Decentralized Adaptive TD() Learning With Linear Function Approximation: Nonasymptotic Analysis
IEEE TRANSACTIONS ON SYSTEMS MAN CYBERNETICS-SYSTEMS(2024)
摘要
In multiagent reinforcement learning, policy evaluation is a central problem. To solve this problem, decentralized temporal-difference (TD) learning is one of the most popular methods, which has been investigated in recent years. However, existing decentralized variants of TD learning often suffer from slow convergence due to the sensitive selection of learning rates. Inspired by the great success of adaptive gradient methods in the training of deep neural networks, this article proposes a decentralized adaptive TD (lambda) learning algorithm for general lambda with linear function approximation, referred to as D-AMSTD(lambda), which can mitigate the selective sensitivity of learning rates. Furthermore, we establish the finite-time performance bounds of D-AMSTD(lambda) under the Markovian observation model. The theoretical results show that D-AMSTD(lambda) can linearly converge to an arbitrarily small size of neighborhood of the optimal weight. Finally, we verify the efficacy of D-AMSTD(lambda) through a variety of experiments. The results show that D-AMSTD(lambda) outperforms existing decentralized TD learning methods.
更多查看译文
关键词
Finite-time bounds,multiagent reinforcement learning (MARL),policy evaluation,temporal-difference (TD) learning
AI 理解论文
溯源树
样例
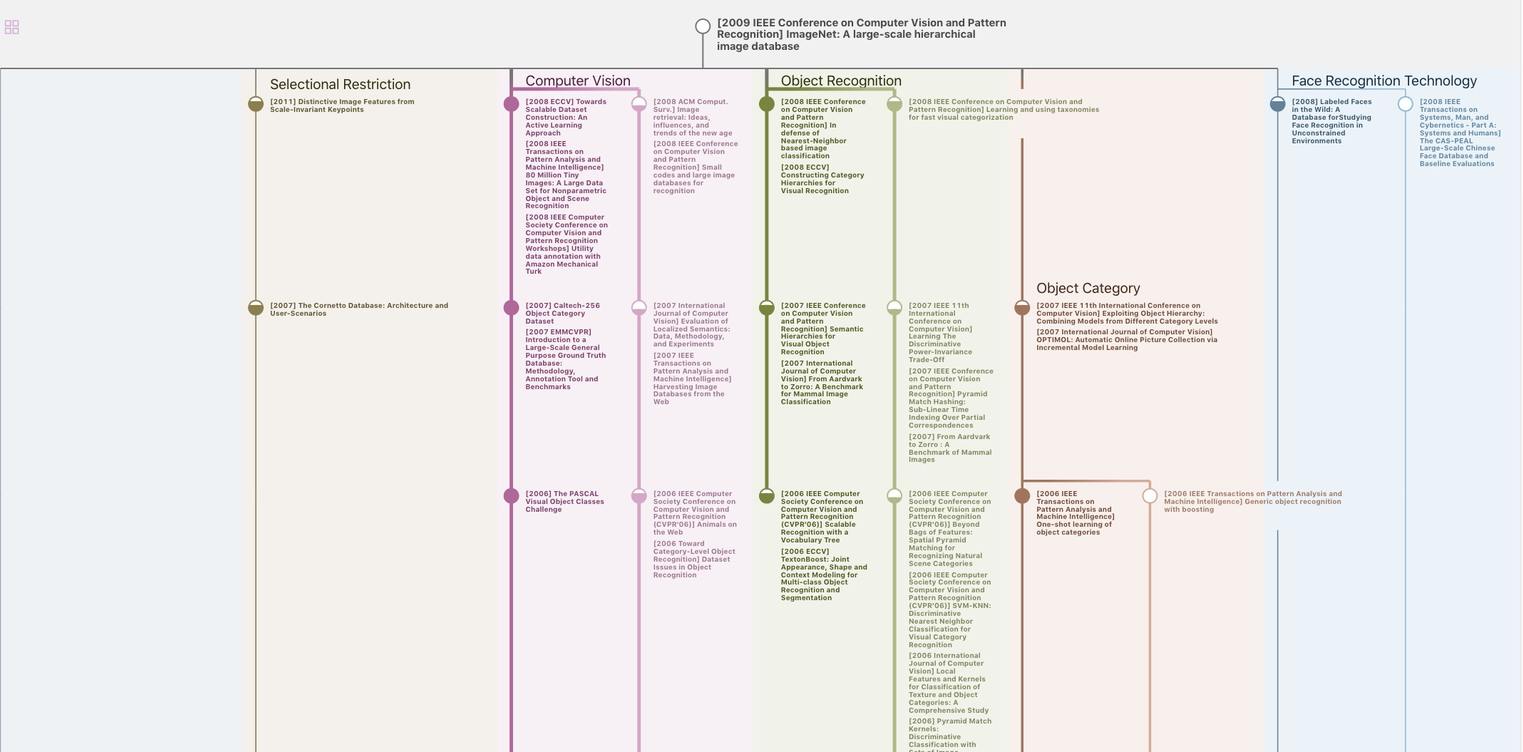
生成溯源树,研究论文发展脉络
Chat Paper
正在生成论文摘要