Bessel Function Mixture Model for Localization in Generalized <inline-formula><tex-math notation="LaTeX">$\eta\text{-}\mu$</tex-math></inl ine-formula> IoT Fading Environment
IEEE TRANSACTIONS ON NETWORK SCIENCE AND ENGINEERING(2024)
摘要
The effectiveness of localization techniques that rely on Received Signal Strength (RSS) is impeded by the existence of small-scale fading and non-line-of-sight (NLOS) phenomena. Therefore, it is crucial to accurately model the fading distribution when estimating the location of smart devices. The utilisation of a generic fading model confers a significant advantage owing to its capacity to accommodate a variety of fading distributions across diverse environments. This work introduces a new approach for device localization in a connected Internet of Things (IoT) setting, utilising a generic $\eta\text{-}\mu$ fading model for RSS. We propose a Bessel Function mixture model that is utilised to estimate the position of the device, taking into account a generic $\eta\text{-}\mu$ fading model. A log-Sigmoid weight function is employed to combine large and small approximations for the modified Bessel function of the first kind. The position coordinates are estimated through a gradient ascent that seeks to optimise the derived maximum likelihood (ML) function. The method under consideration is evaluated in three distinct settings, namely an authentic indoor environment, a simulated $\eta\text{-}\mu$ fading environment, and an outdoor testbed. Our findings demonstrate the effectiveness of the generic fading models, surpassing current state-of-the-art methodologies.
更多查看译文
关键词
Location awareness,Rayleigh channels,Computational modeling,Maximum likelihood estimation,Mixture models,Probability density function,Internet of Things,Generalized fading,localization,modified bessel function
AI 理解论文
溯源树
样例
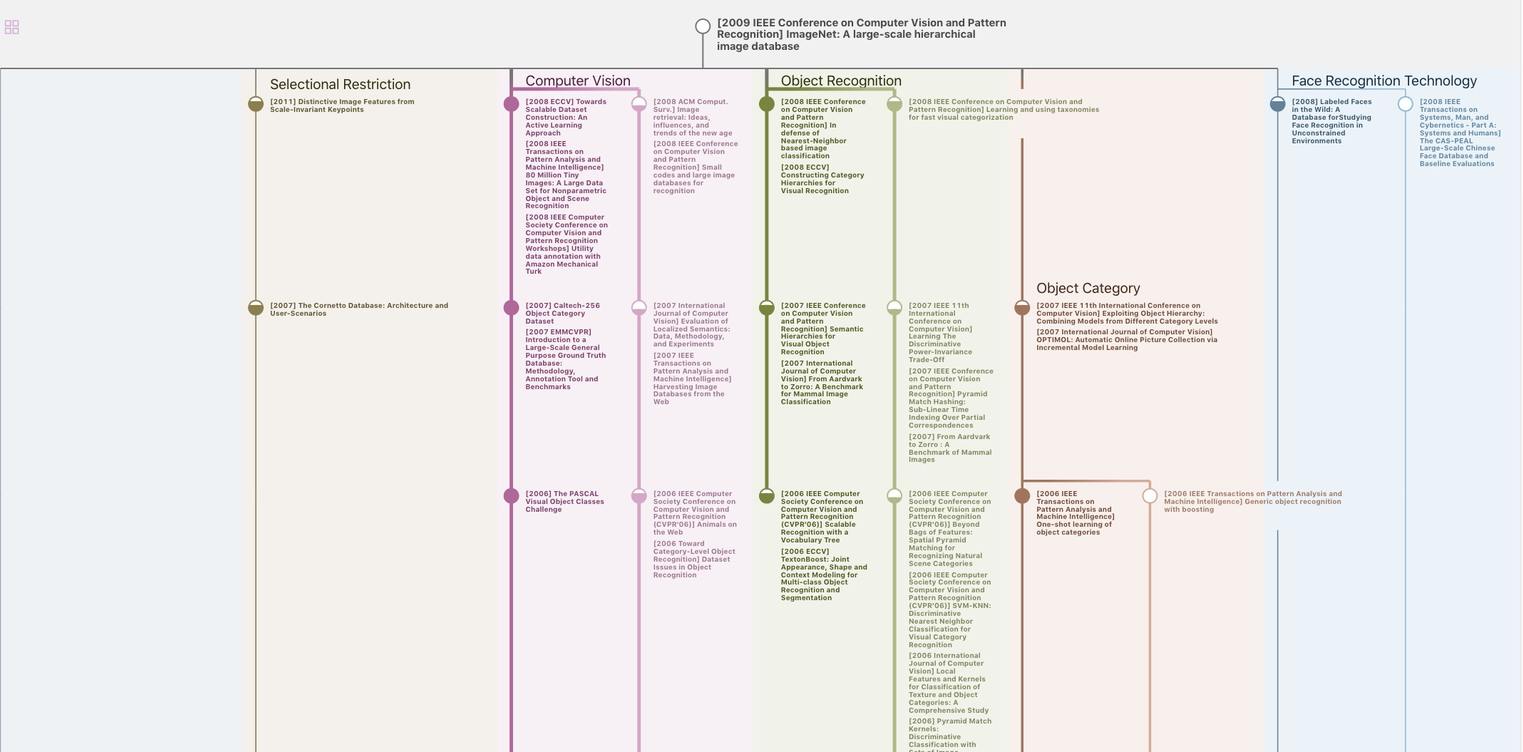
生成溯源树,研究论文发展脉络
Chat Paper
正在生成论文摘要