Artificial Neural Network Based Arousal Detection from Sleep Electroencephalogram Data
Computer, Communications, and Control Technology(2014)
摘要
Electroencephalographic arousals are characterized by a sudden shift in electroencephalogram (EEG) frequency during sleep. Occurrence of arousals causes improper sleep which is the main reason of day-time sleepiness. The arousal during the sleep is detected by analyzing a multimodal multichannel Polysomnographic (PSG) recordings. It is a time consuming task to analyze the recording manually and requires a lot of patience. Hence automation of the process is required. This task becomes difficult because of the presence of a lot of events in time in relation to various bio-medical signals available through Polysomnographic (PSG) recordings. In this paper we present a method to detect the arousals in sleep automatically. Firstly two sets of electroencephalogram (EEG) channels C-4/M-1 and C-3/M-2 and an electromyogram (EMG) channel are chosen for preprocessing. Then the electroencephalogram (EEG) signals are passed through a bandpass filter of 8-30 Hz in order to extract the signal containing only the alpha and beta frequency components. The events are detected when the Power spectral density of this filtered signal crosses a threshold of zero. When an event is detected the relevant features from Alpha, Beta, Theta, Delta, and sigma waves are extracted. Similarly when electromyogram (EMG) signal crosses a threshold of zero events are detected and relevant features are extracted for the corresponding events. Now all these features are grouped together along with the corresponding labels and used as inputs to the Artificial Neural Network (ANN) Classifier to detect the presence of arousals. The novelty of this work relies on using both Hjorth and Power Spectral density difference spectrum features leading to improved accuracy than either of them alone. Considering 10 overnight sleep recordings We recorded an average sensitivity of 0.93262, average specificity of 0.91387, average precision of 0.91693 and average Area under Region of Convergence curve (AUC) of 0.92328 (showing a good measure of overall performance).
更多查看译文
关键词
band-pass filters,electroencephalography,electromyography,feature extraction,medical signal detection,neural nets,sensitivity analysis,signal classification,sleep,spectral analysis,ANN,AUC,Artificial Neural Network Classifier,EEG,EMG,Hjorth,PSG,Power Spectral density difference spectrum,alpha frequency components,alpha waves,artificial neural network based arousal detection,average Area under Region of Convergence curve,bandpass filter,beta frequency components,beta waves,biomedical signals,day-time sleepiness,delta waves,electroencephalogram channels,electroencephalogram frequency,electroencephalogram signals,electroencephalographic arousals,electromyogram channel,electromyogram signal,frequency 8 Hz to 30 Hz,multimodal multichannel Polysomnographic recordings,overnight sleep recordings,power spectral density,sigma waves,signal extraction,sleep electroencephalogram data,theta waves,zero event threshold
AI 理解论文
溯源树
样例
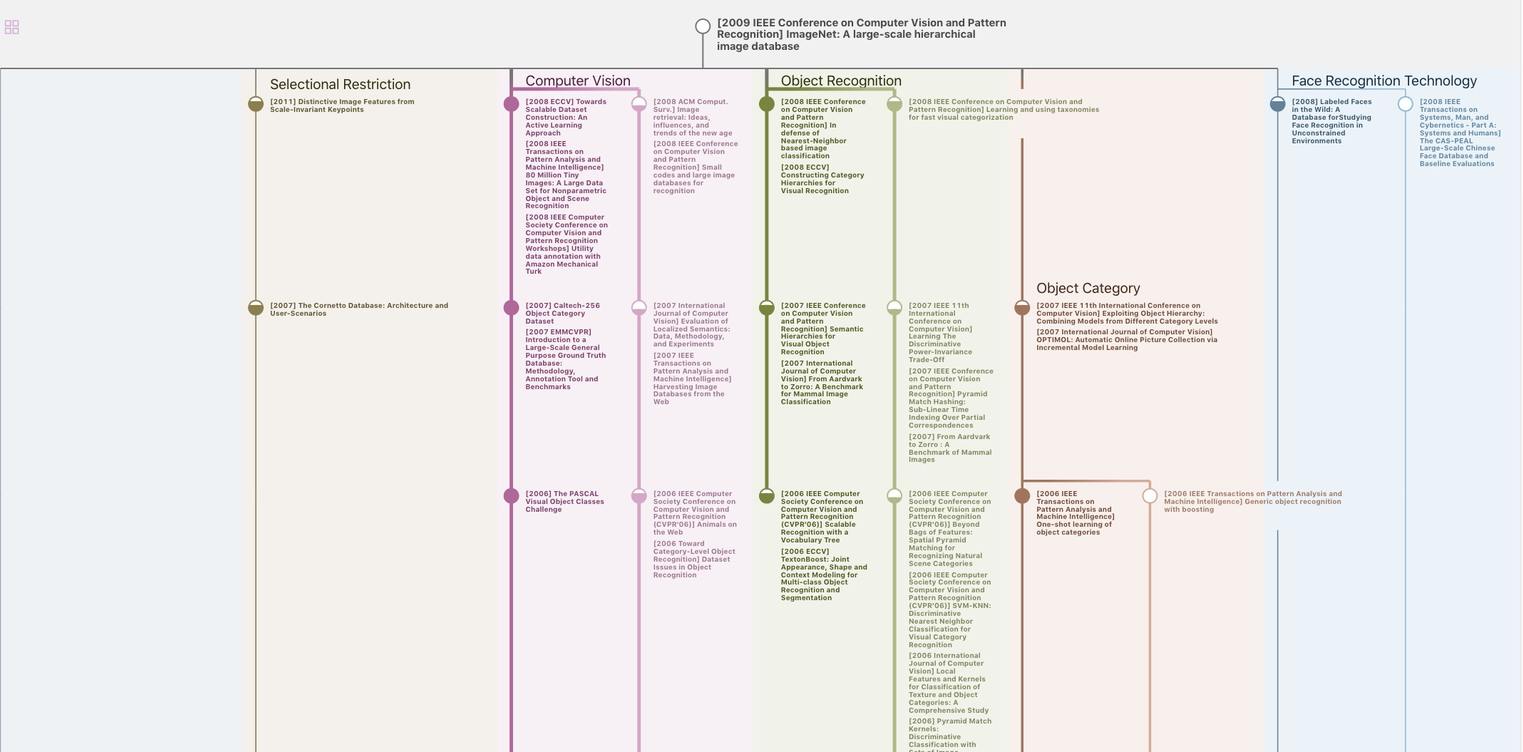
生成溯源树,研究论文发展脉络
Chat Paper
正在生成论文摘要