3D Point-based Multi-Modal Context Clusters GAN for Low-Dose PET Image Denoising
IEEE Transactions on Circuits and Systems for Video Technology(2024)
摘要
To obtain high-quality Positron emission tomography (PET) images while minimizing radiation hazards, various methods have been developed to acquire standard-dose PET (SPET) images from low-dose PET (LPET) images. Recent efforts mainly focus on improving the denoising quality by utilizing multi-modal inputs. However, these methods exhibit certain limitations. First, they neglect the varied significance of each modality in denoising. Second, they rely on inflexible voxel-based representations, failing to explicitly preserve intricate structures and contexts in images. To alleviate these problems, we propose a 3D Point-based Multi-modal Context Clusters GAN, namely PMC2-GAN, for obtaining high-quality SPET images from LPET and magnetic resonance imaging (MRI) images. Specifically, we transform the 3D image into unorganized points to flexibly and precisely express its complex structure. Moreover, a self-context clusters (Self-CC) block is devised to explore fine-grained contextual relationships of the image from the perspective of points. Additionally, considering the diverse importance of different modalities, we introduce a cross-context clusters (Cross-CC) block, which prioritizes PET as the primary modality while regarding MRI as the auxiliary one, to effectively integrate the knowledge from the two modalities. Overall, built on the smart integration of Self- and Cross-CC blocks, our PMC
2
-GAN follows GAN architecture. Extensive experiments validate our superiority.
更多查看译文
关键词
Positron emission topography (PET),low-dose PET denoising,multi-modality,point-based representation,context clusters,generative adversarial network (GAN)
AI 理解论文
溯源树
样例
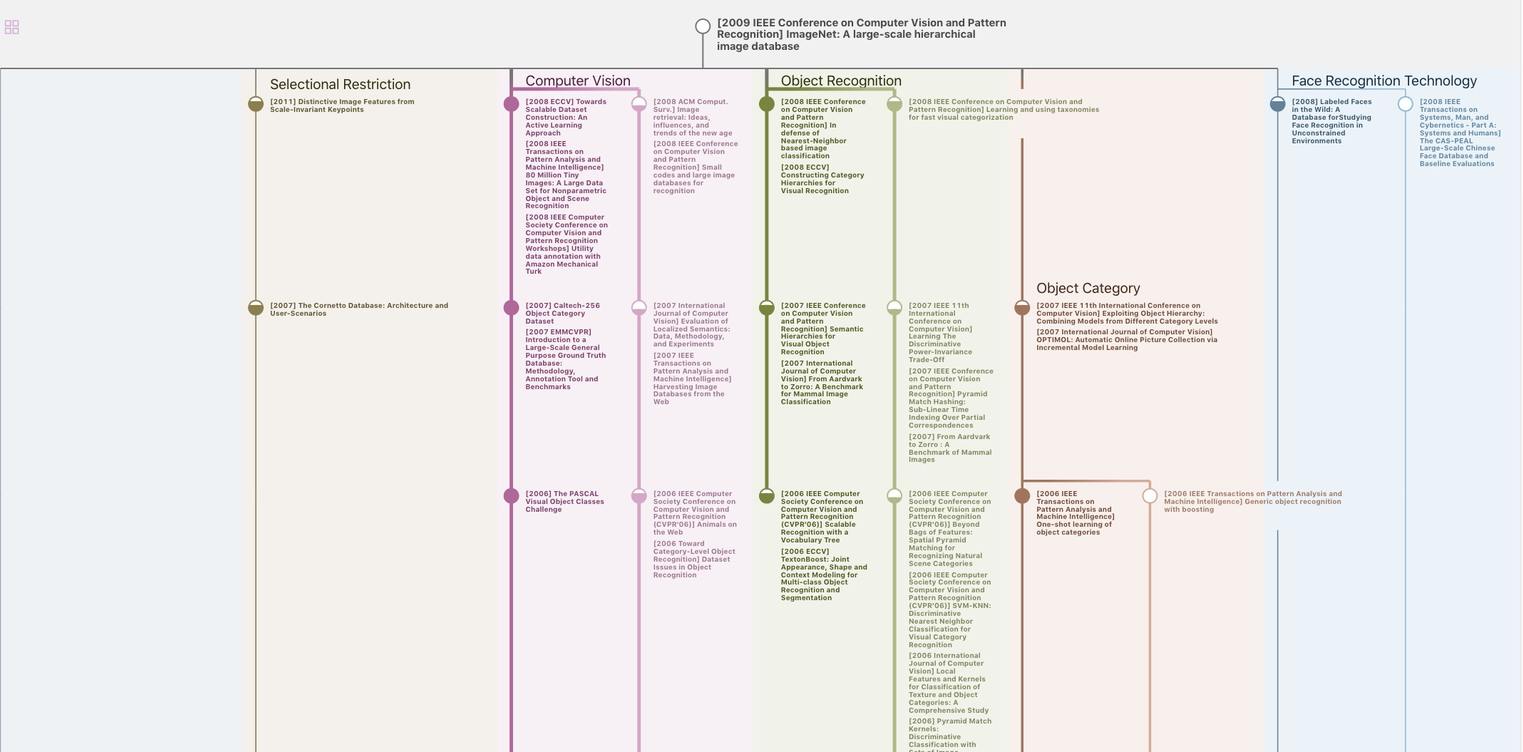
生成溯源树,研究论文发展脉络
Chat Paper
正在生成论文摘要