Improving BMRC Based on Joint Classifier for Aspect Sentiment Triplet Extraction
2023 4th International Conference on Computer, Big Data and Artificial Intelligence (ICCBD+AI)(2023)
摘要
Aspect sentiment triplet extraction(ASTE) is an extremely difficult subtask in aspect-based sentiment analysis. The ASTE task aims to extract triplets of aspect, opinion, and sentiment polarity from review sentences. Bidirectional machine reading comprehension (BMRC) is the current method to settle triplet extraction based on the machine reading comprehension (MRC) paradigm. This method can effectively settle the ASTE task. However, due to the use of shared classifiers, it will cause query conflicts and there will be a problem of reduced probability when predicting answers. Therefore, this paper proposes an improving BMRC based on a joint classifier for aspect sentiment triplet extraction, while adding a balanced span prediction module (BSPM). The joint classifier can effectively settle the query conflict issue and exploit the correlation between subtasks. The balanced span prediction module can make the predicted probabilities of opinion, aspect, and aspect-opinion pairs more consistent with the expectations of the model. We have conducted enough experiments on four benchmark datasets, where our models achieve more significant improvements over the baseline models.
更多查看译文
关键词
aspect-based sentiment analysis,BMRC,ASTE,classifier,span prediction
AI 理解论文
溯源树
样例
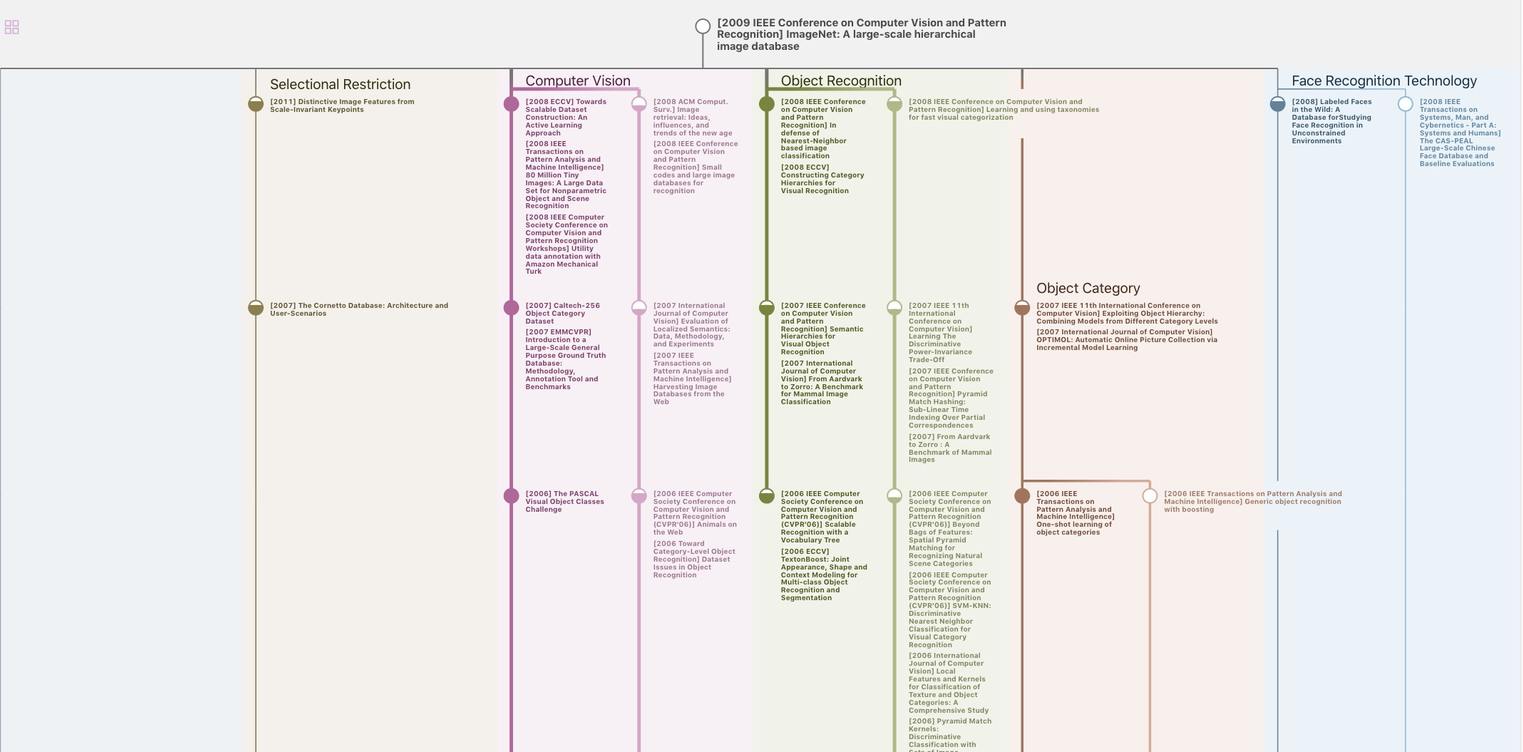
生成溯源树,研究论文发展脉络
Chat Paper
正在生成论文摘要