Dynamic Resource Allocation in IoT Enhanced by Digital Twins and Intelligent Reflecting Surfaces
IEEE Internet of Things Journal(2024)
摘要
Effectively managing network resources in the complex and ever-evolving realms of Internet of Things (IoT) ecosystems presents a formidable challenge. Conventional resource allocation methods often grapple with adapting to the dynamic nature of IoT environments, resulting in suboptimal performance and delayed responsiveness. The advent of stateof-the-art network technologies, notably intelligent reflecting surfaces (IRSs), further amplifies complexity, particularly in optimizing IRS configurations within dynamic networks. To address these intricacies, this paper introduces DTRiD, a fusion framework uniting digital twins (DTs), IRSs, and deep deterministic policy gradient (DDPG) to tackle these challenges. DTRiD offers a distinctive amalgamation of DTs, IRS functionalities, and DDPGs, all aimed at augmenting communication and resource allocation within the IoT. By leveraging real-world data from wireless networks, DTRiD compiles a diverse dataset encapsulating various network conditions. Extensive simulations showcase the frameworks superiority over conventional methodologies across key metrics, including accuracy, convergence, delay reduction, and energy efficiency in multiple dimensions. These results show the potential of DTRiD in restructuring the landscape of IoT communication by optimizing resource allocation dynamically, enabling it to handle complex scenarios and adapt to diverse environmental changes.
更多查看译文
关键词
Intelligent reflecting surface,deep deterministic policy gradient,digital twin,Internet of Things,6G
AI 理解论文
溯源树
样例
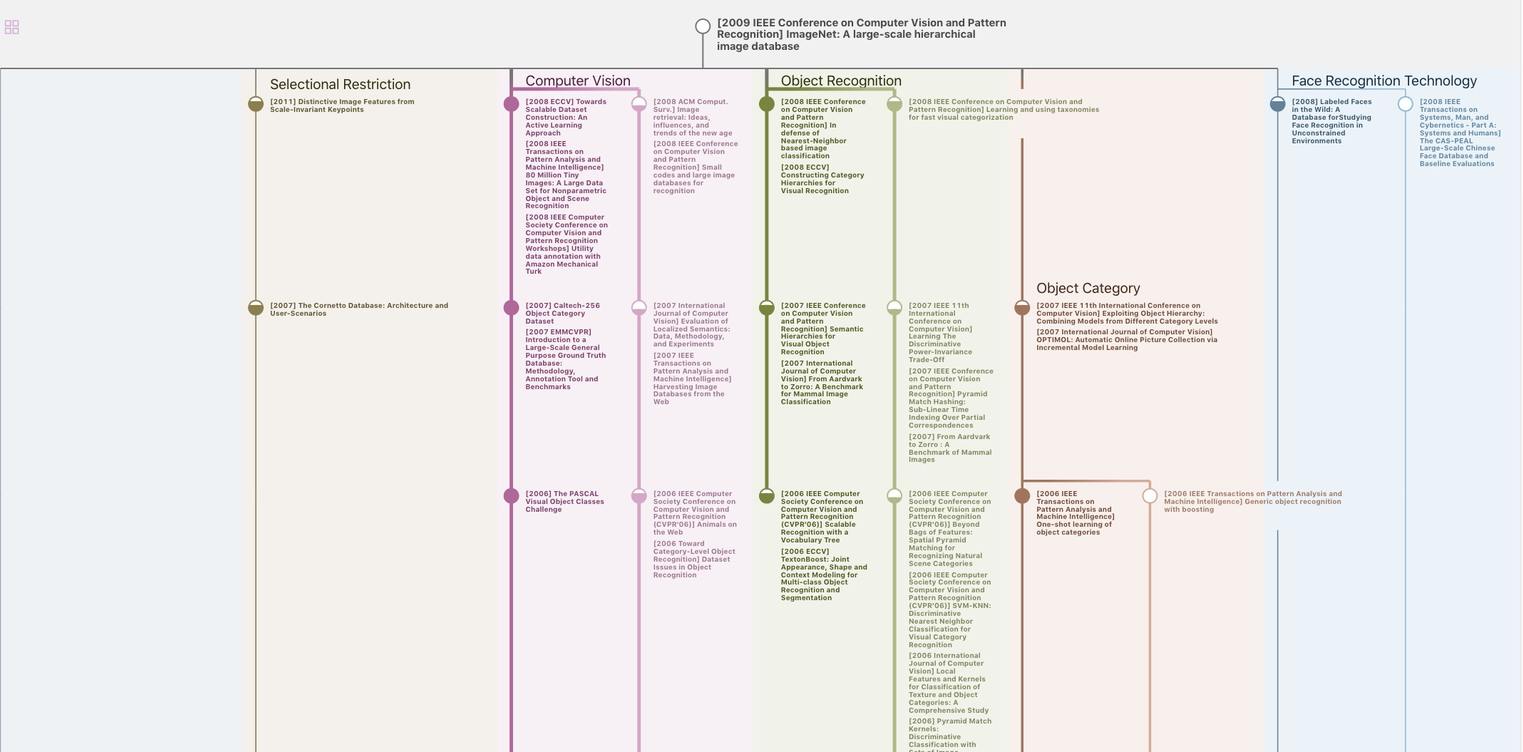
生成溯源树,研究论文发展脉络
Chat Paper
正在生成论文摘要