Multiple-Instance Learning Improves CAD Detection of Masses in Digital Mammography
DIGITAL MAMMOGRAPHY, PROCEEDINGS(2008)
摘要
We propose a novel multiple-instance learning(MIL) algorithm for designing classifiers for use in computer aided detection(CAD). The proposed algorithm has 3 advantages over classical methods. First, unlike traditional learning algorithms that minimize the candidate level misclassification error, the proposed algorithm directly optimizes the patient-wise sensitivity. Second, this algorithm automatically selects a small subset of statistically useful features. Third, this algorithm is very fast, utilizes all of the available training data (without the need for cross-validation etc.), and requires no human hand tuning or intervention. Experimentally the algorithm is more accurate than state of the art support vector machine (SVM) classifier, and substantially reduces the number of features that have to be computed.
更多查看译文
关键词
classical method,traditional learning algorithm,patient-wise sensitivity,novel multiple-instance learning,available training data,small subset,art support vector machine,digital mammography,candidate level misclassification error,proposed algorithm,multiple-instance learning improves cad,human hand tuning,support vector machine,cross validation
AI 理解论文
溯源树
样例
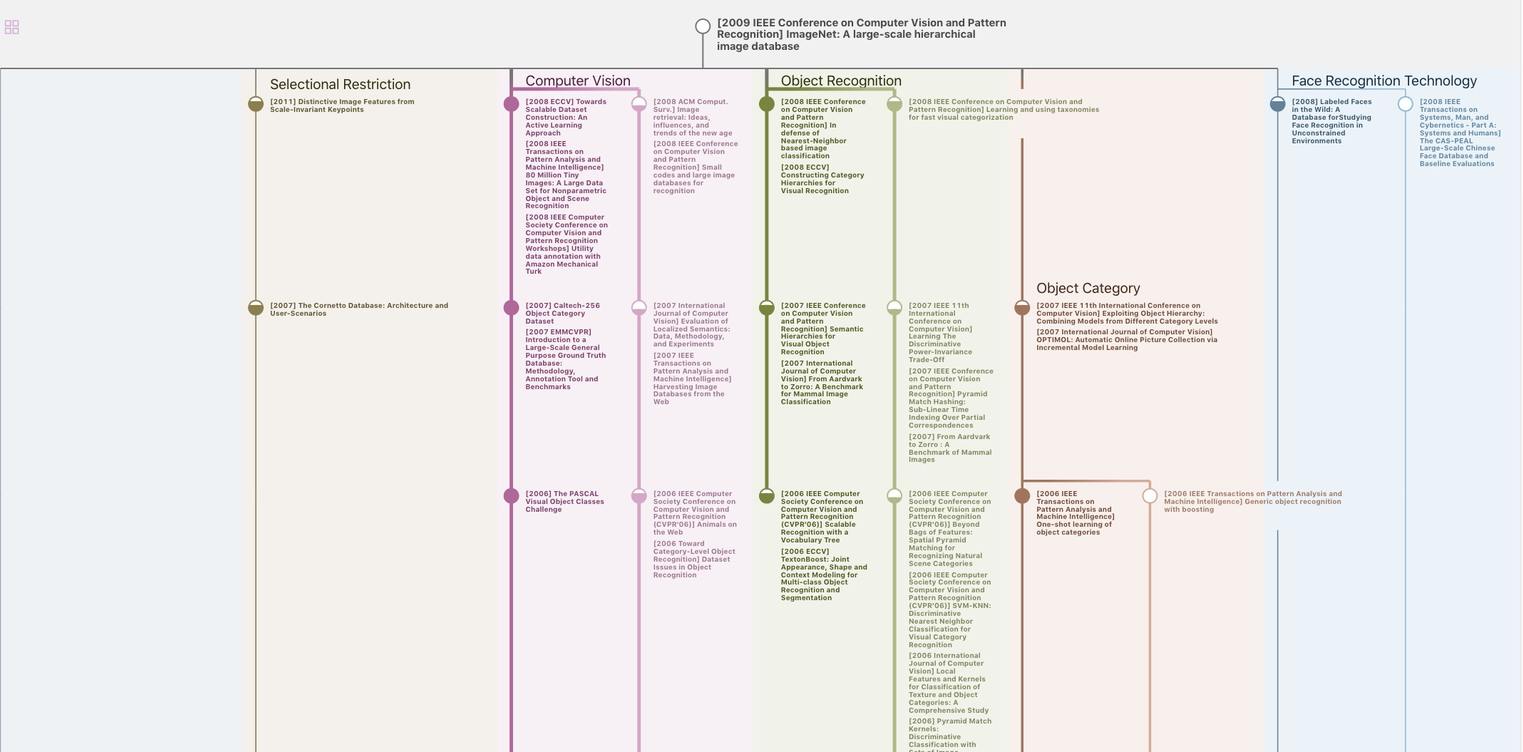
生成溯源树,研究论文发展脉络
Chat Paper
正在生成论文摘要