Automated structural resilience evaluation based on a multi-scale Transformer network using field monitoring data
Mechanical Systems and Signal Processing(2025)
摘要
Structural resilience evaluation is crucial for ensuring structural safety, with structural damage detection (SDD) serving as a core component. Although convolutional neural networks (CNNs) have been proven effective in extracting damage-sensitive features for SDD, their limited receptive field and weak global information processing during feature extraction can lead to insufficient accuracy and reduced stability when dealing with field monitoring data. To tackle these issues, a multi-scale Transformer network called Transformer neural network with multi-scale acceleration feature fusion (TMAFF) is presented for enhanced SDD results. Both overlap patch embedding and dilated overlap patch embedding (DOPE) are employed to acquire feature representations for acceleration at different scales respectively, which reserve more features in acceleration signals and thereby improve the method’s robustness against noisy or incompletely measured data. DOPE employs dilated convolution to enlarge the receptive field of convolutional layers without increasing additional parameters. Additionally, an improved Transformer block, integrating cross-channel correlation-based multi-head attention (CCMA) and gated depth-wise separable convolution feedforward network mechanisms, is included for automated extraction of damage-sensitive features. The use of CCMA improves calculating efficiency and spatial correlation consideration compared to traditional self-attention-based Transformers. Furthermore, employing the GELU activation function enhances the neural network’s nonlinear modeling and complex data distribution fitting capabilities, thereby improving the accuracy and stability of SDD. Numerical simulations conducted on a five-storey rigid frame indicates that TMAFF outperforms CNN in feature extraction, providing more reliable accuracy for SDD. Validations using field monitoring data from both an experimental frame and the Yonghe Bridge demonstrate the feasibility of the proposed method for structural resilience evaluation, even with a limited number of sensors available.
更多查看译文
关键词
Structural resilience evaluation,Structural damage detection,Field monitoring,Multi-scale Transformer network,Dilated overlap patch embedding
AI 理解论文
溯源树
样例
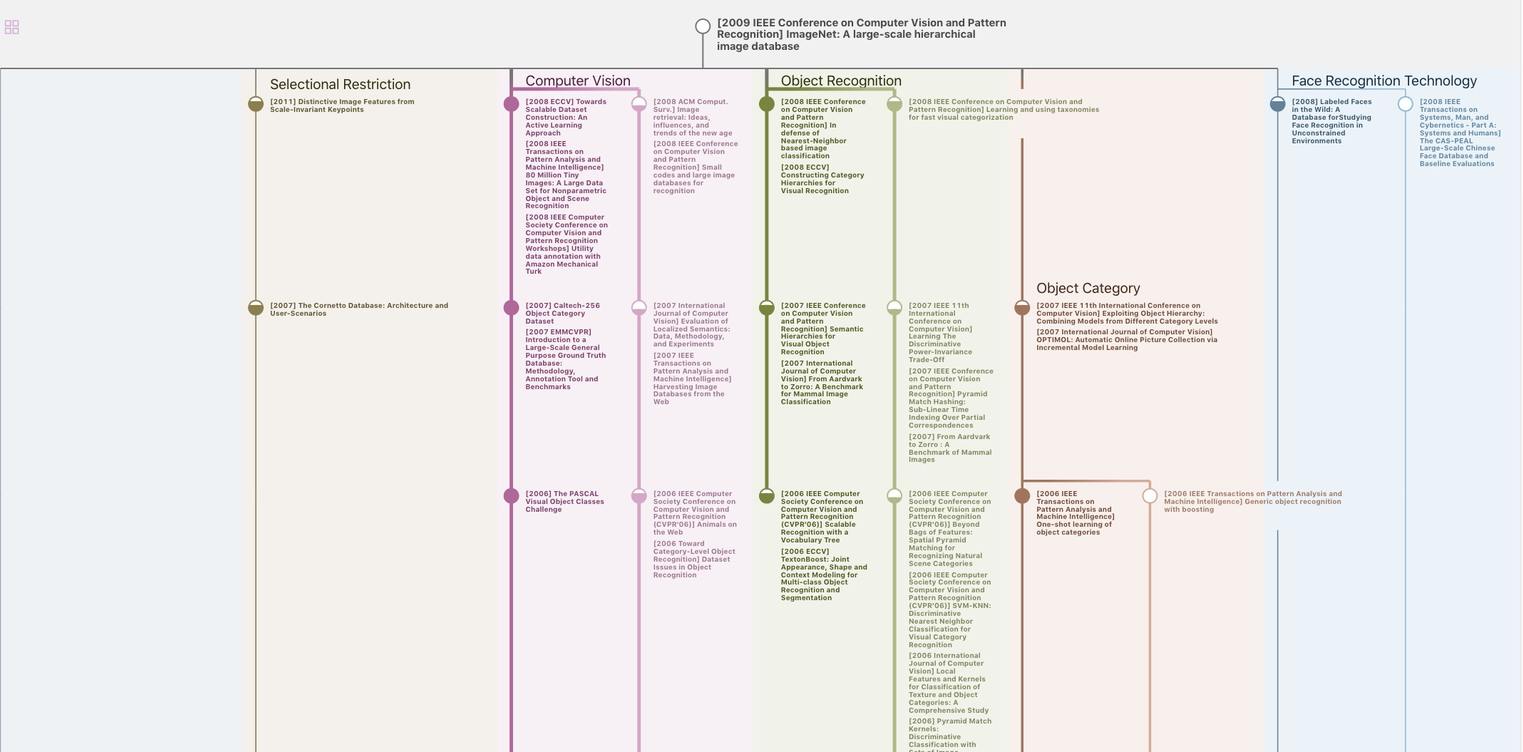
生成溯源树,研究论文发展脉络
Chat Paper
正在生成论文摘要