Enhancing Needle Tracking in Ultrasound Guided Interventions Using Unsupervised Reinforcement Learning Based Accelerated Adaptive Minimum Variance Beamforming
2023 IEEE International Ultrasonics Symposium (IUS)(2023)
摘要
Imaging of specular structures such as needles during a guided intervention has been a challenge in ultrasound (US) imaging. Wider field-of-view imaging using plane waves has advantages over conventional focused transmit imaging for highly directive specular reflections. For receive beamforming, tissue adaptive algorithms are needed so that the apodization weights are not fixed based on the diffuse scattering assumption as in the standard delay and sum beamforming in US imaging. Minimum variance (MV) based beamforming approaches have been the most popular adaptive beamforming but have not been employed widely in practice due to heavy computational cost. In the recent past, the acceleration of MV approaches employing neural networks has been gaining popularity. However, such approaches have two primary issues, 1) the parameters (e.g. sub-aperture length) are empirically fixed and 2) the neural network is often implemented through supervised learning. In this work, these issues are addressed with 1) a reinforcement learning framework for parameter selection (i.e., sub-aperture length) and 2) an image quality based reward to enable fully unsupervised learning. The initial results show promise for further development by incorporating multiple parameters and assessing image quality using more realistic metrics.
更多查看译文
关键词
minimum variance,needle tracking,reinforcement learning,specular reflection,ultrasound
AI 理解论文
溯源树
样例
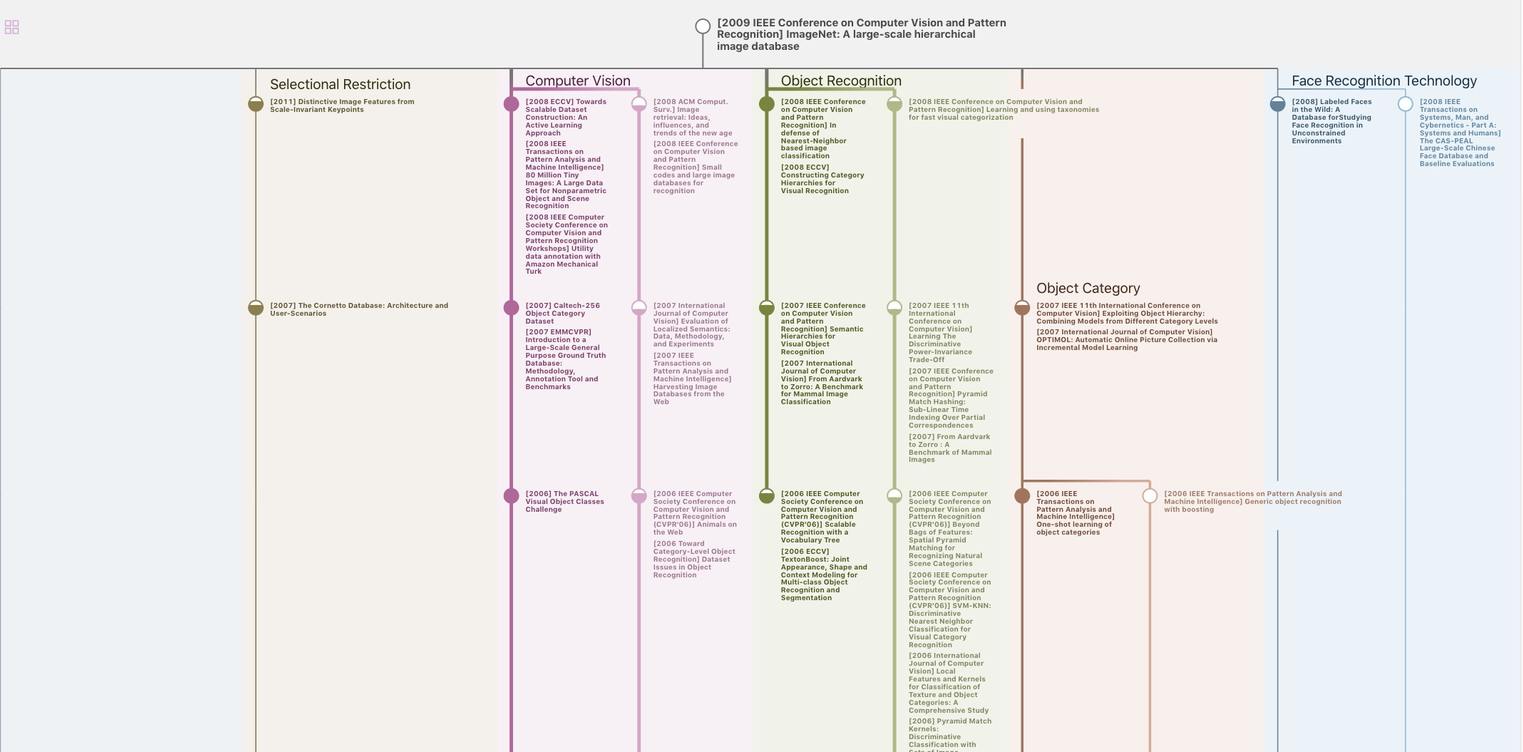
生成溯源树,研究论文发展脉络
Chat Paper
正在生成论文摘要