Cross-Attention Regression Flow for Defect Detection
IEEE transactions on image processing a publication of the IEEE Signal Processing Society(2024)
摘要
Defect detection from images is a crucial and challenging topic of industry scenarios due to the scarcity and unpredictability of anomalous samples. However, existing defect detection methods exhibit low detection performance when it comes to small-size defects. In this work, we propose a Cross-Attention Regression Flow (CARF) framework to model a compact distribution of normal visual patterns for separating outliers. To retain rich scale information of defects, we build an interactive cross-attention pattern flow module to jointly transform and align distributions of multi-layer features, which is beneficial for detecting small-size defects that may be annihilated in high-level features. To handle the complexity of multi-layer feature distributions, we introduce a layer-conditional autoregression module to improve the fitting capacity of data likelihoods on multi-layer features. By transforming the multi-layer feature distributions into a latent space, we can better characterize normal visual patterns. Extensive experiments on four public datasets and our collected industrial dataset demonstrate that the proposed CARF outperforms state-of-the-art methods, particularly in detecting small-size defects.
更多查看译文
关键词
Defect detection,Normalizing Flows,Autoregression
AI 理解论文
溯源树
样例
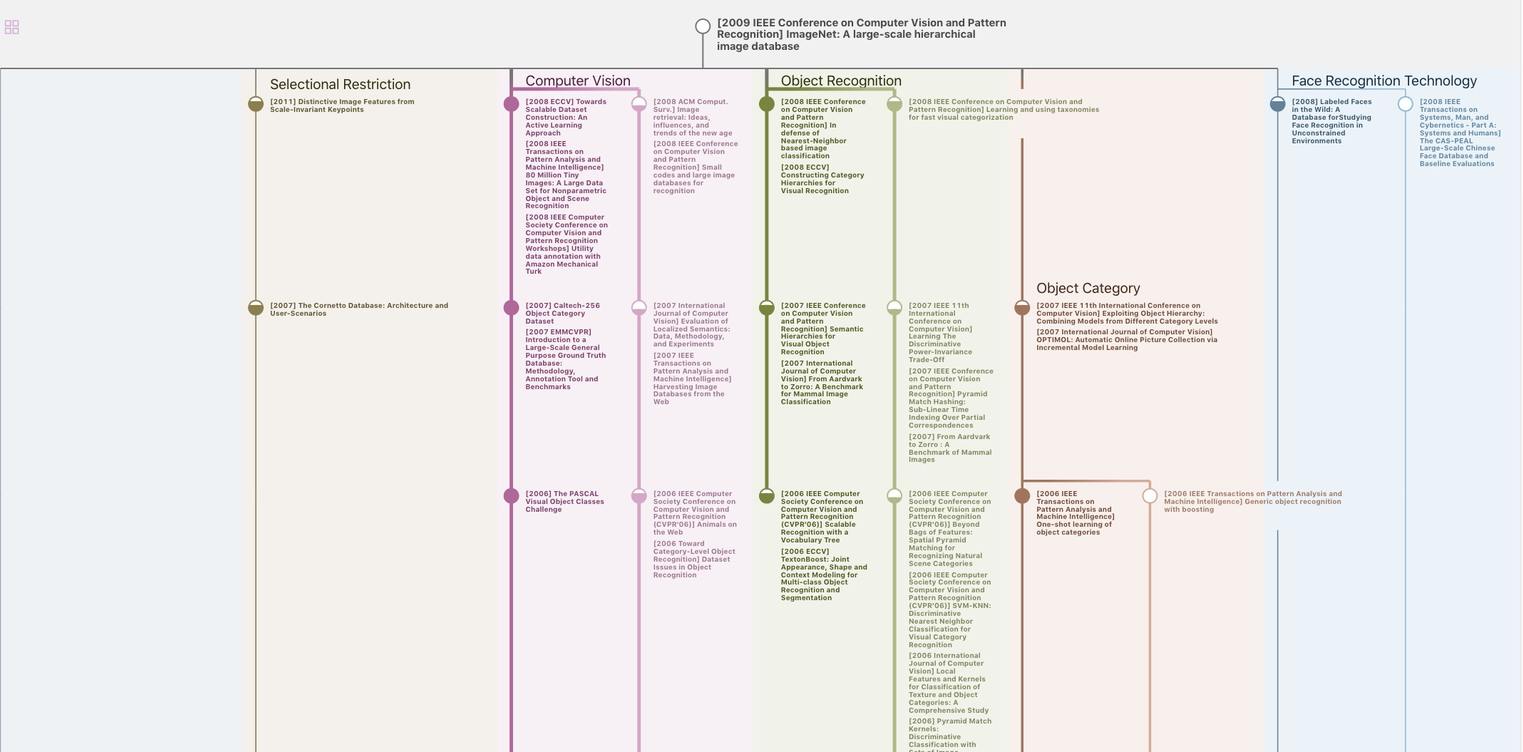
生成溯源树,研究论文发展脉络
Chat Paper
正在生成论文摘要