Unsupervised Hyperspectral Unmixing Based on Constrained Nonnegative Matrix Factorization and Particle Swarm Optimization
GCIS '10 Proceedings of the 2010 Second WRI Global Congress on Intelligent Systems - Volume 03(2010)
摘要
The constrained nonnegative matrix factorization algorithm (CNMF) has previously been shown to be a useful method to solve the unmixing problem in hyper spectral remote sensing images, but it also has some key weaknesses which affect its applied range. It's sensitive to the initial values, and easily falls to the local minimum. To solve the problems, a new intelligent optimization method - PSO(Particle Swarm Optimization) algorithm is combined with CNMF. The end members and abundance fractions obtained by CNMF are adopted as the initial values of PSO, the optimal solution of PSO is in reverse as the new initial value in the next running of CNMF, and this procedure is repeated until the global optimal solution is achieved. The experimental results based on synthetic data and real images demonstrate that the proposed method outperforms the standard CNMF algorithm and CNMF with output of VCA as its initial values.
更多查看译文
关键词
initial value,standard CNMF algorithm,new initial value,new intelligent optimization method,nonnegative matrix factorization algorithm,proposed method,useful method,global optimal solution,optimal solution,Particle Swarm Optimization,Constrained Nonnegative Matrix Factorization,Unsupervised Hyperspectral
AI 理解论文
溯源树
样例
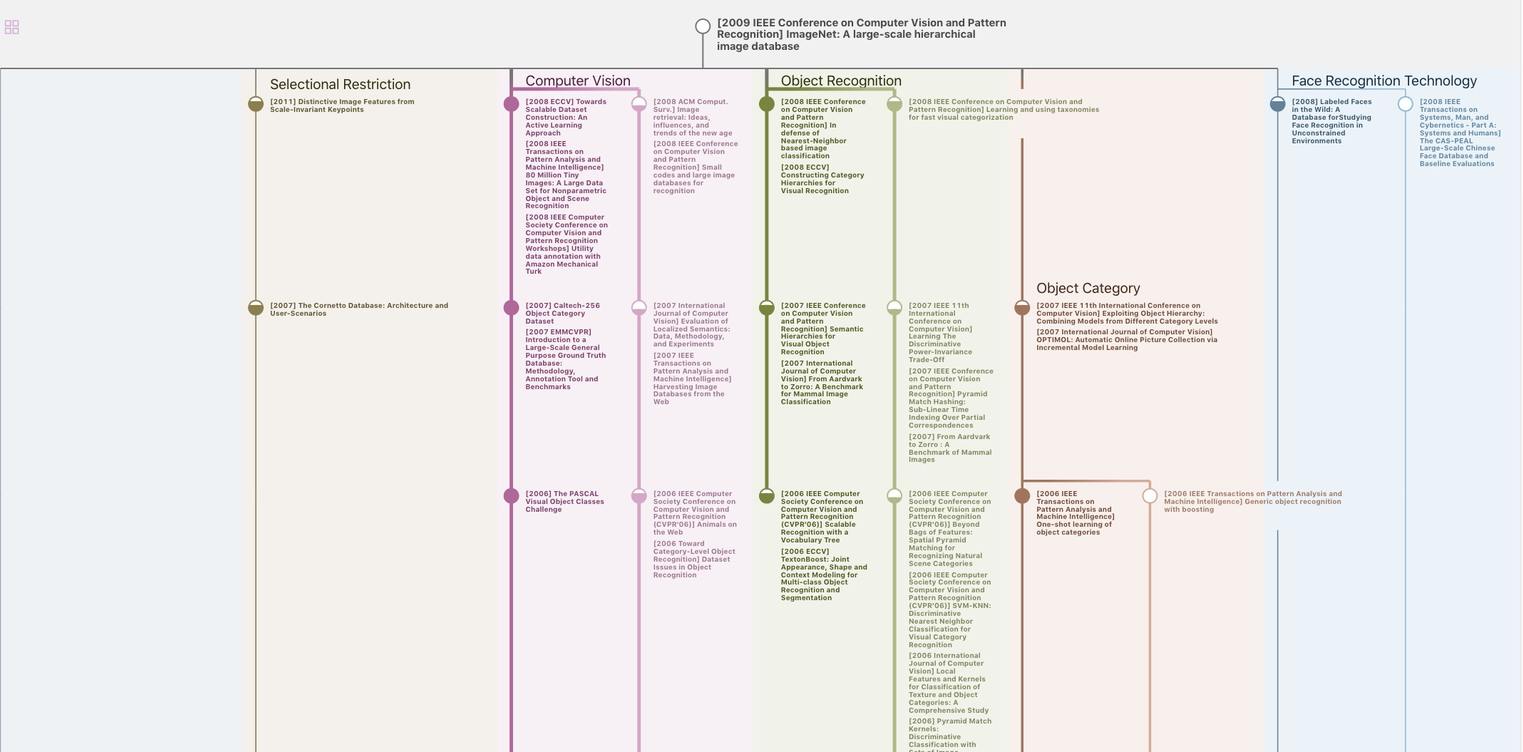
生成溯源树,研究论文发展脉络
Chat Paper
正在生成论文摘要