DeFRCN-MAM: DeFRCN and multi-scale attention mechanism-based industrial defect detection method
Applied Artificial Intelligence(2024)
摘要
ABSTRACTWith the technology development, industrial defect detection based on deep learning has attracted extensive attention in the academic community. Different from general visual objects, industrial defects have the characteristics of small sample, weak visibility and irregular shape, which hinder the application of related studies. According to these problems, a few-shot object detection (FSOD) method based on Decoupled Faster R-CNN (DeFRCN) is proposed in this paper. Firstly, it includes fine-tuning processing, because of the small sample characteristics. To adapt to the invisible characteristics of defects, we introduce the Feature Pyramid Network (FPN) and Residual Attention Module (RAM) into DeFRCN, which can enhance the capture ability of multi-scale features and feature association information. Furthermore, the feature representation ability is strengthened by parallel connecting of two channels, consisting of R-CNN head, box classifier and box regression models. Finally, it is completed that the pre-training, fine-tuning and testing of the proposed network, with DAGM 2007 and NEU-DET public industrial defect datasets as the base class and flange shaft defect data collected in the laboratory as the new class. To verify the effectiveness of the proposed one, we compare them with other classical FSOD methods. The superiority of the proposed method is obvious.
更多查看译文
AI 理解论文
溯源树
样例
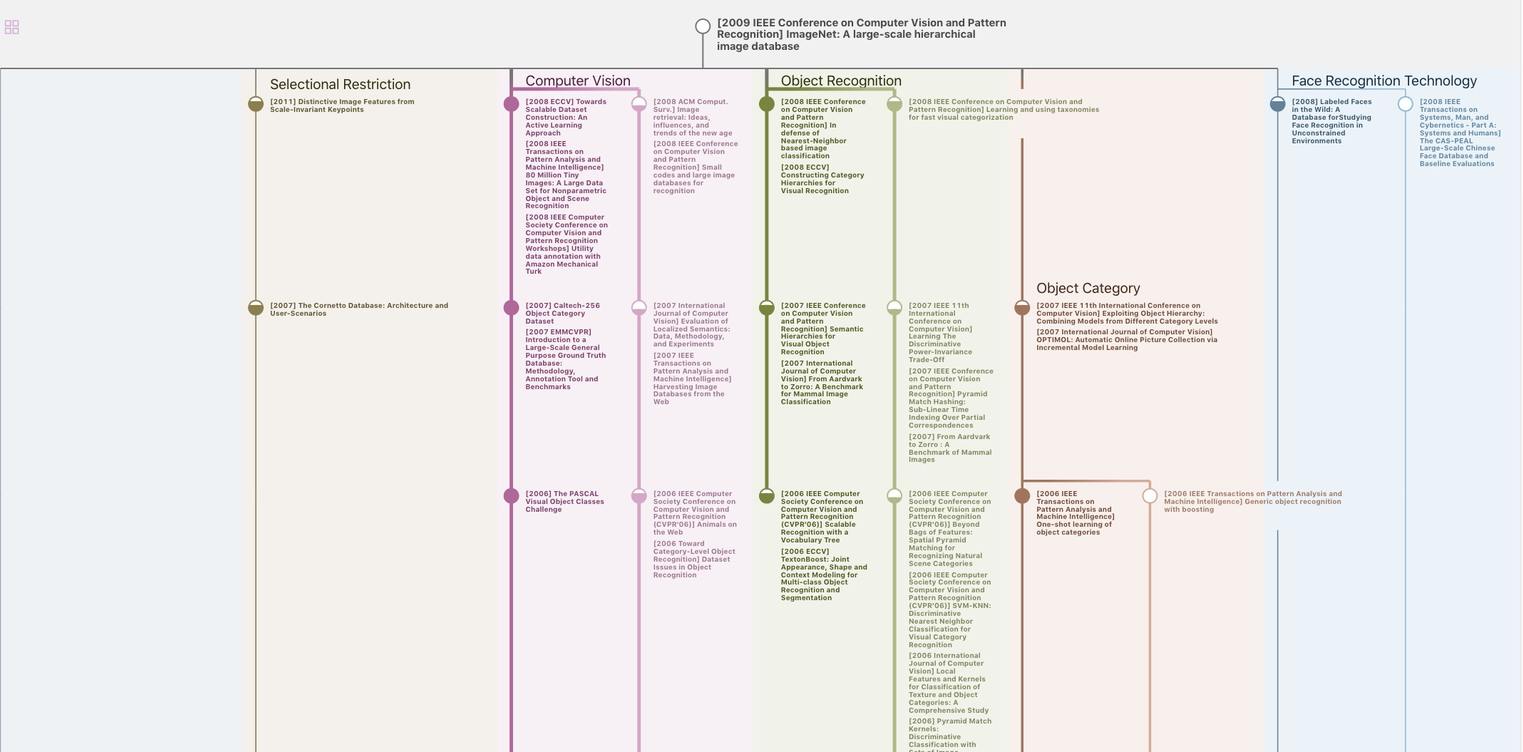
生成溯源树,研究论文发展脉络
Chat Paper
正在生成论文摘要