Combining machine learning and metal–organic frameworks research: Novel modeling, performance prediction, and materials discovery
Coordination Chemistry Reviews(2024)
摘要
Machine learning (ML) is the science of making computers learn and behave like humans, autonomously improving their learning by providing them with data and information through observations and real-world interactions. ML methods have significantly accelerated the progress of materials science research. Researchers can use ML frameworks to construct materials research models and design platforms to analyze and predict enormous data resources on materials. Metal-organic frameworks (MOFs), a rapidly developing coordination polymer in the last two decades, have become the most competitive candidate among thousands of porous materials with the application of numerous ML methods and models that have been successfully developed. This review offers an overview of how ML methods may be well-integrated with studying MOFs. It starts with a brief background on the concept and application of ML, points out the importance of various types of descriptors for ML modeling, and introduces several novel algorithms and models using ML in recent years. Then, we elaborate on the current research status of ML methods in MOFs performance prediction and materials discovery. At last, potential challenges are pointed out, and an outlook is given regarding the basic situation of ML-based MOF research. As various functionalized MOFs continue to be developed and applied in specific directions, ML will bring its advantages to the forefront in designing and discovering novel MOFs. Therefore, this review intends to provide readers with fundamental perspectives on the broad range of applications where ML is combined with MOFs research and expects to help enhance their study.
更多查看译文
关键词
Metal-organic frameworks,Machine learning,Novel modeling,Performance prediction,Materials discovery
AI 理解论文
溯源树
样例
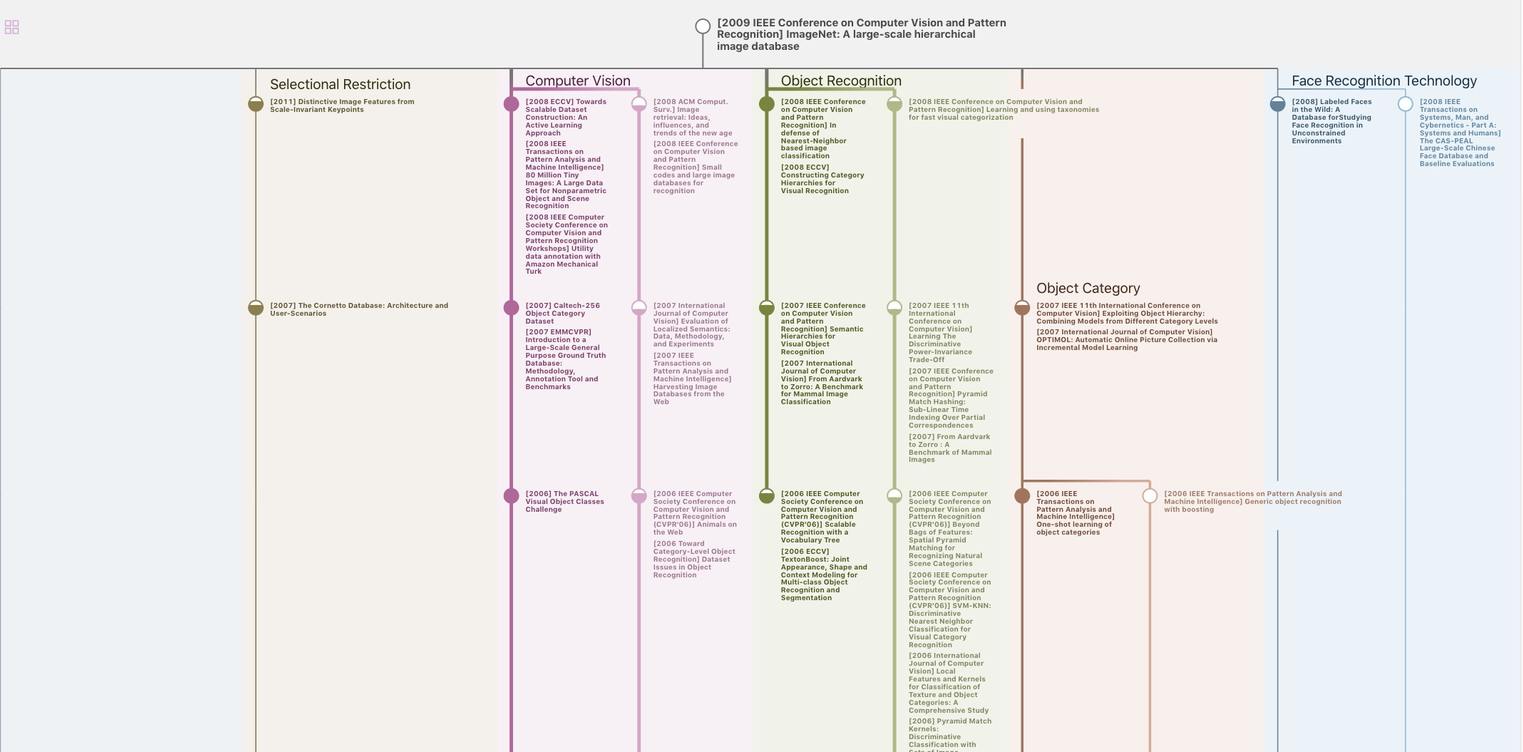
生成溯源树,研究论文发展脉络
Chat Paper
正在生成论文摘要