Reliable hybrid knowledge distillation for multi-source domain adaptive object detection
Knowledge-Based Systems(2024)
摘要
Although great efforts have been made in single source adaptation, most methods do not consider a more practical scenario where the source domain comprises multiple data distributions. In this paper, we propose a new multiple teacher-student framework (MTSF) for multi-source domain adaptive object detection. Our MTSF adopts multiple teacher-student sub-networks to distill domain specific knowledge from different source domains, yielding multiple precise pseudo labels. Considering that the samples from different domains are biased, we propose a new knowledge fusion module namely hybrid knowledge distillation (HKD) to fuse the knowledge by considering the contribution of each sample from different domains to the final decision on the target domain. Our HKD consists of a multi-source prototype construction (MSPC) module and a dense-to-sparse cross-domain pseudo label refinement (DCPR) module. According to the prototypes measured by MSPC, the DCPR distills the knowledge from dense to sparse in a hierarchical way, which makes the generated pseudo labels more reliable. When evaluated on benchmark adaptation scenarios (i.e. cross-camera and cross-time), our proposed method outperforms previous methods, establishing a new state-of-the-art.
更多查看译文
关键词
Domain adaptation,Object detection,Hybrid knowledge distillation (HKD),Multi-source prototype construction (MSPC),Dense-to-sparse cross-domain pseudo label refinement (DCPR)
AI 理解论文
溯源树
样例
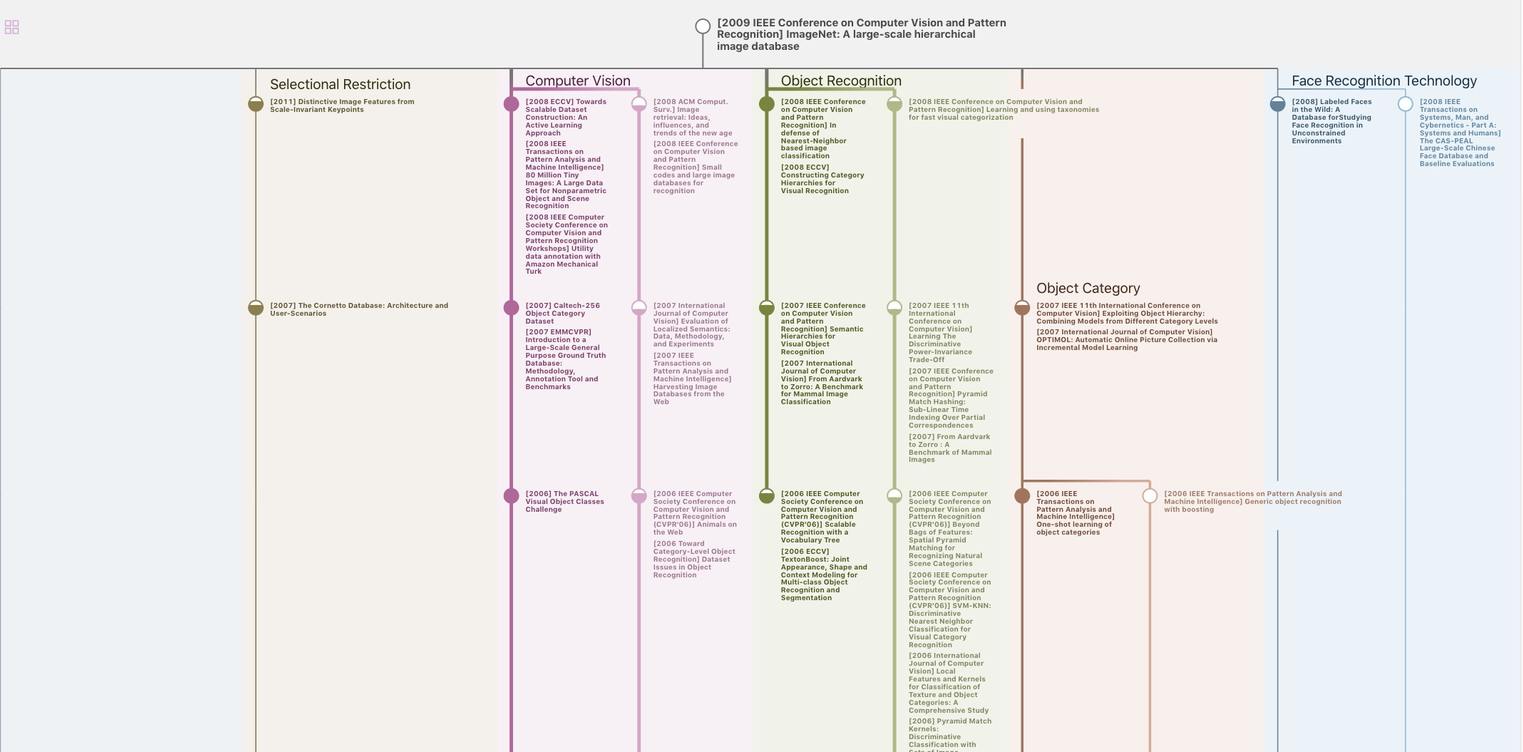
生成溯源树,研究论文发展脉络
Chat Paper
正在生成论文摘要