Potential of Sample Migration and Explainable Machine Learning Model for Monitoring Spatiotemporal Changes of Wetland Plant Communities
IEEE Journal of Selected Topics in Applied Earth Observations and Remote Sensing(2024)
摘要
The composition and dynamics of wetland plant communities play a critical role in maintaining the functionality of wetland ecosystems and serve as important indicators of wetland degradation and restoration. Accurately identifying wetland plant communities using remote sensing techniques remains challenging due to the complex environment and cloud contamination. Here, we applied a sample migration method based on change vector analysis and a random forest (RF) classifier incorporating SHapley Additive exPlanations (SHAP) to explore the spatiotemporal changes of wetland plant communities in the western Songnen Plain of China between 2016 and 2022, and to better understand the decision logic of the RF model. Our work achieved accurate annual wetland classification at the community scale, with an average overall accuracy of 89.5% and an average kappa coefficient of 0.87. Our analysis revealed different spatiotemporal change characteristics of wetland plant communities in the western Songnen Plain and three national nature reserves. The SHAP model showed that MOS_IRECI is the most important feature determining the prediction results of the RF model, and the importance of the features differs at global and local levels. This study confirms the feasibility of annual dynamic monitoring of wetland plant communities at a regional scale. The results are expected to provide a reference for the fine and sustainable management of wetland resources in the western Songnen Plain, as well as valuable data support for related wetland ecology research.
更多查看译文
关键词
Wetland plant communities,Google Earth Engine,random forest,sample migration,SHapley Additive exPlanations
AI 理解论文
溯源树
样例
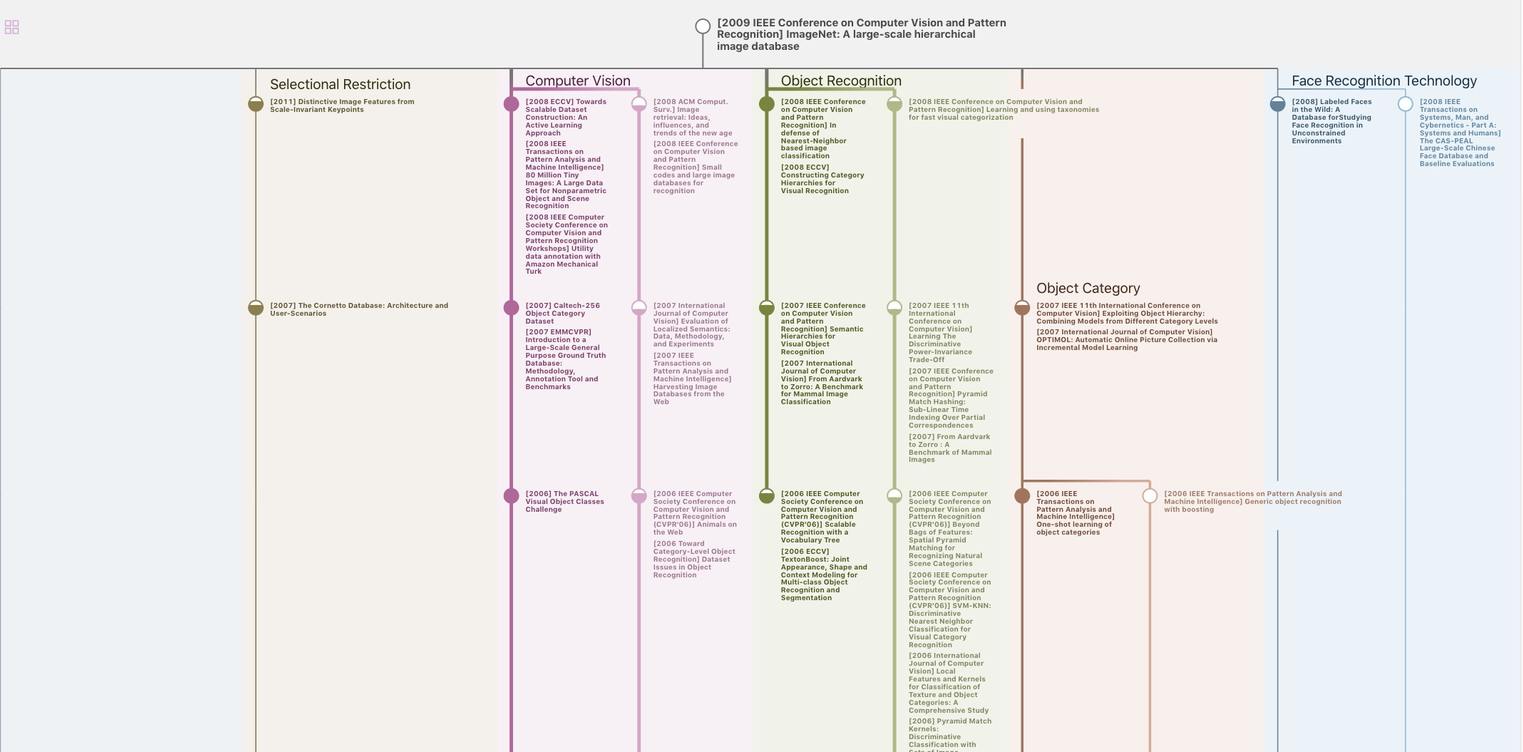
生成溯源树,研究论文发展脉络
Chat Paper
正在生成论文摘要