EEG Signals Classification related to Visual Objects using Long Short-Term Memory Network and Nonlinear Interval Type-2 Fuzzy Regression
Research Square (Research Square)(2023)
摘要
Abstract By comprehending how brain activity is encoded and decoded, we can better comprehend how the brain functions. This study presents a method for classifying EEG signals from visual objects that combines an LSTM network with nonlinear interval type-2 fuzzy regression (NIT2FR). Here, ResNet is used to extract features from the images, the LSTM network is used to extract features from the EEG signal, and NIT2FR is used to map the features from the images to the features from the EEG signal. In this paper, type-2 fuzzy logic is utilized to handle this type of uncertainty due to the nonlinearity and noise of the EEG signals, the short sample size of the data, and the varied mental backgrounds of the experiment participants. The Stanford database was used to implement the research technique, and its effectiveness was assessed using the classification accuracy, precision, recall, and F1 score. The LSTM network successfully categorized images using raw EEG data with an accuracy of 55.83%, according to the findings. When compared to classification accuracy obtained with linear type-2, linear and nonlinear type-1 fuzzy, neural network, and polynomial regression, NIT2FR and SVM classifier performed better (68.05%). NIT2FR can therefore perform better in settings with high levels of uncertainty. Additionally, the accuracy outcomes using NIT2FR are 6.03% better than the top outcome of the most recent study that made use of the same dataset. The same process was followed to get the same result for the other performance raters.
更多查看译文
关键词
fuzzy,nonlinear interval,classification,visual objects,regression,short-term
AI 理解论文
溯源树
样例
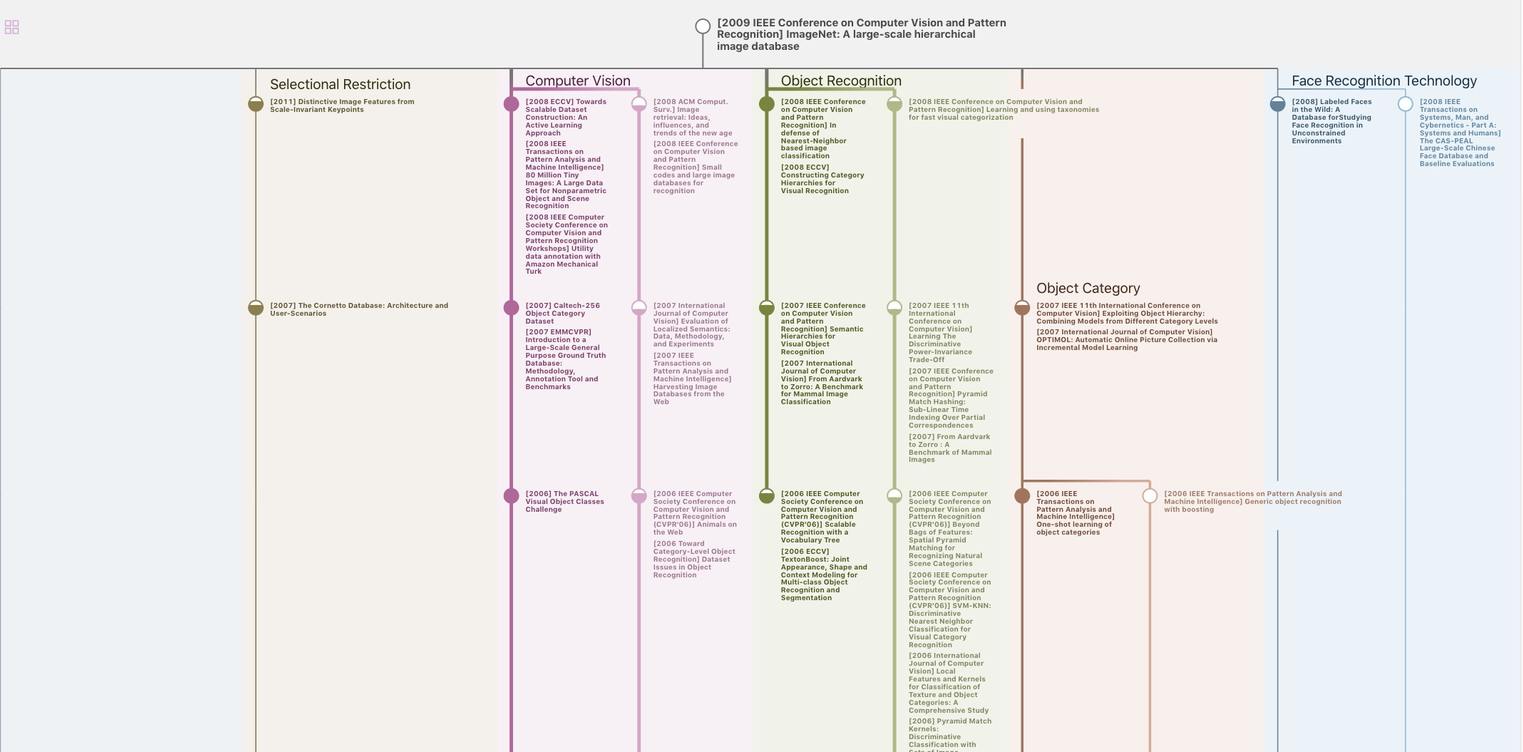
生成溯源树,研究论文发展脉络
Chat Paper
正在生成论文摘要