Validity and Impact of Methods for Collecting Training Data for Myoelectric Prosthetic Control Algorithms.
IEEE transactions on neural systems and rehabilitation engineering : a publication of the IEEE Engineering in Medicine and Biology Society(2024)
摘要
Intuitive regression control of prostheses relies on training algorithms to correlate biological recordings to motor intent. The quality of the training dataset is critical to run-time regression performance, but accurately labeling intended hand kinematics after hand amputation is challenging. In this study, we quantified the accuracy and precision of labeling hand kinematics using two common training paradigms: 1) mimic training, where participants mimic predetermined motions of a prosthesis, and 2) mirror training, where participants mirror their contralateral intact hand during synchronized bilateral movements. We first explored this question in healthy non-amputee individuals where the ground-truth kinematics could be readily determined using motion capture. Kinematic data showed that mimic training fails to account for biomechanical coupling and temporal changes in hand posture. Additionally, mirror training exhibited significantly higher accuracy and precision in labeling hand kinematics. These findings suggest that the mirror training approach generates a more faithful, albeit more complex, dataset. Accordingly, mirror training resulted in significantly better offline regression performance when using a large amount of training data and a non-linear neural network. Next, we explored these different training paradigms online, with a cohort of unilateral transradial amputees actively controlling a prosthesis in real-time to complete a functional task. Overall, we found that mirror training resulted in significantly faster task completion speeds and similar subjective workload. These results demonstrate that mirror training can potentially provide more dexterous control through the utilization of task-specific, user-selected training data. Consequently, these findings serve as a valuable guide for the next generation of myoelectric and neuroprostheses leveraging machine learning to provide more dexterous and intuitive control.
更多查看译文
关键词
Prosthetic Control,Neuroprostheses,Myoelectric Prostheses,Brain-Computer Interfaces,Prosthetic Hand,Machine Learning,Training Data,EMG
AI 理解论文
溯源树
样例
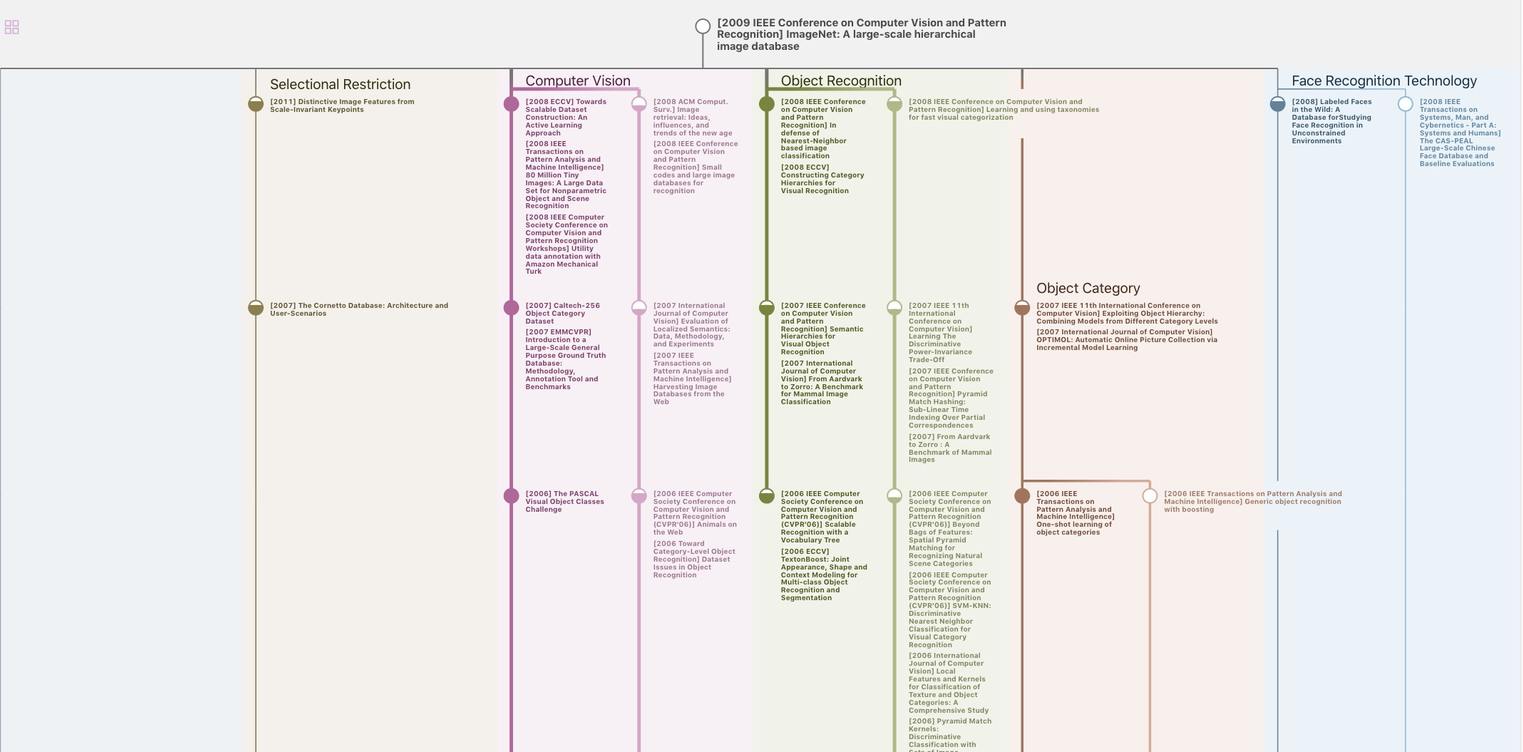
生成溯源树,研究论文发展脉络
Chat Paper
正在生成论文摘要