One-shot Training for Video Object Segmentation
CoRR(2024)
摘要
Video Object Segmentation (VOS) aims to track objects across frames in a
video and segment them based on the initial annotated frame of the target
objects. Previous VOS works typically rely on fully annotated videos for
training. However, acquiring fully annotated training videos for VOS is
labor-intensive and time-consuming. Meanwhile, self-supervised VOS methods have
attempted to build VOS systems through correspondence learning and label
propagation. Still, the absence of mask priors harms their robustness to
complex scenarios, and the label propagation paradigm makes them impractical in
terms of efficiency. To address these issues, we propose, for the first time, a
general one-shot training framework for VOS, requiring only a single labeled
frame per training video and applicable to a majority of state-of-the-art VOS
networks. Specifically, our algorithm consists of: i) Inferring object masks
time-forward based on the initial labeled frame. ii) Reconstructing the initial
object mask time-backward using the masks from step i). Through this
bi-directional training, a satisfactory VOS network can be obtained. Notably,
our approach is extremely simple and can be employed end-to-end. Finally, our
approach uses a single labeled frame of YouTube-VOS and DAVIS datasets to
achieve comparable results to those trained on fully labeled datasets. The code
will be released.
更多查看译文
AI 理解论文
溯源树
样例
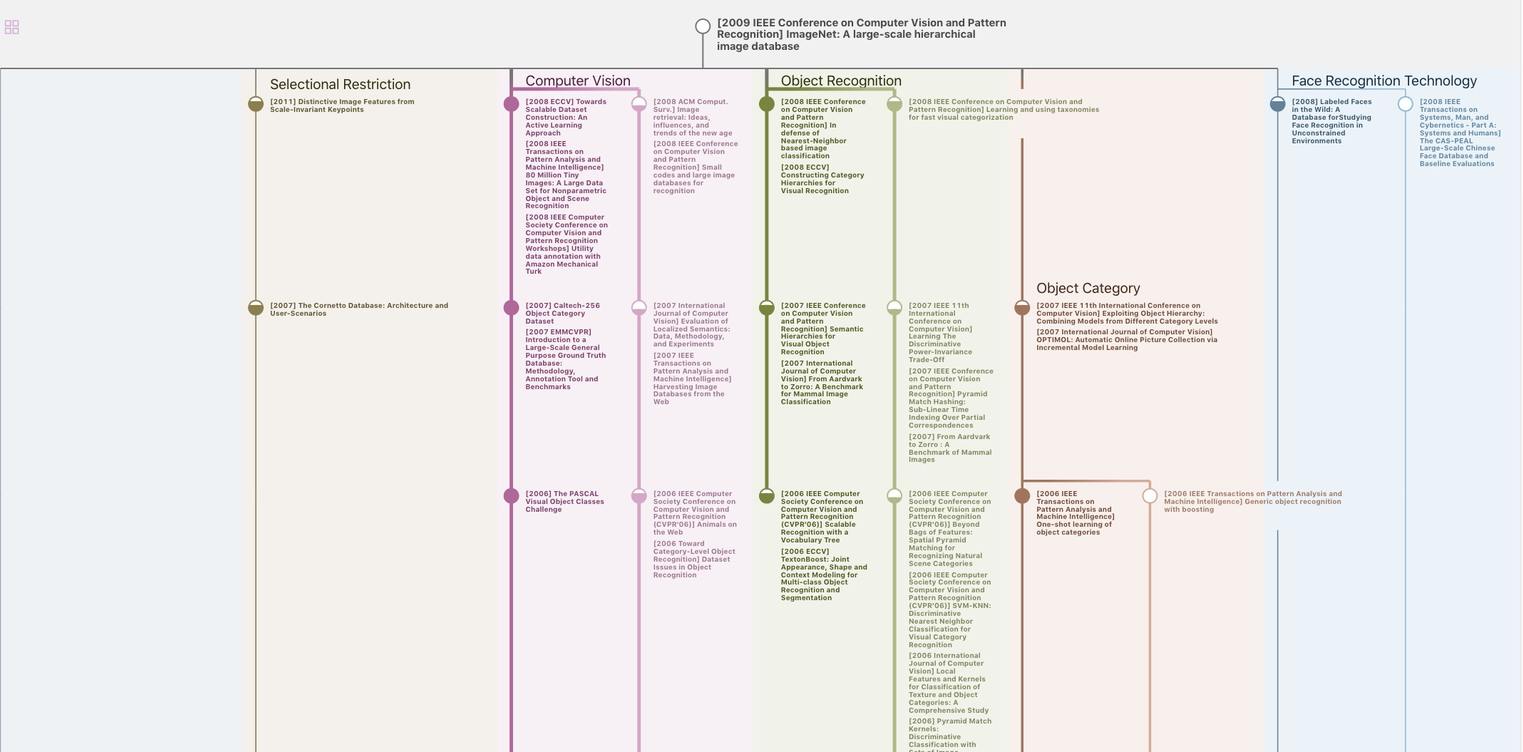
生成溯源树,研究论文发展脉络
Chat Paper
正在生成论文摘要