Two-Stage Adaptive Memetic Algorithm with Surprisingly Popular Mechanism for Energy-Aware Distributed Hybrid Flow Shop Scheduling Problem with Sequence-Dependent Setup Time
Complex System Modeling and Simulation(2024)
摘要
This paper considers the impact of setup time in production scheduling and proposes energy-aware distributed hybrid flow shop scheduling problem with sequence-dependent setup time (EADHFSP-ST) that simultaneously optimizes the makespan and the energy consumption. We develop a mixed integer linear programming model to describe this problem and present a two-stage adaptive memetic algorithm (TAMA) with a surprisingly popular mechanism. First, a hybrid initialization strategy is designed based on the two optimization objectives to ensure the convergence and diversity of solutions. Second, multiple population co-evolutionary approaches are proposed for global search to escape from traditional cross-randomization and to balance exploration and exploitation. Third, considering that the memetic algorithm (MA) framework is less efficient due to the randomness in the selection of local search operators, TAMA is proposed to balance the local and global searches. The first stage accumulates more experience for updating the surprisingly popular algorithm (SPA) model to guide the second stage operator selection and ensures population convergence. The second stage gets rid of local optimization and designs an elite archive to ensure population diversity. Fourth, five problem-specific operators are designed, and non-critical path deceleration and right-shift strategies are designed for energy efficiency. Finally, to evaluate the performance of the proposed algorithm, multiple experiments are performed on a benchmark with 45 instances. The experimental results show that the proposed TAMA can solve the problem effectively.
更多查看译文
关键词
distributed hybrid flow shop,setup time,multiple population,energy-aware,memetic algorithm,surprisingly popular algorithm
AI 理解论文
溯源树
样例
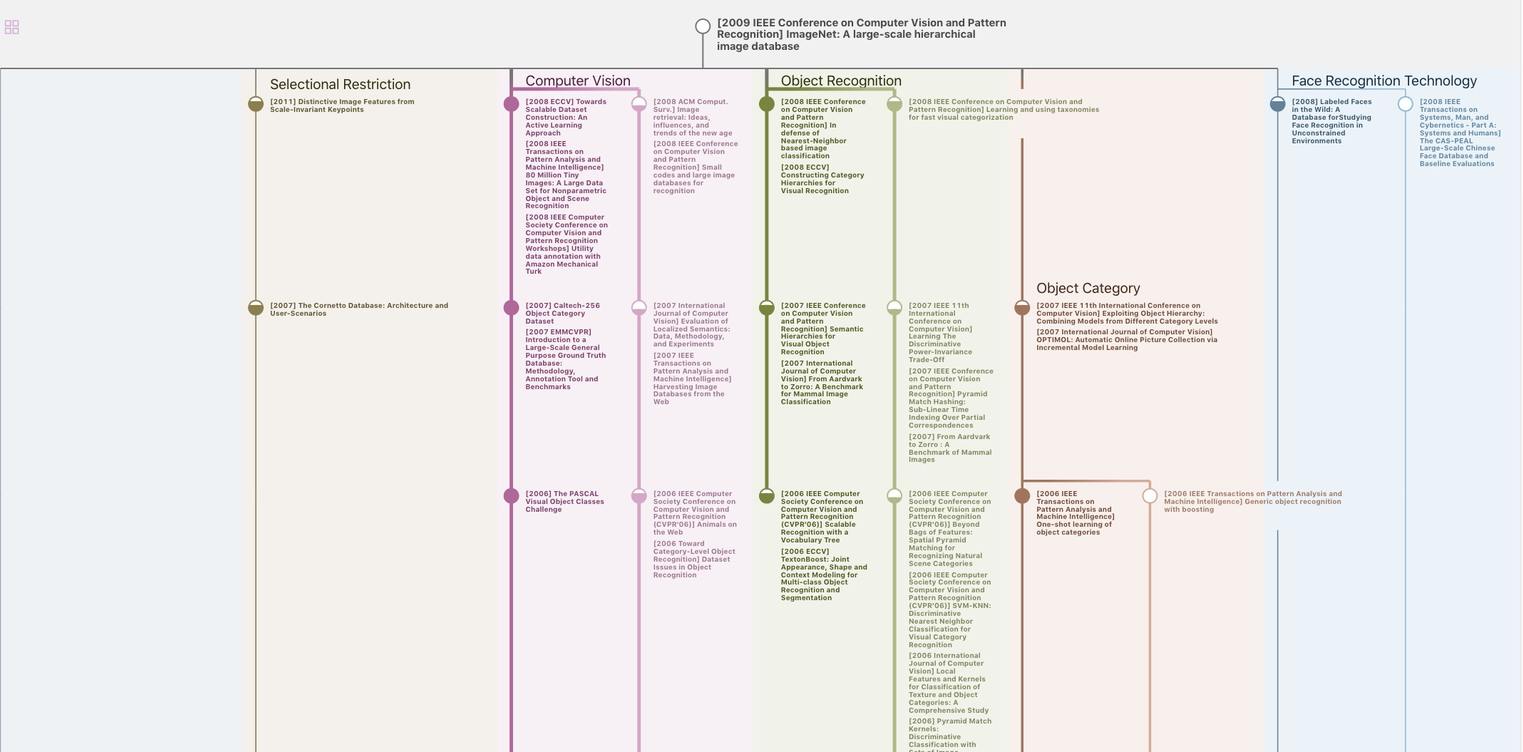
生成溯源树,研究论文发展脉络
Chat Paper
正在生成论文摘要