Spectrum Prediction for Mobile Internet of Things Based on a DB-LSTM Algorithm
IEEE Transactions on Vehicular Technology(2024)
摘要
The fast advancement of 5G mobile communications, artificial intelligence and big data technology has driven the development of the Internet of Things (IoT), while at the same time putting great pressure on existing spectrum resources. Spectrum prediction, as a technology to improve spectrum utilization, faces problems such as poor modeling and ineffective use of historical spectrum sensing data. In addition, the different transmission environments and geographical locations of IoT devices lead to complex and variable wireless mobile channels, which are susceptible to interference from noise and external environments. They lead to inaccurate spectrum sensing results, which can affect the accuracy of spectrum prediction. Therefore, employing long short term memory (LSTM) and bidirectional LSTM (Bi-LSTM) neural networks proposes a dual branch neural network mobile spectrum prediction model (DB-LSTM). The historical sensing data under variable channels are fully utilized to extract forward and backward temporal feature information to overcome the effect of variable environment. First, spectrum sensing using a residual network (ResNet) module and convolutional neural network (CNN) is proposed to extract intrinsic features of signal data and improve the accuracy of spectrum sensing results. This data is employed to train the DB-LSTM model and make for spectrum prediction. The
M
/
G
/1 queuing-based model under
N
-Nakagami channel is used to simulate and generate the signal data set under 2FSK and QPSK modulation methods. The proposed DB-LSTM method is evaluated from three perspectives of accuracy, Error and mean square error (MSE). The results obtained show that compared to the Temporal Convolutional Networks (TCN), Transformer, and Bidirectional Encoder Representations from Transformer (BERT) models, the spectrum prediction accuracy is improved by 2%, 10%, and 1% respectively, the Error is reduced by 29%, 53%, and 4%, respectively, and the MSE is reduced by 23%, 65%, and 4%, respectively.
更多查看译文
关键词
Internet of Things,Cognitive radio network,Spectrum prediction,Spectrum sensing,DB-LSTM
AI 理解论文
溯源树
样例
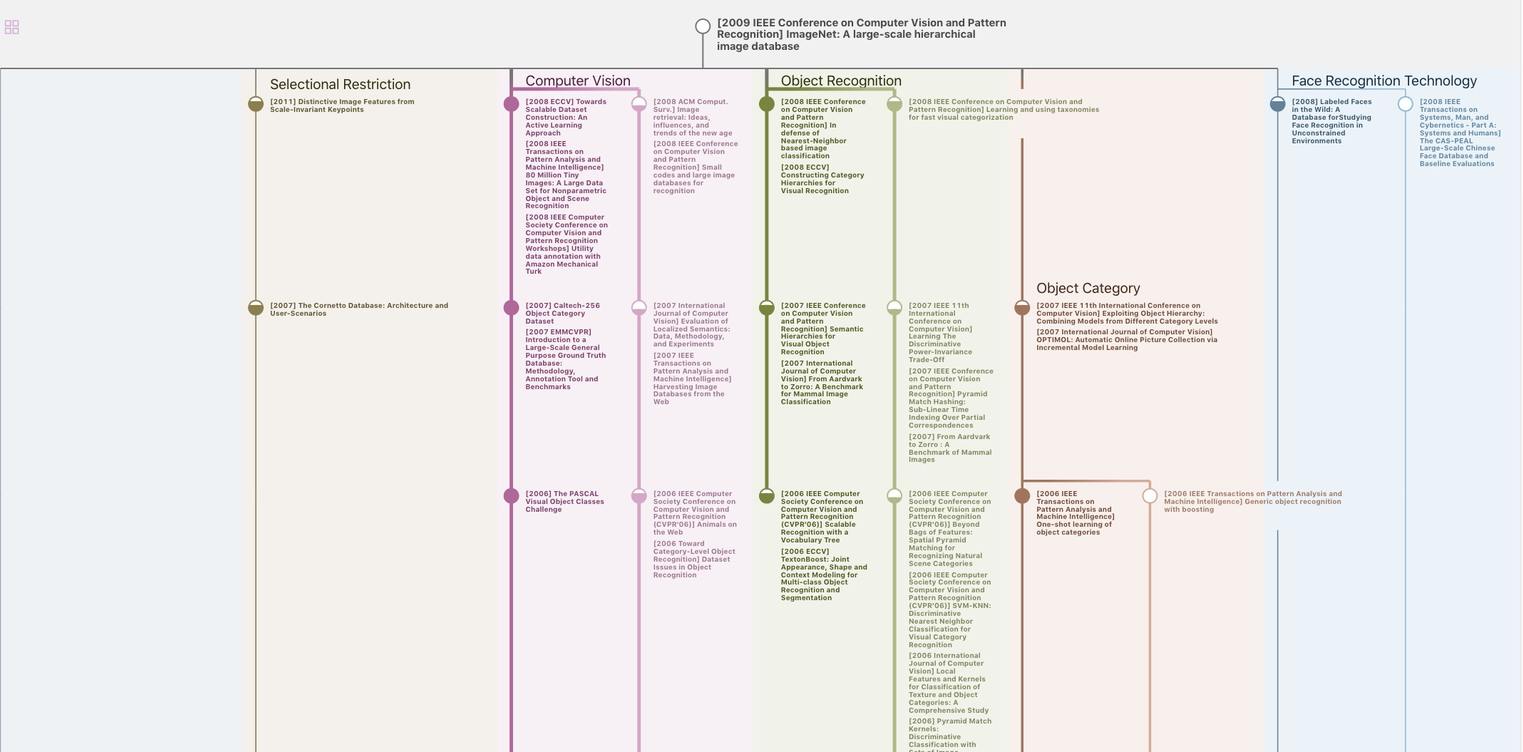
生成溯源树,研究论文发展脉络
Chat Paper
正在生成论文摘要