Discriminating Spectral-Spatial Feature Extraction for Hyperspectral Image Classification: A Review.
Sensors (Basel, Switzerland)(2024)
摘要
Hyperspectral images (HSIs) contain subtle spectral details and rich spatial contextures of land cover that benefit from developments in spectral imaging and space technology. The classification of HSIs, which aims to allocate an optimal label for each pixel, has broad prospects in the field of remote sensing. However, due to the redundancy between bands and complex spatial structures, the effectiveness of the shallow spectral-spatial features extracted by traditional machine-learning-based methods tends to be unsatisfying. Over recent decades, various methods based on deep learning in the field of computer vision have been proposed to allow for the discrimination of spectral-spatial representations for classification. In this article, the crucial factors to discriminate spectral-spatial features are systematically summarized from the perspectives of feature extraction and feature optimization. For feature extraction, techniques to ensure the discrimination of spectral features, spatial features, and spectral-spatial features are illustrated based on the characteristics of hyperspectral data and the architecture of models. For feature optimization, techniques to adjust the feature distances between classes in the classification space are introduced in detail. Finally, the characteristics and limitations of these techniques and future challenges in facilitating the discrimination of features for HSI classification are also discussed further.
更多查看译文
关键词
hyperspectral image classification,discriminating spectral–spatial features,feature extraction,feature optimization
AI 理解论文
溯源树
样例
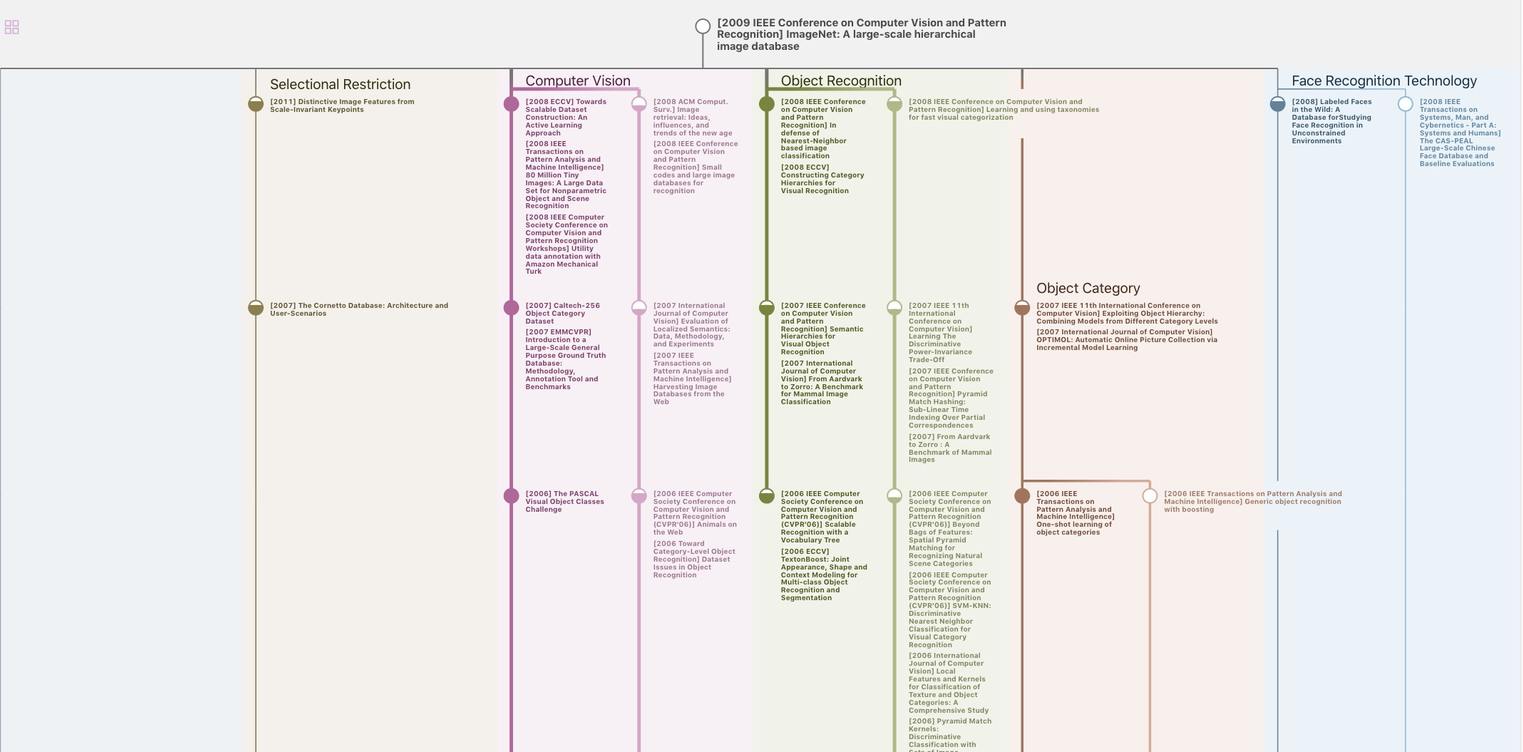
生成溯源树,研究论文发展脉络
Chat Paper
正在生成论文摘要