating a long-term measure of system performance, the so-called value function. For example, RL algorithms have been applied to walking robots to examine the connection between robot motion and the brain, which is known as embodied cognition. In this paper, RL algorithms are analysed using an exemplar test problem. A closed form solution for the value function is calculated and this is represented in terms of a set of basis functions and parameters, which is used to investigate parameter convergence. The value function expression is shown to have a polynomial form where the polynomial terms depend on the plant's parameters and the value function's discount factor. It is shown that the temporal difference error introduces a null space for the differenced higher order basis associated with the effects of controller switching (saturated to linear control or terminating an experiment) apart from the time of the switch. This leads to slow convergence in the relevant subspace. It is also shown that badly conditioned learning problems can occur, and this is a function of the value function discount factor and the controller switching points. Finally, a comparison is performed between the residual gradient and TD(0) learning algorithms, and it is shown that the former has a faster rate of convergence for this test problem.
更多查看译文
关键词
polynomial basis,trajectory null space,badly conditioned learning,rate of convergence,temporal difference learning parameter convergence
AI 理解论文
溯源树
样例
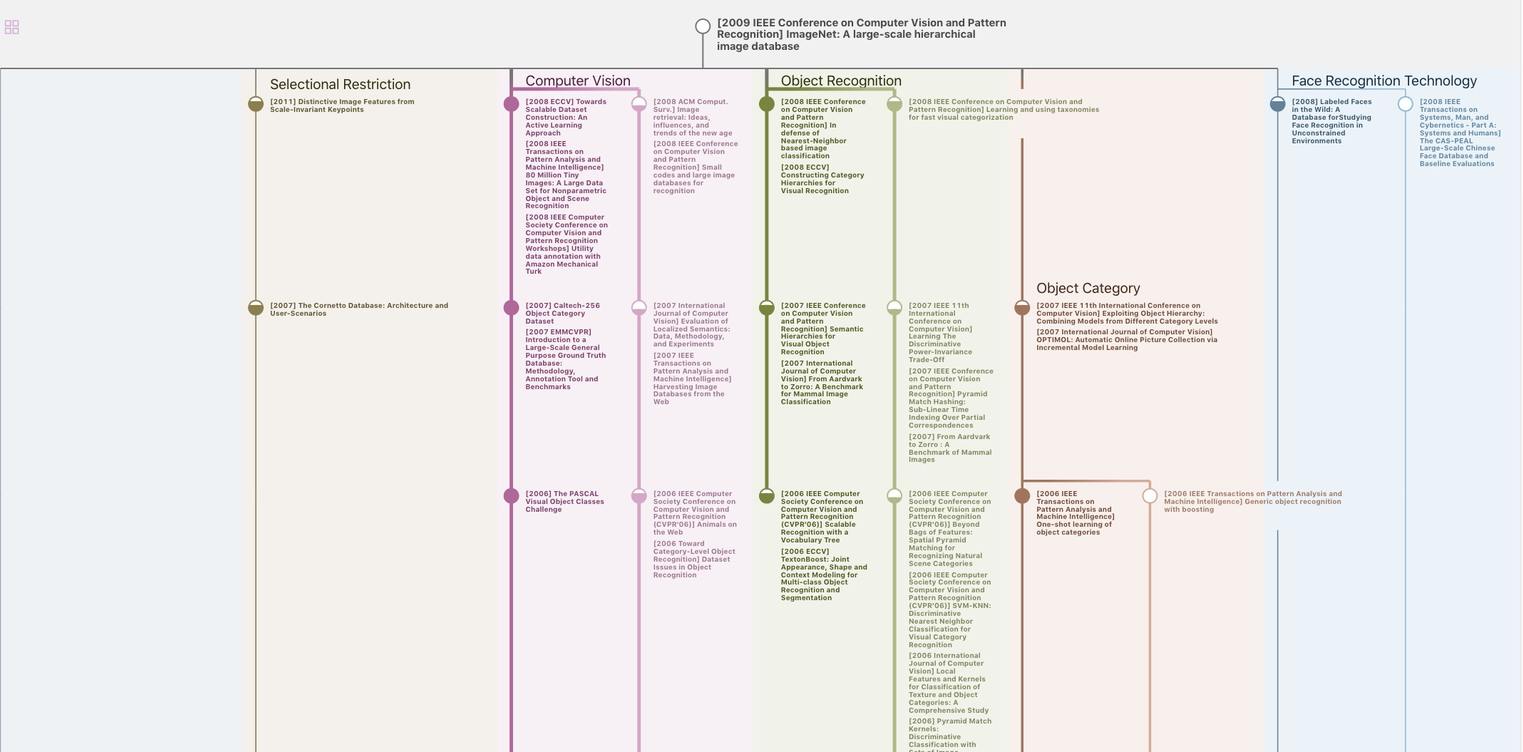
生成溯源树,研究论文发展脉络
Chat Paper
正在生成论文摘要