Learning consensus representations in multi-latent spaces for multi-view clustering
Neurocomputing(2024)
摘要
Multi-view clustering integrates features from different views to perform clustering. This problem has attracted increasing attention in recent years because multi-view data has become more common. The mainstream methods focus on learning a common representation or decoupling the view-specific and the shared representations in subspaces and then performing clustering on the fused results. However, it is still an open question how to best enforce that the learned representations possess good clustering properties and thus improve the clustering performance. In this paper, we propose a novel unsupervised model called Deep Multi-view Consensus Clustering (DMCC) to learn consensus view-specific representations in multiple latent spaces, where specificity and consistency are jointly retained for representation learning. For each view, DMCC learns view-specific representations in individual latent spaces with the help of a reconstruction target and a soft K-means objective. Furthermore, by aligning the cluster indicator matrices of each view, DMCC encourages consensus across views and enables one view to get help from other views to guide its representation learning. Thus, the learned representations are cluster-friendly within each view, and consistent across views. The proposed method achieves state-of-the-art performance in four metrics on extensive datasets. Among all datasets, our proposed method DMCC achieves an average of 2.6% and 2.5% better performance than state-of-the-art methods in RI and NMI, respectively. We also visualize the learned representations to show that our approach does learn cluster-friendly representations and to demonstrate the effectiveness of encouraging mutual consensus across views.
更多查看译文
关键词
Multi-view learning,Deep clustering,Latent space,Consensus representations
AI 理解论文
溯源树
样例
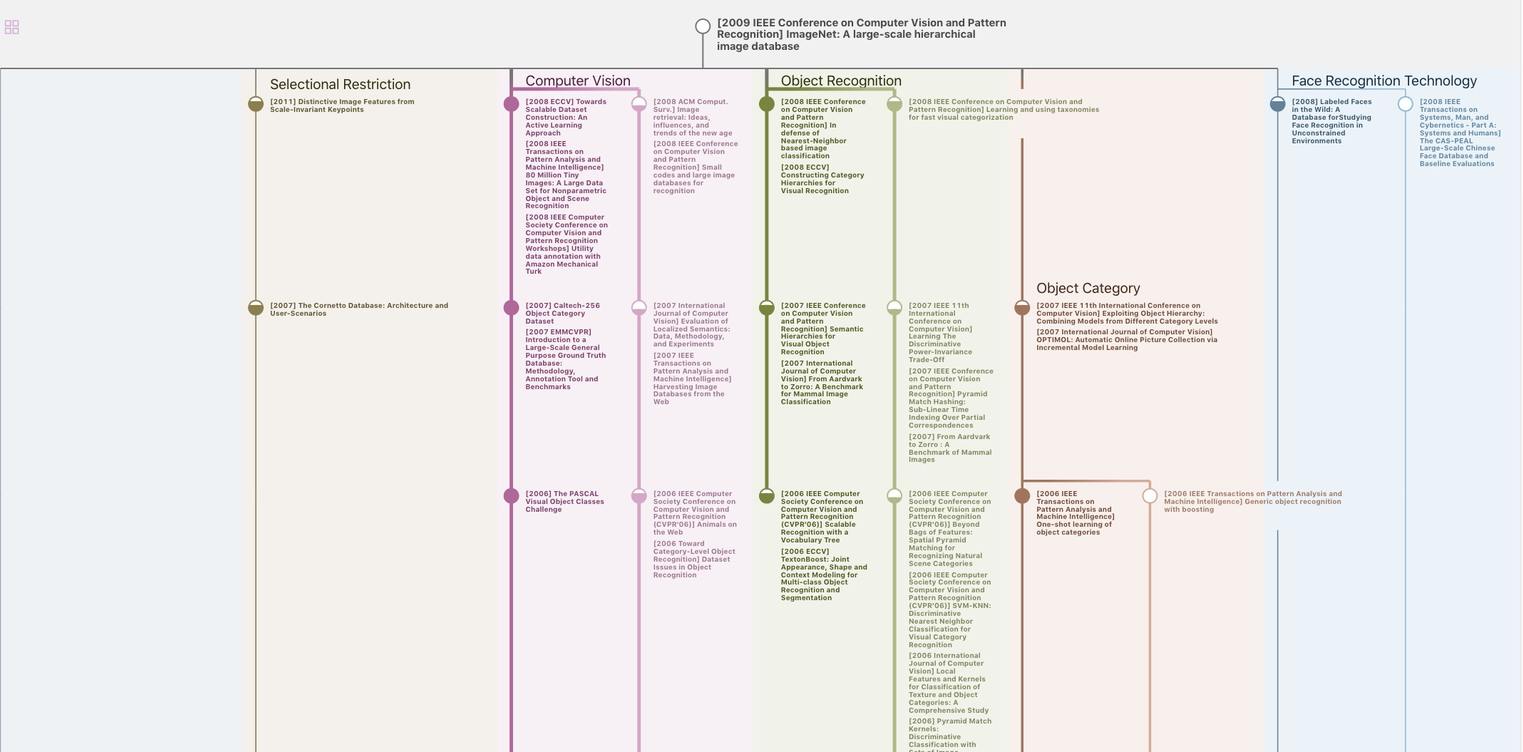
生成溯源树,研究论文发展脉络
Chat Paper
正在生成论文摘要