Dynamic evolution graph attention network for semi-supervised hyperspectral image classification
IEEE Geoscience and Remote Sensing Letters(2024)
摘要
Graph Attention Network (GAT) has a wide range of applications in HSI classification. The GAT-based semi-supervised learning approach enables the integration of valuable information from both labeled and unlabeled samples, effectively reducing the model’s reliance on labeled data. However, the node-wise training approach of GAT often overlooks the inherent global feature of graph data and the long-range dependencies among nodes, thereby limiting the model’s generalization ability on unlabeled data. Therefore, we propose a semi-supervised HSI classification model based on the dynamic evolution graph attention network (DEGAT). The main contributions: 1)We design a dynamic graph evolution mechanism (DGEM) that enables the model to capture the interactive information between local graph attention coefficients and the global graph structure, thus obtaining more discriminative graph representations. 2)DEGAT utilizes the multi-scale mechanism and message-passing mechanism to capture the information of nodes with long-range dependencies, extracting richer spatial-spectral features. State-of-the-art results are achieved with very few labeled training samples on two typical benchmark HSI datasets, where the overall accuracy reaches 95.12% and 98.76% respectively.
更多查看译文
关键词
Hyperspectral image classification,Graph attention network,Dynamic Graph Evolution Mechanism,Semi-supervised classification
AI 理解论文
溯源树
样例
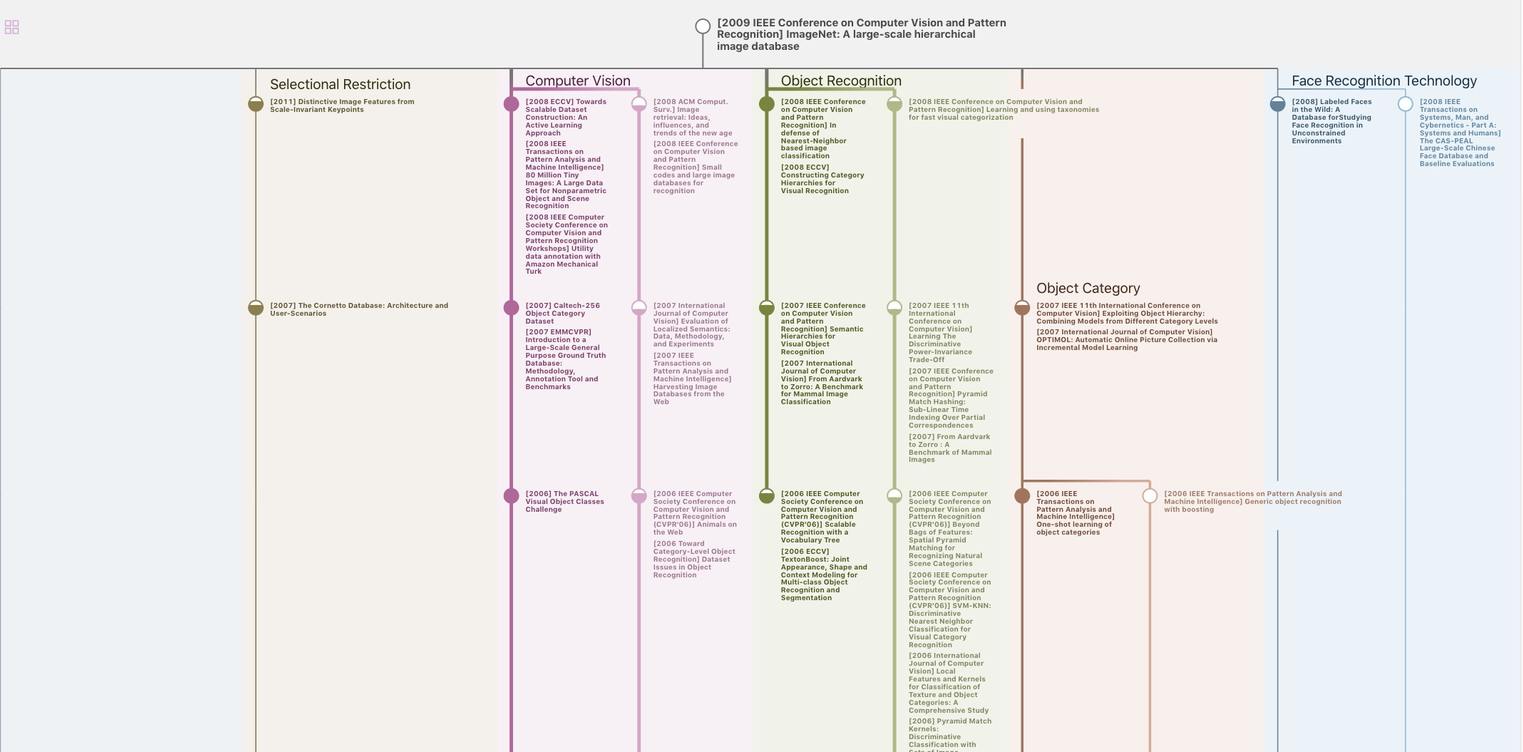
生成溯源树,研究论文发展脉络
Chat Paper
正在生成论文摘要