Cross-validatory extreme value threshold selection and uncertainty with application to ocean storm severity
JOURNAL OF THE ROYAL STATISTICAL SOCIETY SERIES C-APPLIED STATISTICS(2017)
摘要
Design conditions for marine structures are typically informed by threshold-based extreme value analyses of oceanographic variables, in which excesses of a high threshold are modelled by a generalized Pareto distribution. Too low a threshold leads to bias from model misspecification, and raising the threshold increases the variance of estimators: a bias-variance trade-off. Many existing threshold selection methods do not address this trade-off directly but rather aim to select the lowest threshold above which the generalized Pareto model is judged to hold approximately. In the paper Bayesian cross-validation is used to address the trade-off by comparing thresholds based on predictive ability at extreme levels. Extremal inferences can be sensitive to the choice of a single threshold. We use Bayesian model averaging to combine inferences from many thresholds, thereby reducing sensitivity to the choice of a single threshold. The methodology is applied to significant wave height data sets from the northern North Sea and the Gulf of Mexico.
更多查看译文
关键词
Cross-validation,Extreme value theory,Generalized Pareto distribution,Predictive inference,Threshold
AI 理解论文
溯源树
样例
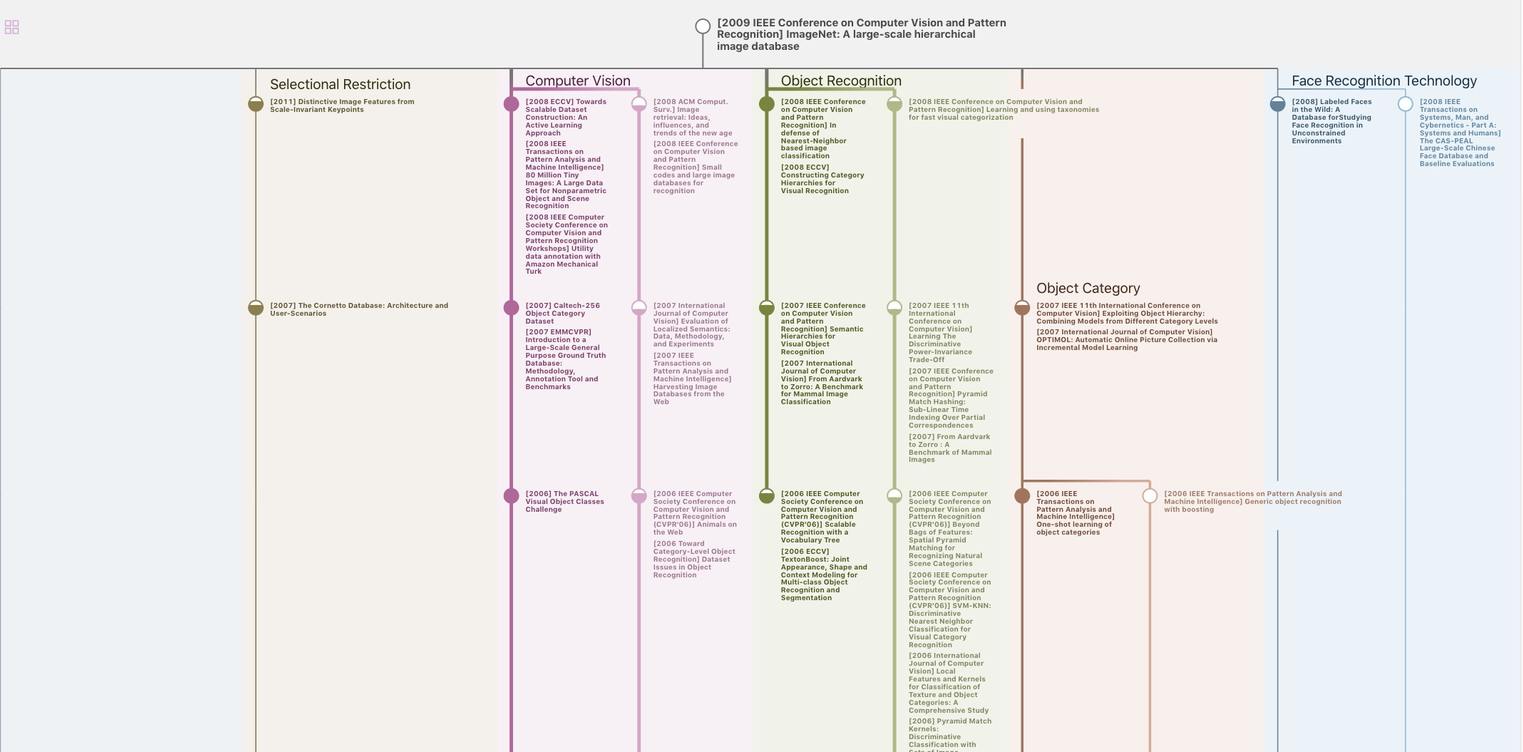
生成溯源树,研究论文发展脉络
Chat Paper
正在生成论文摘要