Structural Entities Extraction and Patient Indications Incorporation for Chest X-ray Report Generation
arxiv(2024)
Abstract
The automated generation of imaging reports proves invaluable in alleviating
the workload of radiologists. A clinically applicable reports generation
algorithm should demonstrate its effectiveness in producing reports that
accurately describe radiology findings and attend to patient-specific
indications. In this paper, we introduce a novel method, Structural
Entities extraction and patient indications Incorporation
(SEI) for chest X-ray report generation. Specifically, we employ a structural
entities extraction (SEE) approach to eliminate presentation-style vocabulary
in reports and improve the quality of factual entity sequences. This reduces
the noise in the following cross-modal alignment module by aligning X-ray
images with factual entity sequences in reports, thereby enhancing the
precision of cross-modal alignment and further aiding the model in
gradient-free retrieval of similar historical cases. Subsequently, we propose a
cross-modal fusion network to integrate information from X-ray images, similar
historical cases, and patient-specific indications. This process allows the
text decoder to attend to discriminative features of X-ray images, assimilate
historical diagnostic information from similar cases, and understand the
examination intention of patients. This, in turn, assists in triggering the
text decoder to produce high-quality reports. Experiments conducted on
MIMIC-CXR validate the superiority of SEI over state-of-the-art approaches on
both natural language generation and clinical efficacy metrics.
MoreTranslated text
AI Read Science
Must-Reading Tree
Example
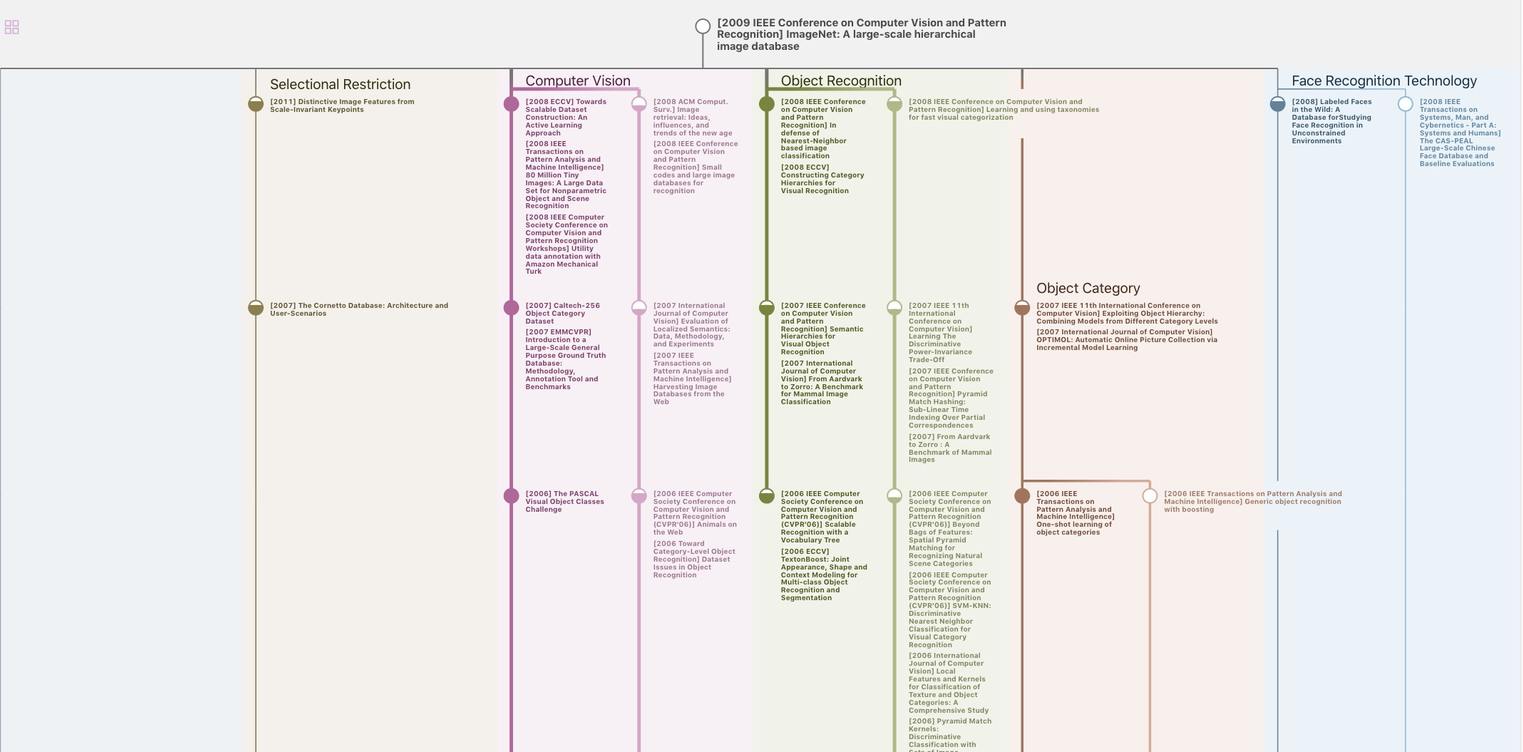
Generate MRT to find the research sequence of this paper
Chat Paper
Summary is being generated by the instructions you defined