Privacy-preserving Cancelable Multi-Biometrics for Identity Information Management
Information Processing & Management(2025)
摘要
Biometrics have copious merits over traditional authentication schemes and promote information management. The demand for large-scale biometric identification and certification booms. In spite of enhanced efficiency and scalability in cloud-based biometrics, they suffer from compromised privacy during the transmission and storage of irrevocable biometric information. Existing biometric protection strategies fatally degrade the recognition performance, due to two folds: inherent drawbacks of uni-biometrics and inevitable information loss caused by over-protection. Hence, how to make a trade-off between performance and protection is an alluring challenge. To settle these issues, we are the first to present a cancelable multi-biometric system combining iris and periocular traits with recognition performance improved and privacy protection emphasized. Our proposed binary mask-based cross-folding integrates multi-instance and multi-modal fusion tactics. Further, the steganography based on a low-bit strategy conceals sensitive biometric fusion into QR code with transmission imperceptible. Subsequently, a fine-grained hybrid attention dual-path network through stage-wise training models inter-class separability and intra-class compactness to extract more discriminative templates for biometric fusion. Afterward, the random graph neural network transforms the template into the protection domain to generate the cancelable template versus the malicious. Experimental results on two benchmark datasets, namely IITDv1 and MMUv1, show the proposed algorithm attains promising performance against state-of-the-art approaches in terms of equal error rate. What is more, extensive privacy analysis demonstrates prospective irreversibility, unlinkability, and revocability, respectively.
更多查看译文
关键词
Cloud-based identity information management,Multi-biometric system,Cancelable template,Deep learning
AI 理解论文
溯源树
样例
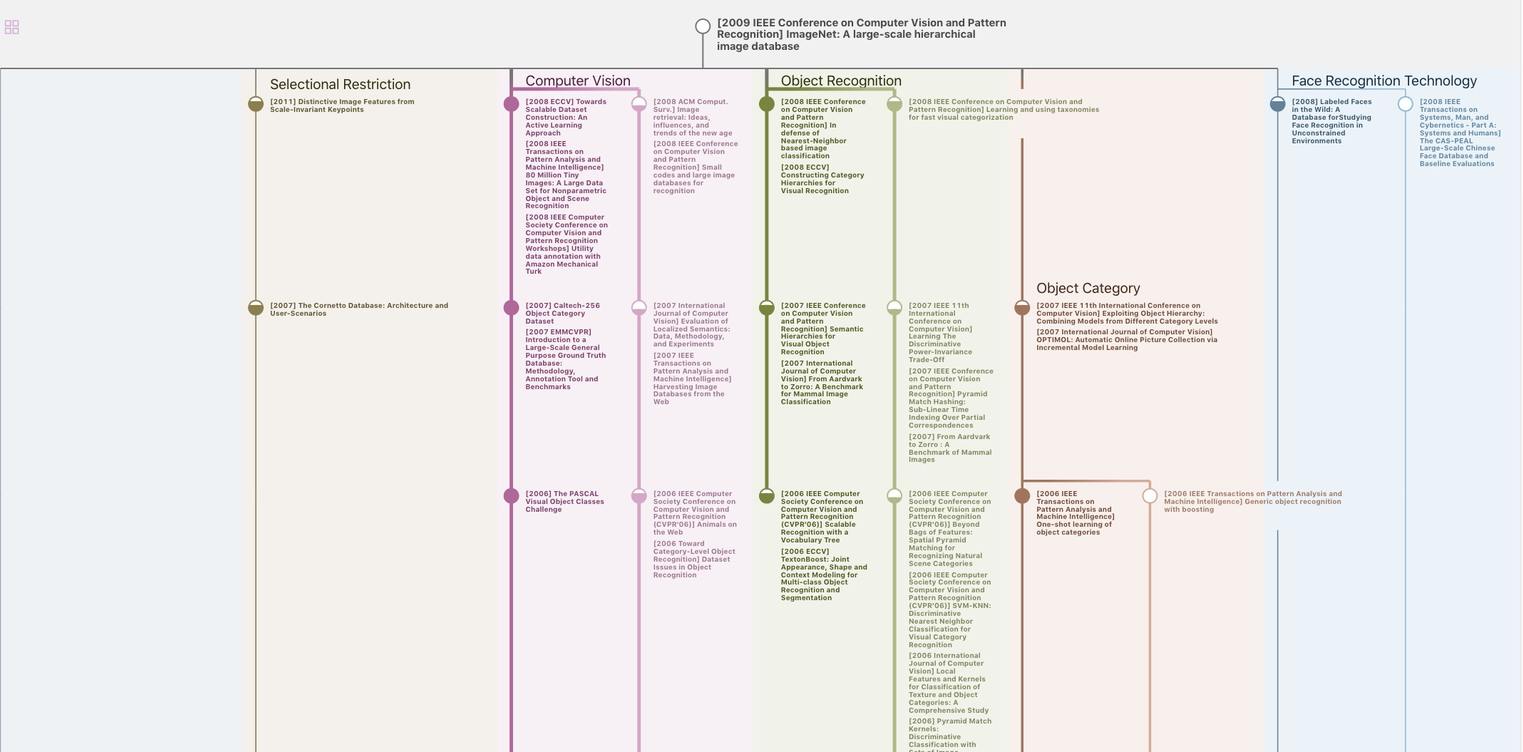
生成溯源树,研究论文发展脉络
Chat Paper
正在生成论文摘要