Optimal Privacy Guarantees for a Relaxed Threat Model: Addressing Sub-Optimal Adversaries in Differentially Private Machine Learning.
NeurIPS 2023(2023)
摘要
Differentially private mechanisms restrict the membership inference capabilities of powerful (optimal) adversaries against machine learning models. Such adversaries are rarely encountered in practice. In this work, we examine a more realistic threat model relaxation, where (sub-optimal) adversaries lack access to the exact model training database, but may possess related or partial data. We then formally characterise and experimentally validate adversarial membership inference capabilities in this setting in terms of hypothesis testing errors. Our work helps users to interpret the privacy properties of sensitive data processing systems under realistic threat model relaxations and choose appropriate noise levels for their use-case.
更多查看译文
关键词
Differential Privacy,Membership Inference Attack,Hypothesis Testing,Data Reconstruction Attack,Security
AI 理解论文
溯源树
样例
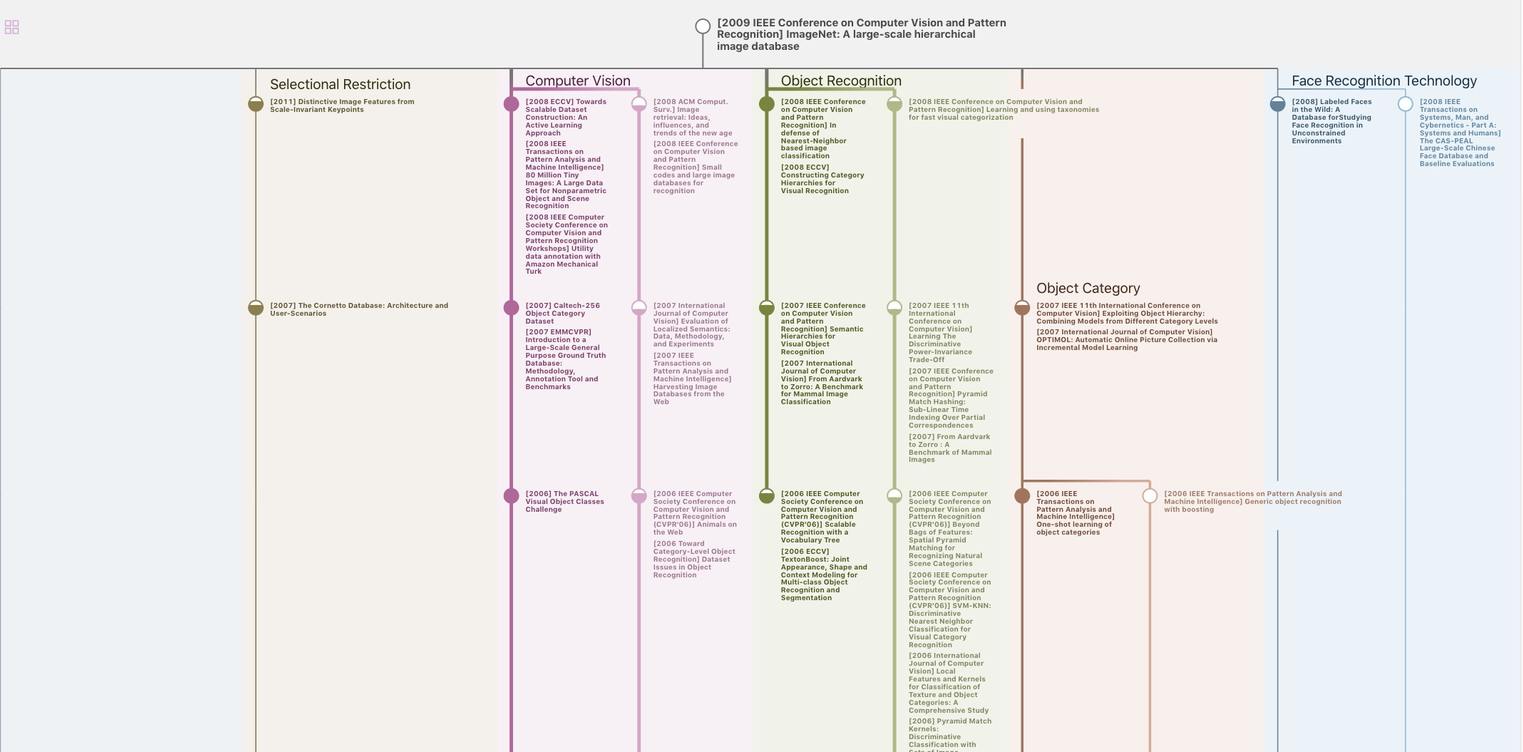
生成溯源树,研究论文发展脉络
Chat Paper
正在生成论文摘要