Hypersonic Inlet Flow Field Reconstruction Dominated by Shock Wave and Boundary Layer Based on Small Sample Physics-informed Neural Networks
Aerospace Science and Technology(2024)
摘要
High Mach number inlets face complex challenges such as shock wave/boundary layer interference, adversely impacting the aerodynamic characteristics and stable operating boundaries. Traditional computational fluid dynamics (CFD) simulations for performance and flow field analysis are slow, and data-driven deep learning technologies struggle with predicting flow fields in complex aerodynamic phenomena like shock wave/boundary layer interactions, boundary layer separation, and Mach disks. This study introduces a rapid, robust method for reconstructing hypersonic viscous inlet flow fields using inlet geometry design parameters. This method is called shock wave and boundary layer dominated two-dimensional multiphysical field reconstruction model based on multi-scale sensory field fusion residual physical information neural network (PIMSRF_ResNet), ensuring high-precision training data. Using the optimal Pareto solution set from multi-objective optimization with a small sample intelligence method, 150 sets of multi-physics fields under Ma10 conditions with varying geometric design parameters are calculated. Compared to traditional pure data-driven models, PIMSRF_ResNet's structural similarity in the test set reaches 0.9773, with a peak signal-to-noise ratio close to 30 dB and a correlation coefficient exceeding 98%. These experimental results demonstrated that the proposed method is a promising tool for real-time prediction of complex internal flow fields dominated by high Mach number shock waves and boundary layers.
更多查看译文
关键词
Hypersonic inlet,Physics-informed neural networks,Small sample,Shock wave/boundary layer interaction
AI 理解论文
溯源树
样例
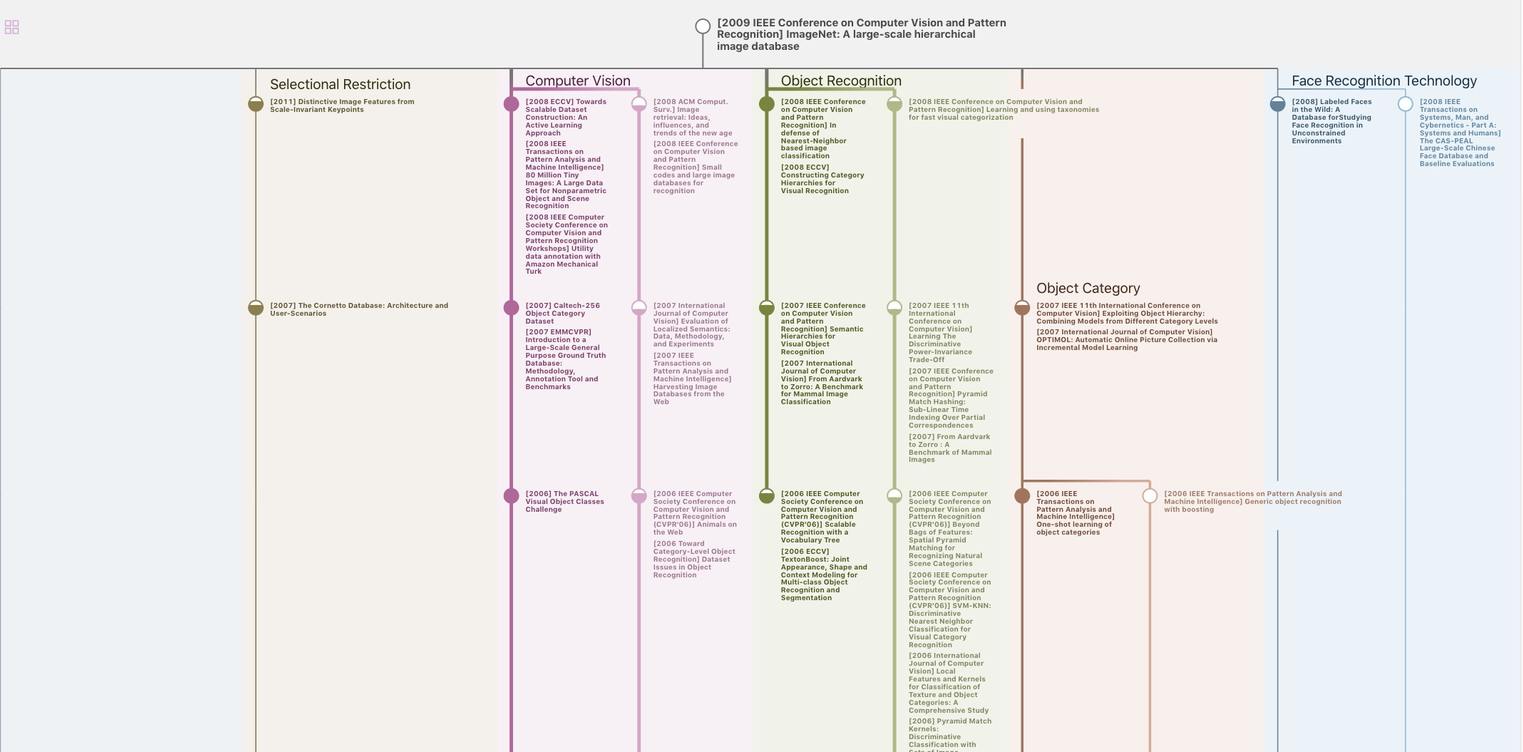
生成溯源树,研究论文发展脉络
Chat Paper
正在生成论文摘要