A Unified Framework of Surrogate Loss by Refactoring and Interpolation
Computer Vision – ECCV 2020 Lecture Notes in Computer Science(2020)
摘要
We introduce UniLoss, a unified framework to generate surrogate losses for training deep networks with gradient descent, reducing the amount of manual design of task-specific surrogate losses. Our key observation is that in many cases, evaluating a model with a performance metric on a batch of examples can be refactored into four steps: from input to real-valued scores, from scores to comparisons of pairs of scores, from comparisons to binary variables, and from binary variables to the final performance metric. Using this refactoring we generate differentiable approximations for each non-differentiable step through interpolation. Using UniLoss, we can optimize for different tasks and metrics using one unified framework, achieving comparable performance compared with task-specific losses. We validate the effectiveness of UniLoss on three tasks and four datasets. Code is available at https://github.com/princeton-vl/uniloss.
更多查看译文
关键词
Loss design,Image classification,Pose estimation
AI 理解论文
溯源树
样例
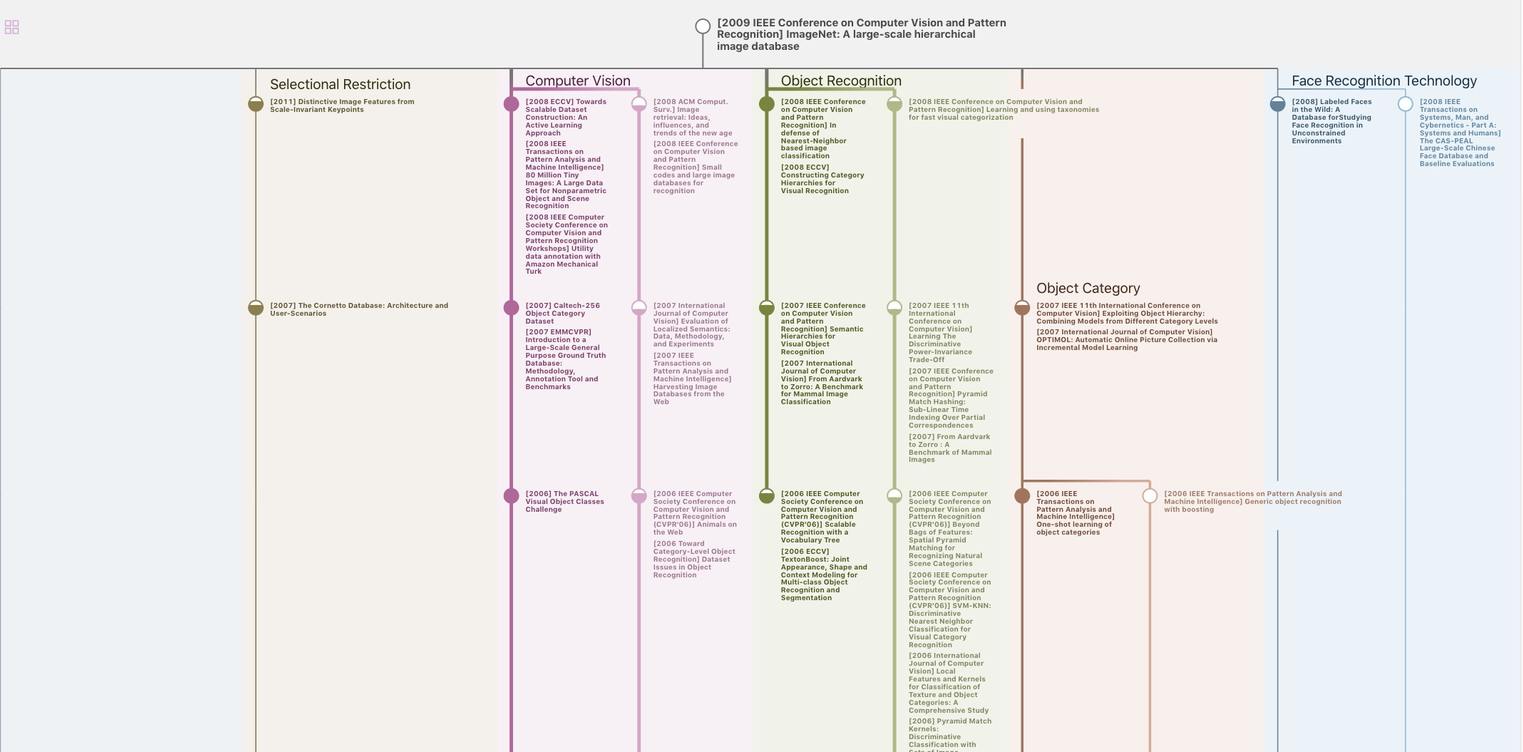
生成溯源树,研究论文发展脉络
Chat Paper
正在生成论文摘要