Efficient Algorithm for Sparse Coding and Dictionary Learning with Applications to Face Recognition
Journal of electronic imaging(2015)
摘要
Sparse representation has been successfully applied to pattern recognition problems in recent years. The most common way for producing sparse coding is to use the I-1-norm regularization. However, the I-1-norm regularization only favors sparsity and does not consider locality. It may select quite different bases for similar samples to favor sparsity, which is disadvantageous to classification. Besides, solving the I-1-minimization problem is time consuming, which limits its applications in large-scale problems. We propose an improved algorithm for sparse coding and dictionary learning. This algorithm takes both sparsity and locality into consideration. It selects part of the dictionary columns that are close to the input sample for coding and imposes locality constraint on these selected dictionary columns to obtain discriminative coding for classification. Because an analytic solution of the coding is derived by only using part of the dictionary columns, the proposed algorithm is much faster than the I-1-based algorithms for classification. Besides, we also derive an analytic solution for updating the dictionary in the training process. Experiments conducted on five face databases show that the proposed algorithm has better performance than the competing algorithms in terms of accuracy and efficiency. (C) 2015 SPIE and IS&T
更多查看译文
关键词
sparse representation,dictionary learning,face recognition
AI 理解论文
溯源树
样例
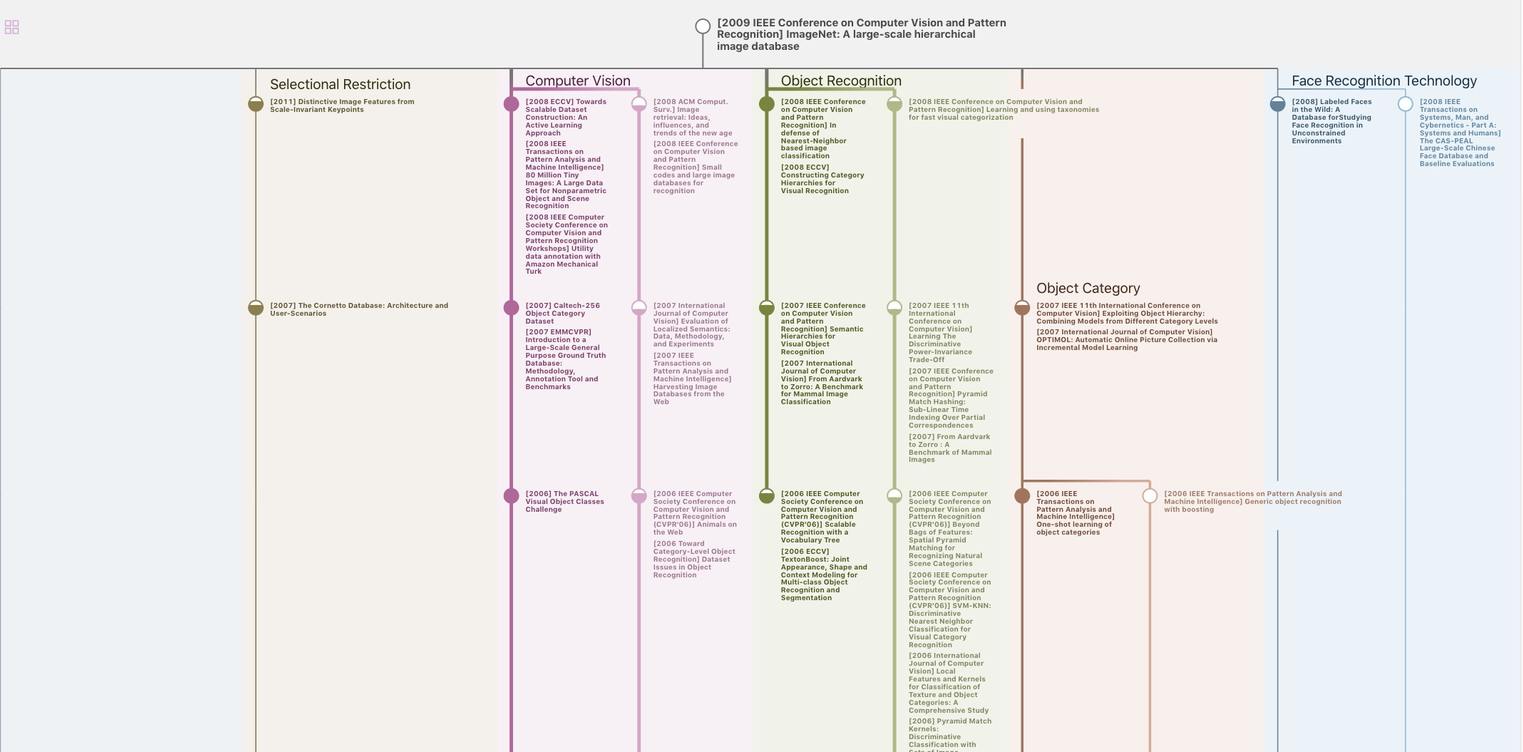
生成溯源树,研究论文发展脉络
Chat Paper
正在生成论文摘要