Higher-order embedded learning for heterogeneous information networks and adaptive POI recommendation
Information Processing & Management(2024)
摘要
Traditional POI recommendation results tend to be homogeneous due to data sparsity, and cannot adapt to the dynamic switching of user locations. Regarding the issues, we propose an adaptive Point of Interest (POI) recommendation algorithm that integrates the user social attributes and POI natural attributes. On the one hand, to fully utilize the complex node interactions and semantic information of heterogeneous networks, an effective higher-order embedding learning framework based on multi-head attention mechanism and multi-task shared features, called UI-HEHo, is presented. It divides hierarchical embedding learning into inter-order and intra-order aggregation to learn richer and more comprehensive representations. Based on UI-HEHo framework, a POI-user social attribute model is constructed to obtain the relational distance between interest points. On the other hand, a POI-natural attributes model based on user preference activity range prediction is constructed to further draw the influence between interest points. Furthermore, a tensor-based dynamic update scheme is designed to reduce the high cost of model updates. Finally, the Radviz graph is utilized to obtain the final recommendation results. Experimental results demonstrate our proposed approach significantly improves the POI recommendation performance, especially achieves 20.7% and 23.8% improvement on the Foursquare and Yelp datasets in terms of the newly defined diversity and novelty metrics.
更多查看译文
关键词
POI recommendation,Social relations,Geographical natural attribute,Heterogeneous information network,Multi-head graph attention
AI 理解论文
溯源树
样例
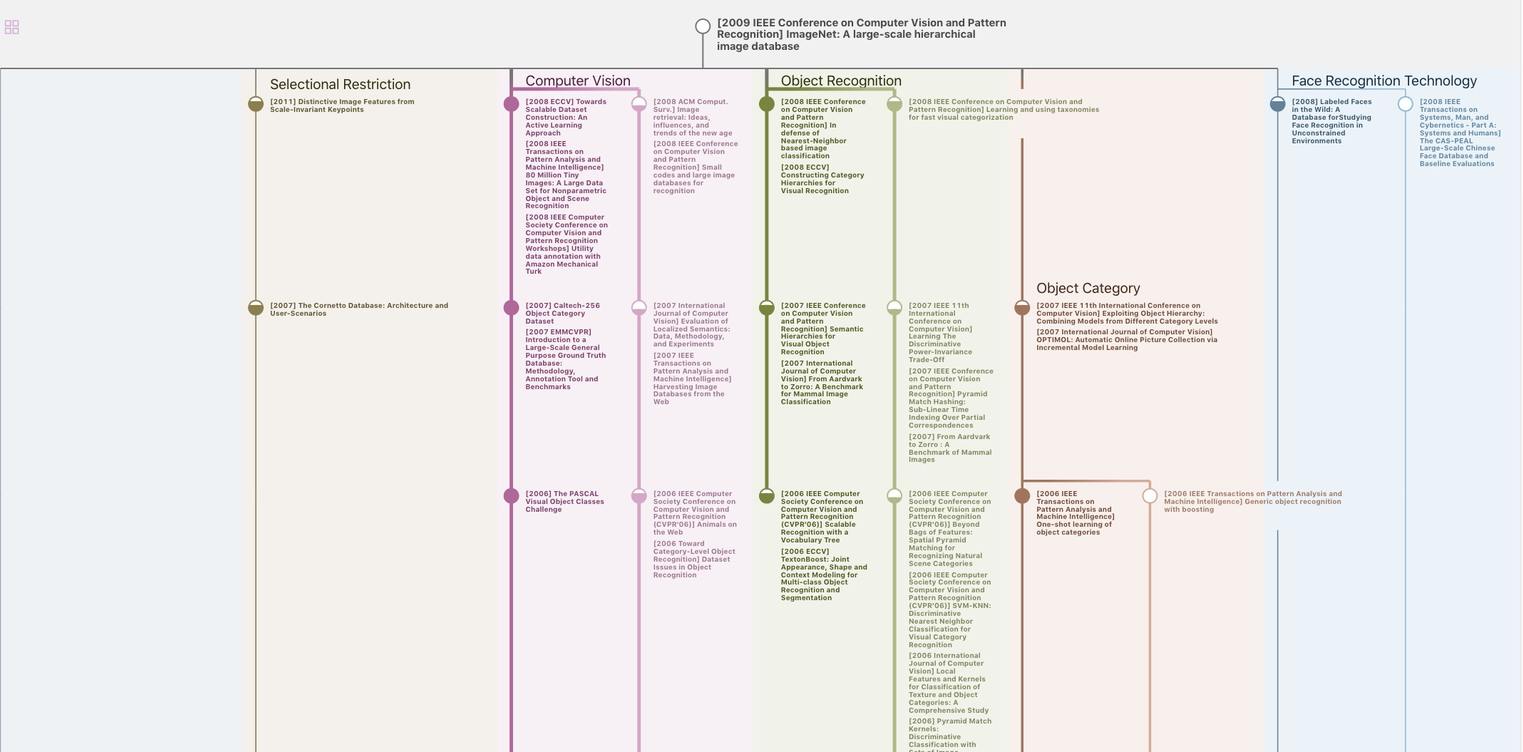
生成溯源树,研究论文发展脉络
Chat Paper
正在生成论文摘要