Category Alignment Mechanism for Few-Shot Image Classification.
IEEE transactions on neural networks and learning systems(2024)
摘要
While humans can excel at image classification tasks by comparing a few images, existing metric-based few-shot classification methods are still not well adapted to novel tasks. Performance declines rapidly when encountering new patterns, as feature embeddings cannot effectively encode discriminative properties. Moreover, existing matching methods inadequately utilize support set samples, focusing only on comparing query samples to category prototypes without exploiting contrastive relationships across categories for discriminative features. In this work, we propose a method where query samples select their most category-representative features for matching, making feature embeddings adaptable and category-related. We introduce a category alignment mechanism (CAM) to align query image features with different categories. CAM ensures features chosen for matching are distinct and strongly correlated to intra-and inter-contrastive relationships within categories, making extracted features highly related to their respective categories. CAM is parameter-free, requires no extra training to adapt to new tasks, and adjusts features for matching when task categories change. We also implement a cross-validation-based feature selection technique for support samples, generating more discriminative category prototypes. We implement two versions of inductive and transductive inference and conduct extensive experiments on six datasets to demonstrate the effectiveness of our algorithm. The results indicate that our method consistently yields performance improvements on benchmark tasks and surpasses the current state-of-the-art methods.
更多查看译文
关键词
Category alignment mechanism (CAM),feature selection,few-shot classification,metric-based
AI 理解论文
溯源树
样例
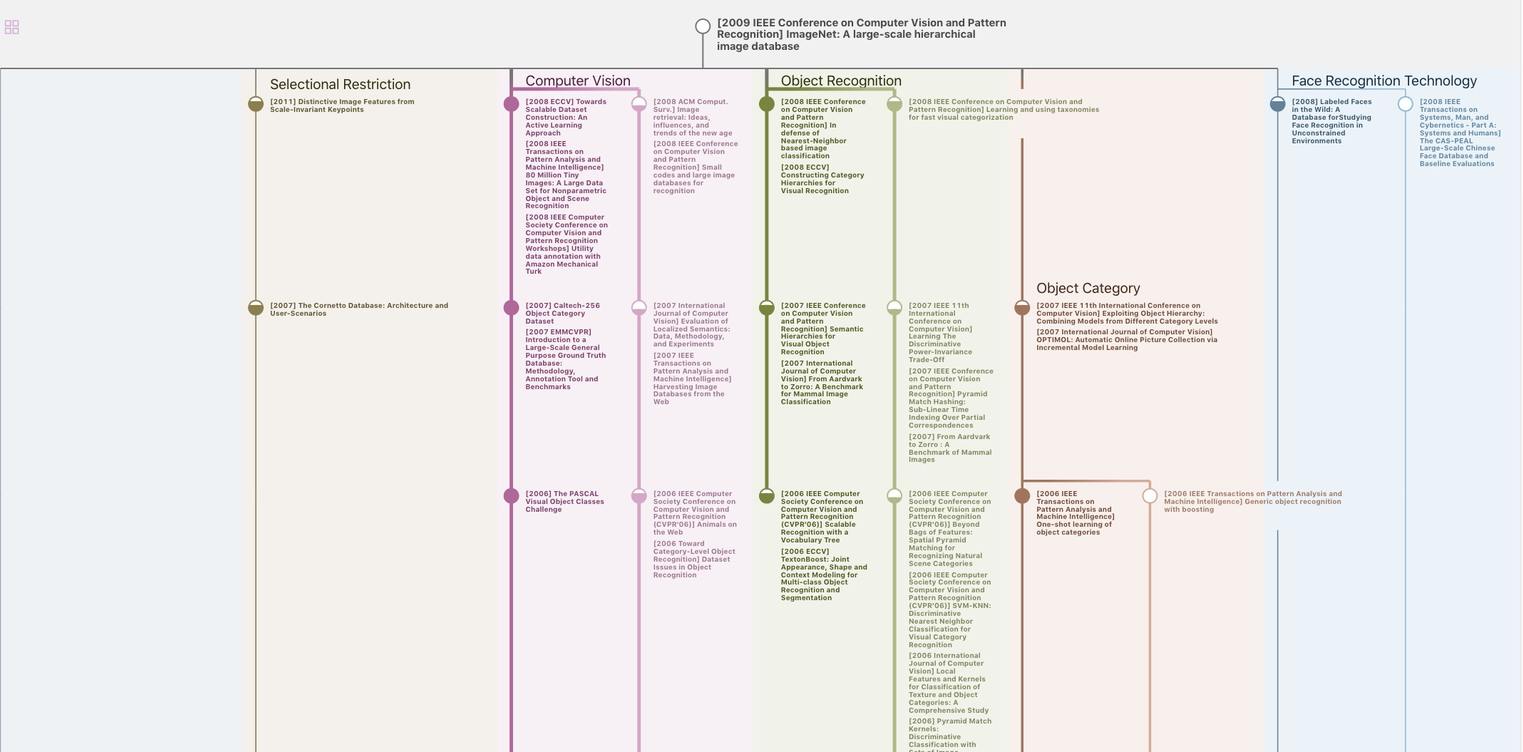
生成溯源树,研究论文发展脉络
Chat Paper
正在生成论文摘要