Data-driven approaches for fatigue prediction of Ti-6Al-4V parts fabricated by laser powder bed fusion
INTERNATIONAL JOURNAL OF FATIGUE(2024)
摘要
This study introduces an innovative methodology for predicting fatigue properties of additively manufactured Titanium alloy (Ti-6Al-4V) fabricated using laser powder bed fusion (LPBF). This study entails two key components: a classification model to identify sites likely to initiate cracks and a Probabilistic Physics -Guided Neural Network (PPgNN 2.0) to forecast fatigue life. The classification model capitalizes on parameters related to surface roughness and internal defects. This methodology minimizes false positives and negatives by leveraging computed tomography (CT) images for accurate surface topology and internal defects morphology. Data augmentation techniques were employed to address the challenge of imbalanced data distribution. PPgNN 2.0 represents an advancement over classical regression models due to its incorporation of physics -based constraints and probabilistic approaches, leading to highly accurate and physically consistent predictions. Training of the model involves multiple stress-fatigue life (S-N) curve datasets from literature and pertinent morphological data, while evaluation uses data extracted from in-house testing and imaging. The key strength of PPgNN 2.0 lies in its ability to offer flexible and robust fatigue life predictions, encompassing point estimates and uncertainties in the form of mean and standard deviation. A new loss function is proposed, which effectively captures the underlying distribution, contributing to minimizing prediction errors.
更多查看译文
关键词
Additive manufacturing,Ti-6Al-4V,Fatigue life prediction,Uncertainty quantification,Crack initiation
AI 理解论文
溯源树
样例
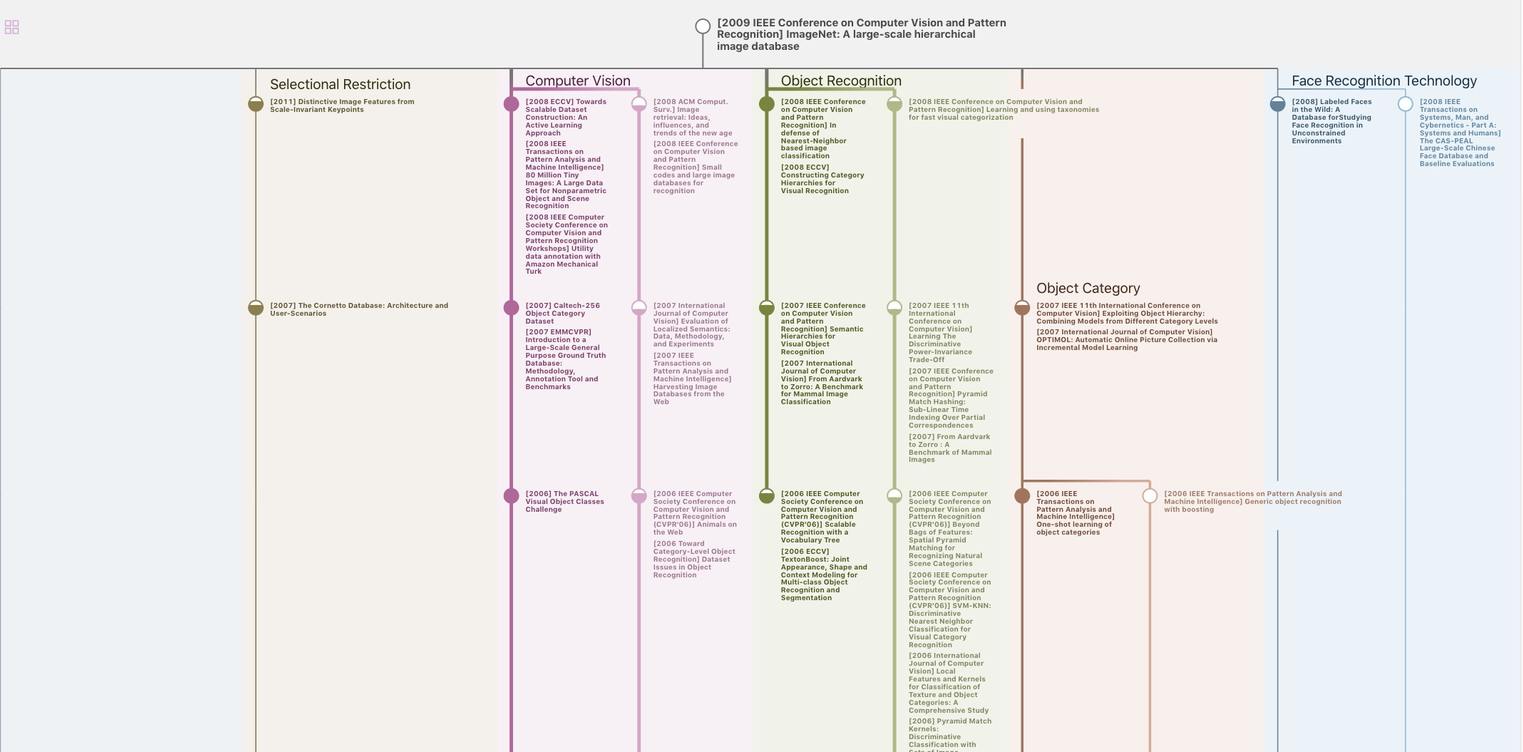
生成溯源树,研究论文发展脉络
Chat Paper
正在生成论文摘要