T 3 RD: Test-Time Training for Rumor Detection on Social Media.
WWW 2024(2024)
摘要
With the increasing number of news uploaded to the internet daily, rumor detection has garnered significant attention in recent years. Existing rumor detection methods excel on familiar topics with sufficient training data (high resource) collected from the same domain. However, when facing emergent events or rumors propagated in different languages, the performance of these models is significantly degraded, due to the lack of training data and prior knowledge (low resource). To tackle this challenge, we introduce the Test-Time Training for Rumor Detection (T^3RD) to enhance the performance of rumor detection models on low-resource datasets. Specifically, we introduce self-supervised learning (SSL) as an auxiliary task in the test-time training. It consists of global and local contrastive learning, in which the global contrastive learning focuses on obtaining invariant graph representations and the local one focuses on acquiring invariant node representations. We employ the auxiliary SSL tasks for both the training and test-time training phases to mine the intrinsic traits of test samples and further calibrate the trained model for these test samples. To mitigate the risk of distribution distortion in test-time training, we introduce feature alignment constraints aimed at achieving a balanced synergy between the knowledge derived from the training set and the test samples. The experiments conducted on the two widely used cross-domain datasets demonstrate that the proposed model achieves a new state-of-the-art in performance. Our code is available at https://github.com/social-rumors/T3RD.
更多查看译文
AI 理解论文
溯源树
样例
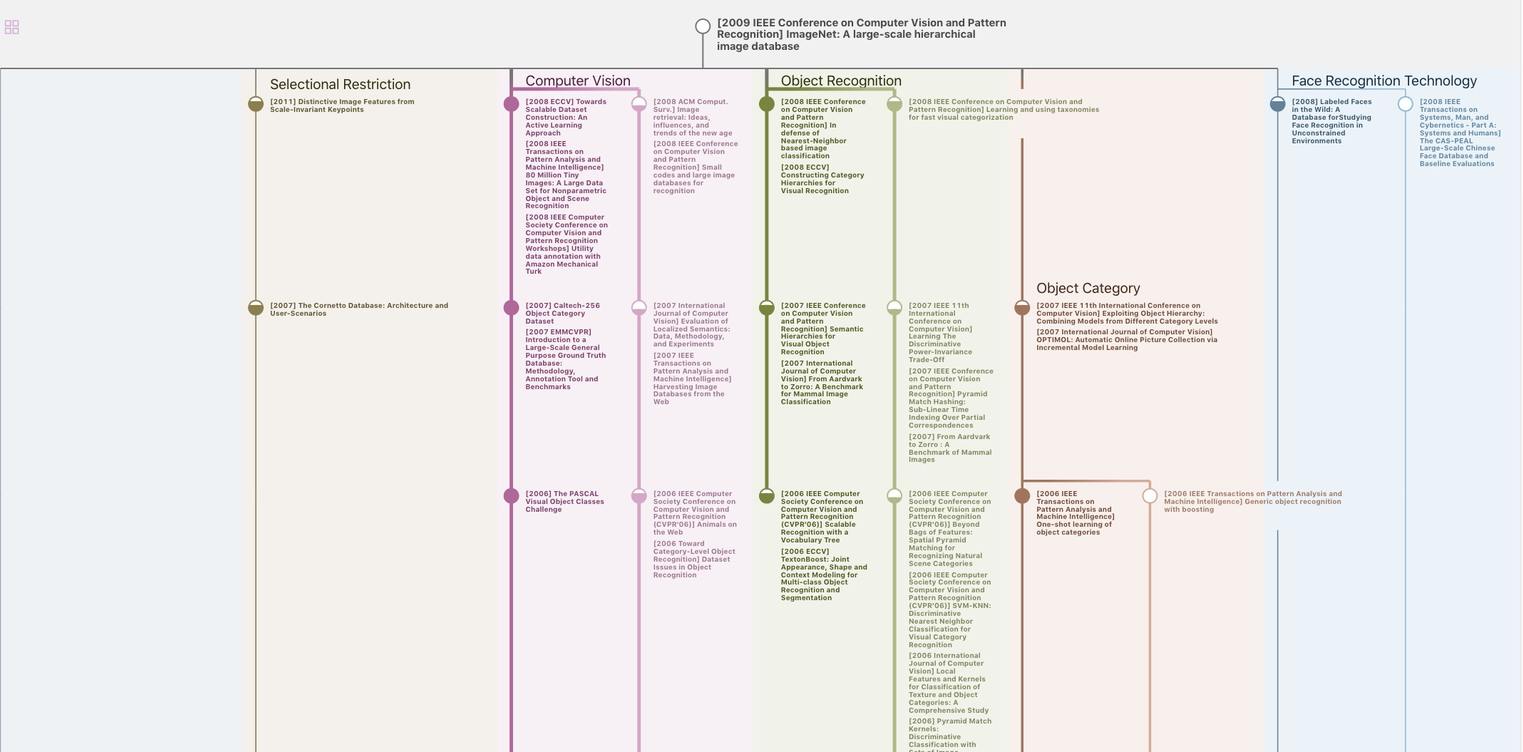
生成溯源树,研究论文发展脉络
Chat Paper
正在生成论文摘要