A 1-TFLOPS/W, 28-nm Deep Neural Network Accelerator Featuring Online Compression and Decompression and BF16 Digital In-Memory-Computing Hardware
2024 IEEE Custom Integrated Circuits Conference (CICC)(2024)
摘要
With the recent advances in deep neural networks (DNN), researchers have proposed various hardware accelerators. However, many neglected the energy consumption of off-chip memory access for weight and activation data, which can dominate the total energy consumption. To reduce off-chip data traffic, some works adopted aggressively quantized arithmetic, such as 1-4b fixed-point (FX1-FX4) or block-floating-point (BFP). However, they achieve only a limited computation precision, hurting DNN inference accuracy.
更多查看译文
关键词
Deep Neural Network,Online Compression,Root Mean Square Error,Energy Consumption,Energy Efficiency,Processing Unit,Weight Data,Lookup Table,Compressor,Binary Search,Hardware Accelerators,Compression Algorithm,Network Training Process,Bit-width,Computational Layers,Round Of Search,Alignment Blocks,Off-chip Memory,NOR Gate,Binary Search Algorithm
AI 理解论文
溯源树
样例
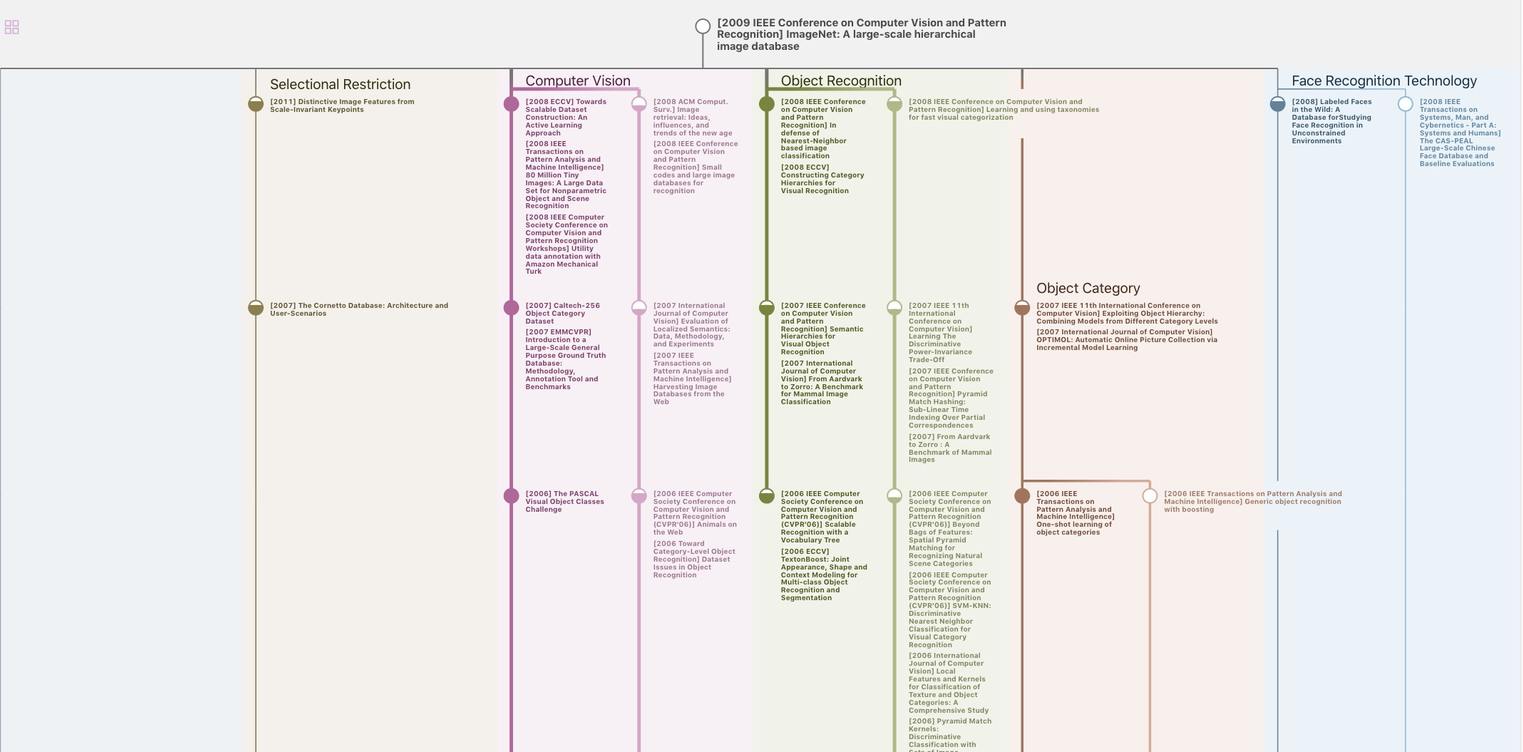
生成溯源树,研究论文发展脉络
Chat Paper
正在生成论文摘要