Conv-Basis: A New Paradigm for Efficient Attention Inference and Gradient Computation in Transformers
arxiv(2024)
摘要
Large Language Models (LLMs) have profoundly changed the world. Their
self-attention mechanism is the key to the success of transformers in LLMs.
However, the quadratic computational cost O(n^2) to the length n input
sequence is the notorious obstacle for further improvement and scalability in
the longer context. In this work, we leverage the convolution-like structure of
attention matrices to develop an efficient approximation method for attention
computation using convolution matrices. We propose a 𝖼𝗈𝗇𝗏 basis
system, "similar" to the rank basis, and show that any lower triangular
(attention) matrix can always be decomposed as a sum of k structured
convolution matrices in this basis system. We then design an algorithm to
quickly decompose the attention matrix into k convolution matrices. Thanks to
Fast Fourier Transforms (FFT), the attention inference can be computed in
O(knd log n) time, where d is the hidden dimension. In practice, we have d ≪ n, i.e., d=3,072 and n=1,000,000 for Gemma. Thus, when kd =
n^o(1), our algorithm achieve almost linear time, i.e., n^1+o(1).
Furthermore, the attention training forward and backward gradient
can be computed in n^1+o(1) as well. Our approach can avoid explicitly
computing the n × n attention matrix, which may largely alleviate the
quadratic computational complexity. Furthermore, our algorithm works on any
input matrices. This work provides a new paradigm for accelerating attention
computation in transformers to enable their application to longer contexts.
更多查看译文
AI 理解论文
溯源树
样例
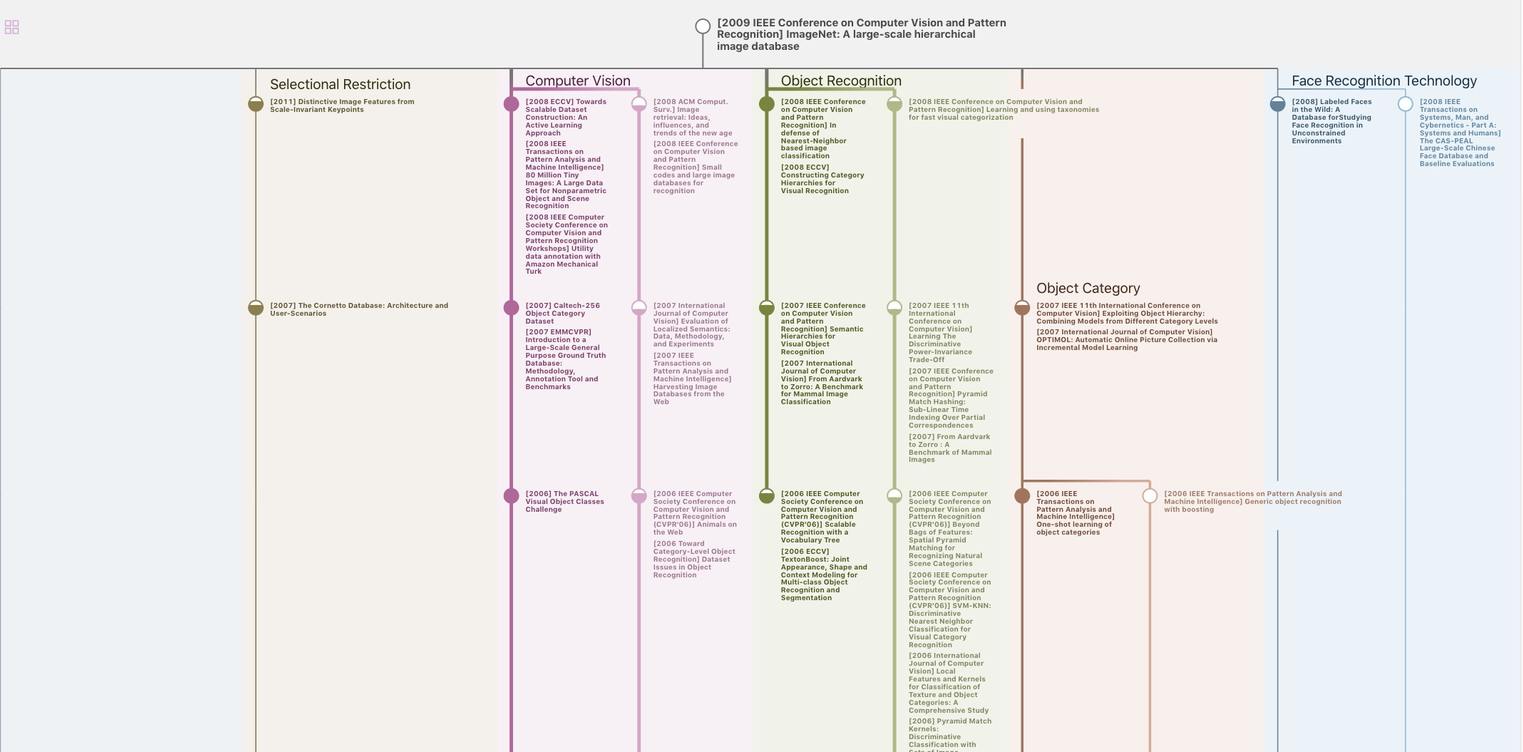
生成溯源树,研究论文发展脉络
Chat Paper
正在生成论文摘要