Towards a Framework for Openness in Foundation Models: Proceedings from the Columbia Convening on Openness in Artificial Intelligence
CoRR(2024)
摘要
Over the past year, there has been a robust debate about the benefits and
risks of open sourcing foundation models. However, this discussion has often
taken place at a high level of generality or with a narrow focus on specific
technical attributes. In part, this is because defining open source for
foundation models has proven tricky, given its significant differences from
traditional software development. In order to inform more practical and nuanced
decisions about opening AI systems, including foundation models, this paper
presents a framework for grappling with openness across the AI stack. It
summarizes previous work on this topic, analyzes the various potential reasons
to pursue openness, and outlines how openness varies in different parts of the
AI stack, both at the model and at the system level. In doing so, its authors
hope to provide a common descriptive framework to deepen a nuanced and rigorous
understanding of openness in AI and enable further work around definitions of
openness and safety in AI.
更多查看译文
AI 理解论文
溯源树
样例
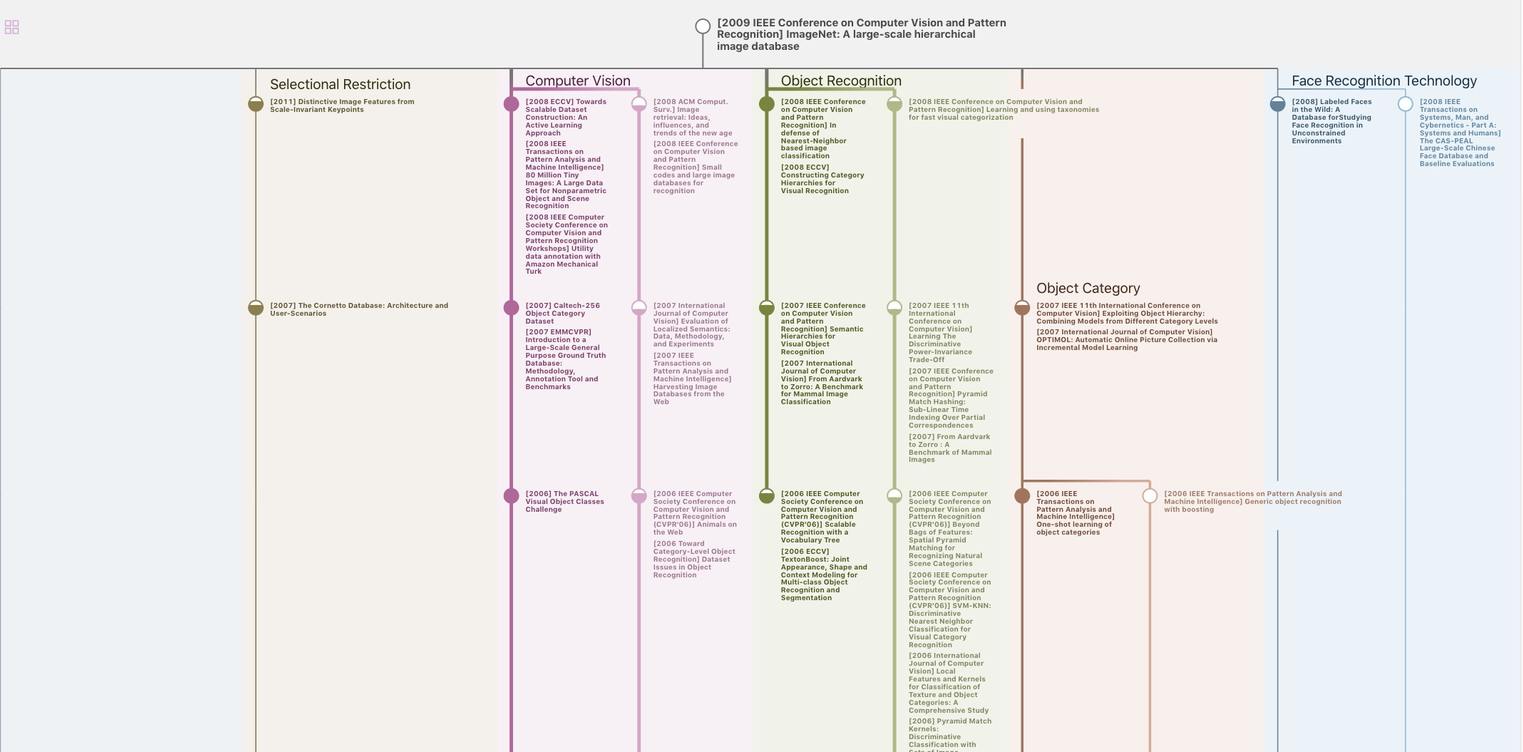
生成溯源树,研究论文发展脉络
Chat Paper
正在生成论文摘要