Memory Augmented Hierarchical Attention Network for Next Point-of-Interest Recommendation
IEEE Transactions on Computational Social Systems(2021)
摘要
Next point-of-interest (POI) recommendation has been an important task for location-based intelligent services. However, the application of such promising technique is still limited due to the following three challenges: 1) the difficulty of capturing complicated spatiotemporal patterns of user movements; 2) the hardness of modeling fine-grained long-term preferences of users; and 3) the effective learning of interaction between long- and short-term preferences. Motivated by this, we propose a memory augmented hierarchical attention network (MAHAN), which considers both short-term check-in sequences and long-term memories. To capture the complicated interest tendencies of users within a short-term period, we design a spatiotemporal self-attention network (ST-SAN). For long-term preferences modeling, we employ a memory network to maintain fine-grained preferences of users and dynamically operate them based on users' constantly updated check-ins. Moreover, we first employ a coattention network/mechanism to integrate the proposed ST-SAN and memory network, which can fully learn the dynamic interaction between long- and short-term preferences. Our extensive experiments on two publicly available data sets demonstrate the effectiveness of MAHAN.
更多查看译文
关键词
Coattention,dynamic preference,memory network,point-of-interest (POI) recommendation,self-attention
AI 理解论文
溯源树
样例
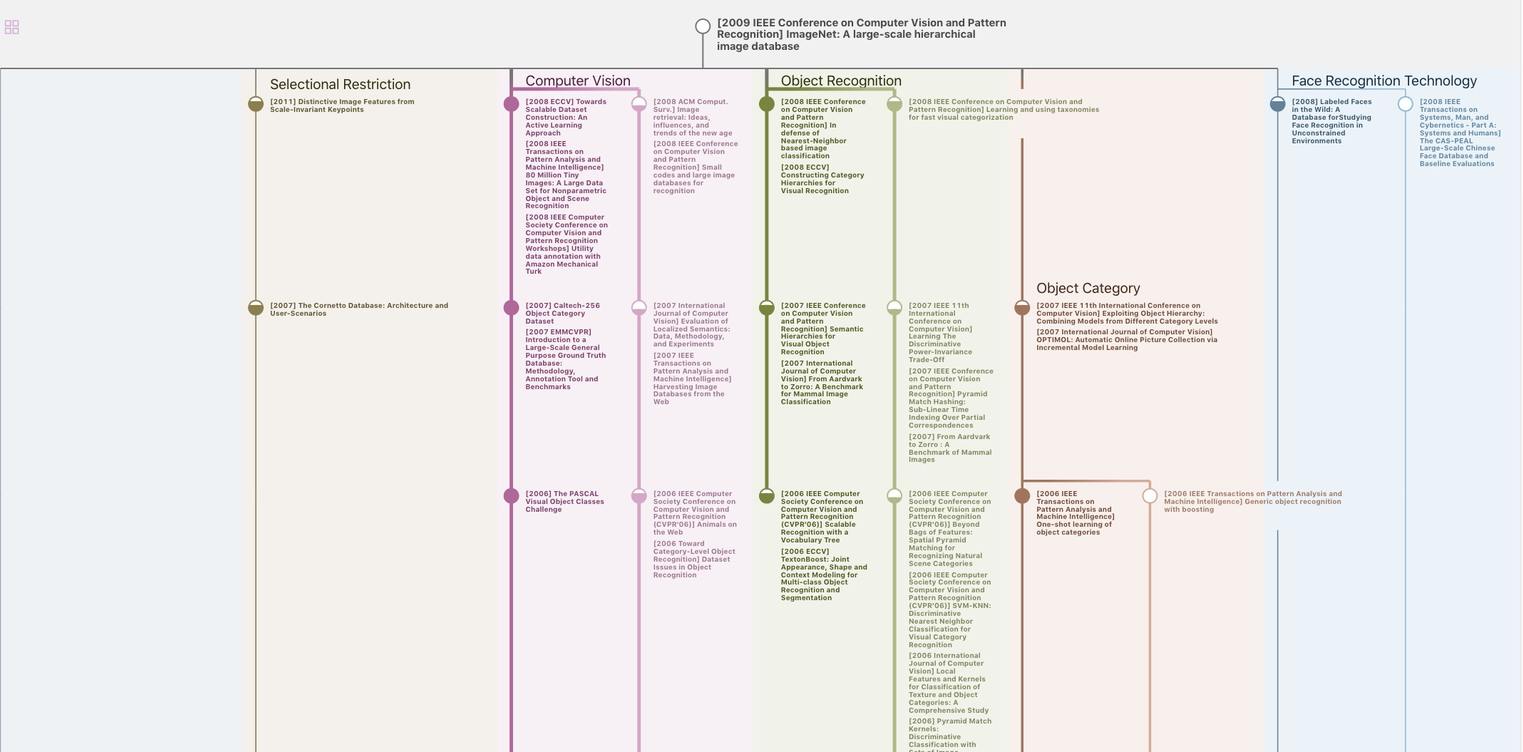
生成溯源树,研究论文发展脉络
Chat Paper
正在生成论文摘要