Misaligned Visible-Thermal Object Detection: A Drone-based Benchmark and Baseline
IEEE Transactions on Intelligent Vehicles(2024)
摘要
Multispectral object detection has achieved remarkable results due to its ability to fuse information from visible and thermal modalities in recent years. However, the existing visible-thermal datasets are constructed based on manually aligned image pairs, which cannot fully represent the challenges of real-world scenarios where image pairs are often misaligned. Existing methods for visible-thermal object detection are based on aligned data and are limited by the accuracy of registration. To address the above issues, we propose a dataset, namely DVTOD, which is a misaligned visible-thermal object detection dataset captured by drones. DVTOD includes 16 challenging attributes and 54 capture scenes. Furthermore, we introduce a cross-modal alignment detector (CMA-Det) for misaligned visible-thermal object detection. Firstly, we design an alignment network to estimate the visible-to-thermal deformation field, which is used to correct for misalignment of the corresponding visible and thermal features. Secondly, we propose a strategy called Object Search Rectification (OSR) to improve the robustness of feature alignment. To better remove the interference of complex backgrounds, a bi-directional feature correction fusion module (BFCFM) is designed to calibrate bimodal features by exploiting the correlation of channel and spatial information between two modalities. CMA-Det outperforms existing methods on the DVTOD dataset and two other visible-thermal object detection datasets. The dataset and code will be published at
https://github.com/VDT-2048/DVTOD
.
更多查看译文
关键词
Multispectral object detection,visible-thermal dataset,cross-modal alignment,feature alignment
AI 理解论文
溯源树
样例
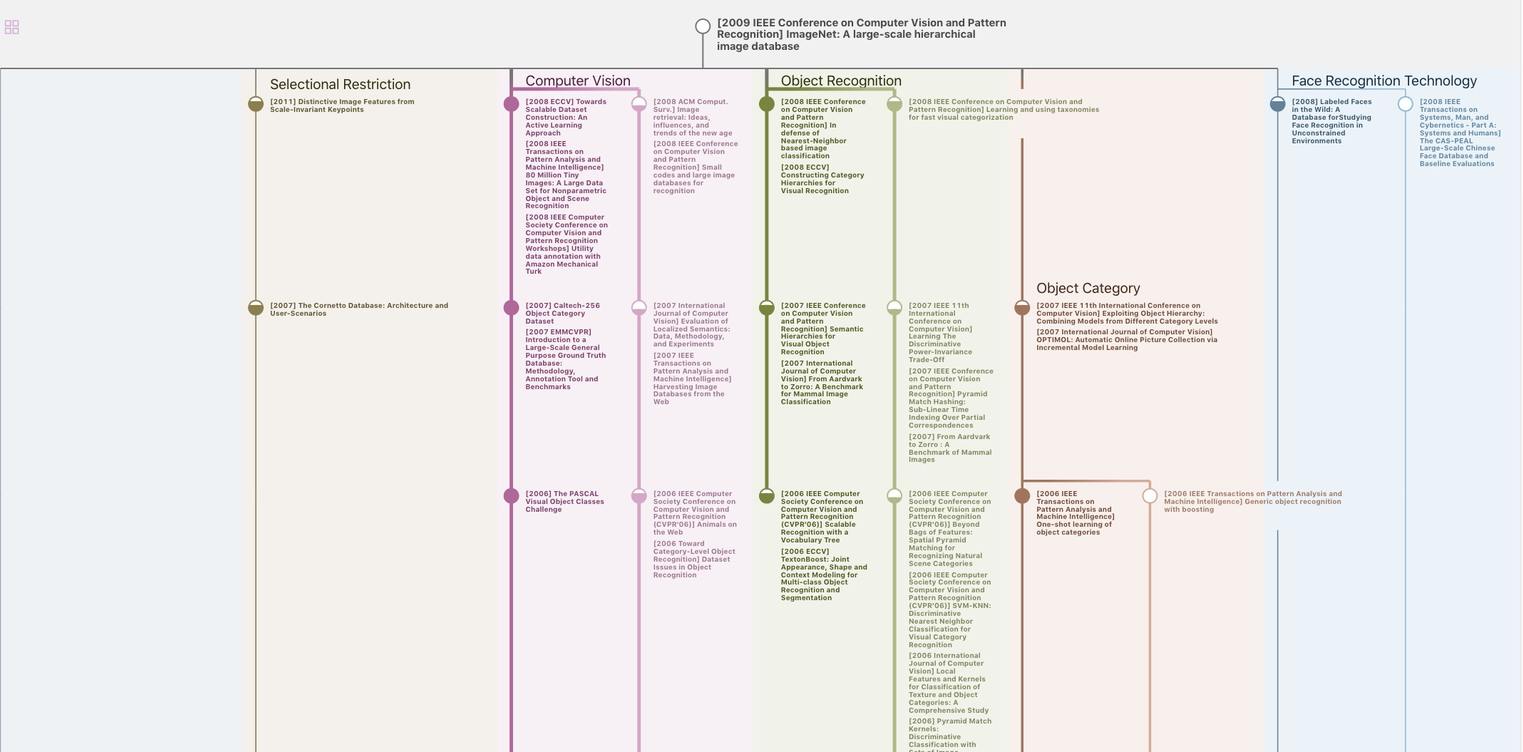
生成溯源树,研究论文发展脉络
Chat Paper
正在生成论文摘要