Efficient Multimodal Large Language Models: A Survey
arxiv(2024)
摘要
In the past year, Multimodal Large Language Models (MLLMs) have demonstrated
remarkable performance in tasks such as visual question answering, visual
understanding and reasoning. However, the extensive model size and high
training and inference costs have hindered the widespread application of MLLMs
in academia and industry. Thus, studying efficient and lightweight MLLMs has
enormous potential, especially in edge computing scenarios. In this survey, we
provide a comprehensive and systematic review of the current state of efficient
MLLMs. Specifically, we summarize the timeline of representative efficient
MLLMs, research state of efficient structures and strategies, and the
applications. Finally, we discuss the limitations of current efficient MLLM
research and promising future directions. Please refer to our GitHub repository
for more details:
https://github.com/lijiannuist/Efficient-Multimodal-LLMs-Survey.
更多查看译文
AI 理解论文
溯源树
样例
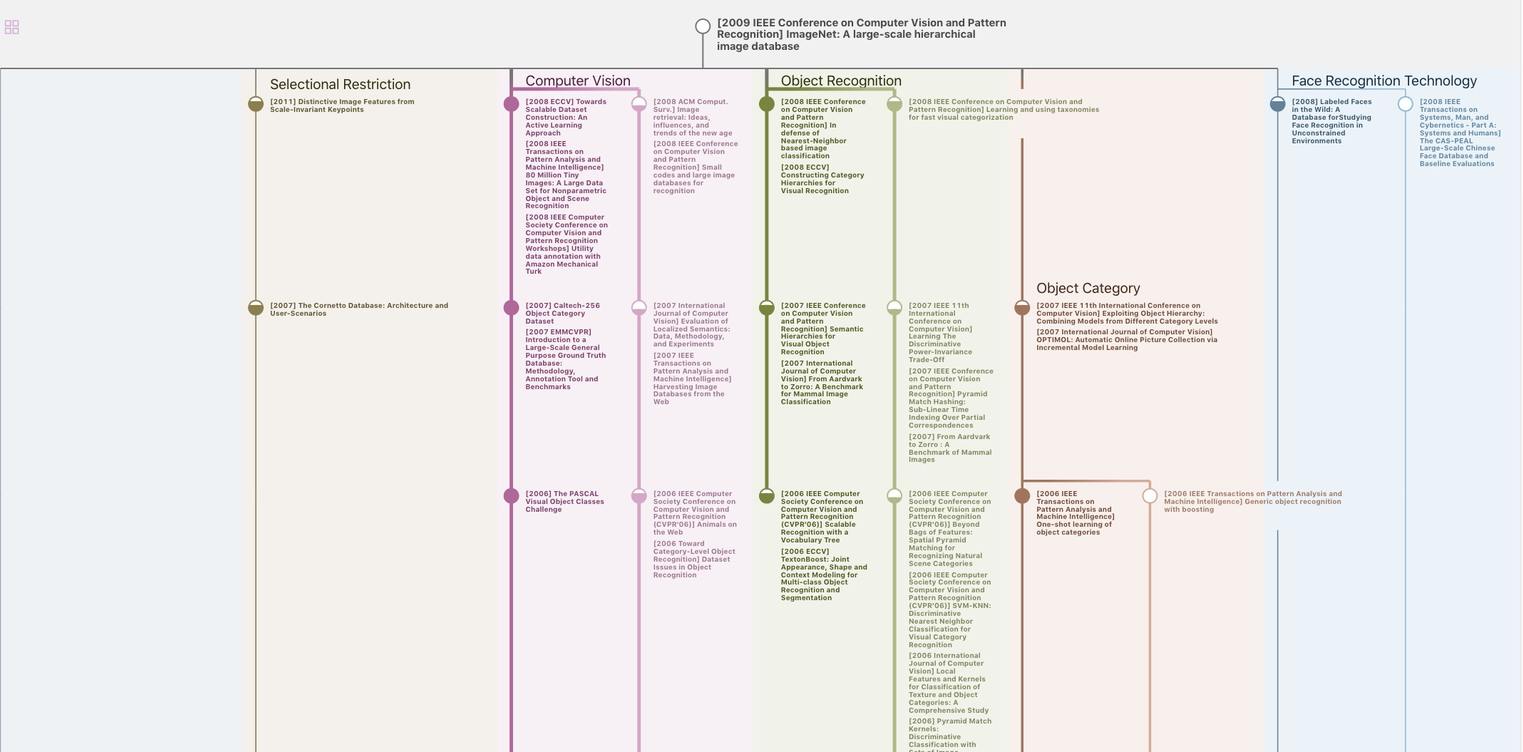
生成溯源树,研究论文发展脉络
Chat Paper
正在生成论文摘要