Towards Visually Interpreting Variational Autoencoders
crossref(2024)
摘要
Abstract Recent advances in convolutional neural network (CNN) interpretability have led to a wide-variety of gradient-based visual attention techniques for generating visual attention maps. However, most of these methods require a classification-type design architecture, and consequently concentrate on classification/categorization-type tasks. Extending these methods to generate visual attention maps for other kinds of computer vision models, e.g., variational autoencoders (VAE) is not trivial. In this paper, we present a method that helps bridge this crucial gap, proposing to compute VAE attention as a means for interpreting the latent space learned by a VAE. We first present methods to generate visual attention maps from the learned latent space, and then show how they can be used in a variety of applications: localizing anomalies in images, including medical imagery, and improved latent space disentanglement. We conduct extensive experiments on a wide-variety of benchmark datasets to demonstrate the efficacy of the proposed VAE attention.
更多查看译文
AI 理解论文
溯源树
样例
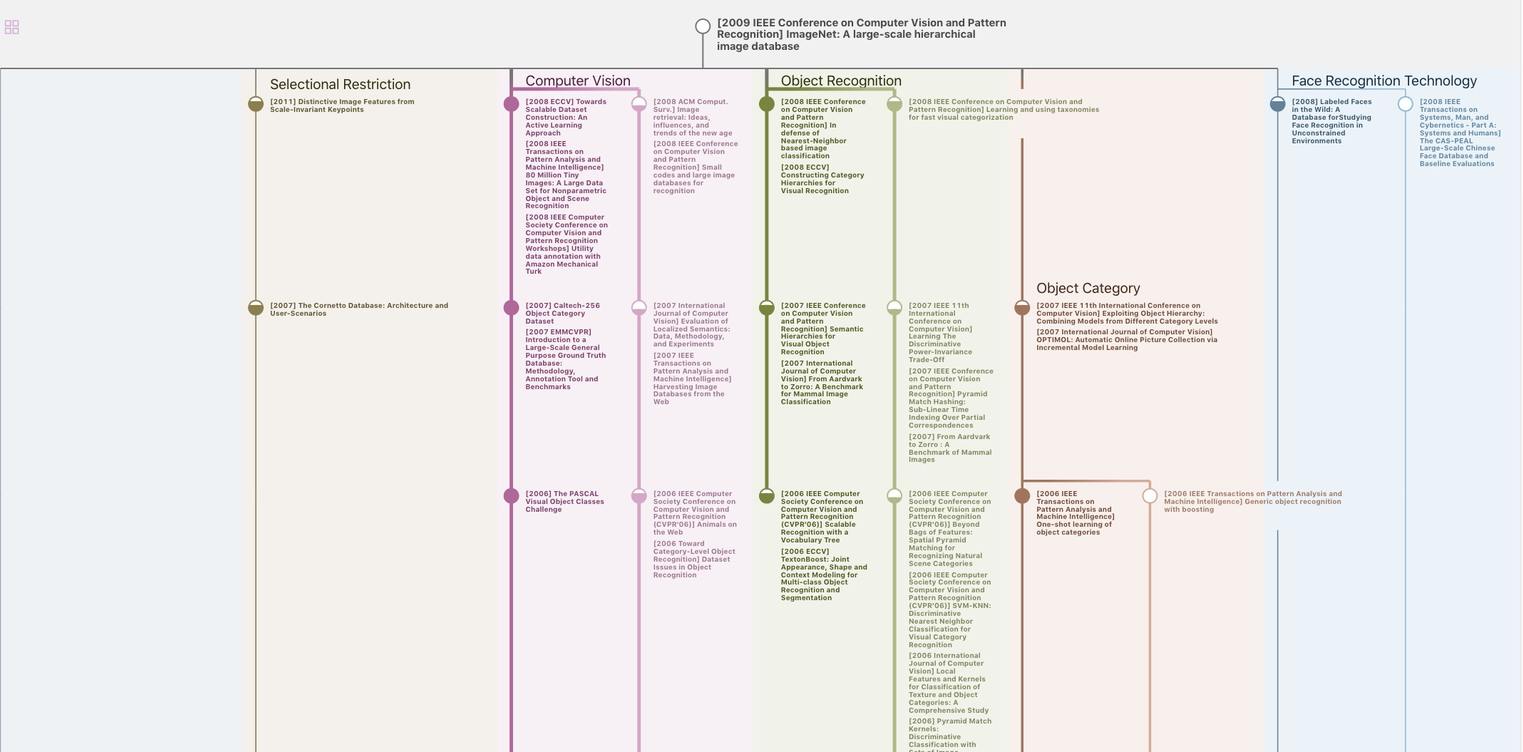
生成溯源树,研究论文发展脉络
Chat Paper
正在生成论文摘要