Train-induced vibration response reconstruction for bridge damage detection with a deep learning methodology
Structures(2024)
摘要
Sensor malfunctions in bridge structural health monitoring system (BSHMS) could cause the missing of measured data, reducing the accuracy of damage detection. It is of great significance to reconstruct the data of faulty sensors with measured data of well-functioning sensors for bridge structural state assessment. In this paper, a train-induced vibration response reconstruction approach for bridge damage detection is proposed. An enhanced deep densely connected neural network (ED-DCNN) introducing a multi-head self-attention mechanism and a skip connection is designed to reconstruct the missing bridge responses, aiming to improve the vibration correlation between the faulty sensors and good sensors and benefit the learning of temporal and spatial features of responses. Then to further validate the accuracy and reliability of the ED-DCNN, an alternate down-sampling residual network (AD-ResNet) is built to identify bridge damage with the complete responses, including the reconstructed ones and the measured, which extracts the structural damage features at different scales utilizing different types of residual blocks in an alternating manner. The efficiency and robustness of the two networks are comprehensively studied using the collected acceleration data from a heavy-haul railway bridge, considering different noise levels. The results demonstrate that under various conditions, the reconstructed responses match the real responses well in time domain, with a low RMSE below 0.4% and a high correlation coefficient of 0.9744, which indicates that the incorporation of a multi-head self-attention module effectively improves the construction of correlations between responses. Additionally, the natural frequencies of bridge are accurately identified from the reconstructed responses. In terms of bridge damage detection, taking both the measured and reconstructed responses as inputs, the identification accuracy obviously improves by 33.57% compared to cases without the reconstructed responses, further emphasizing an excellent reconstruction capability of the ED-DCNN.
更多查看译文
关键词
Data reconstruction,Deep learning,Self-attention mechanism,Structural health monitoring,Damage detection,Heavy-haul railway bridges
AI 理解论文
溯源树
样例
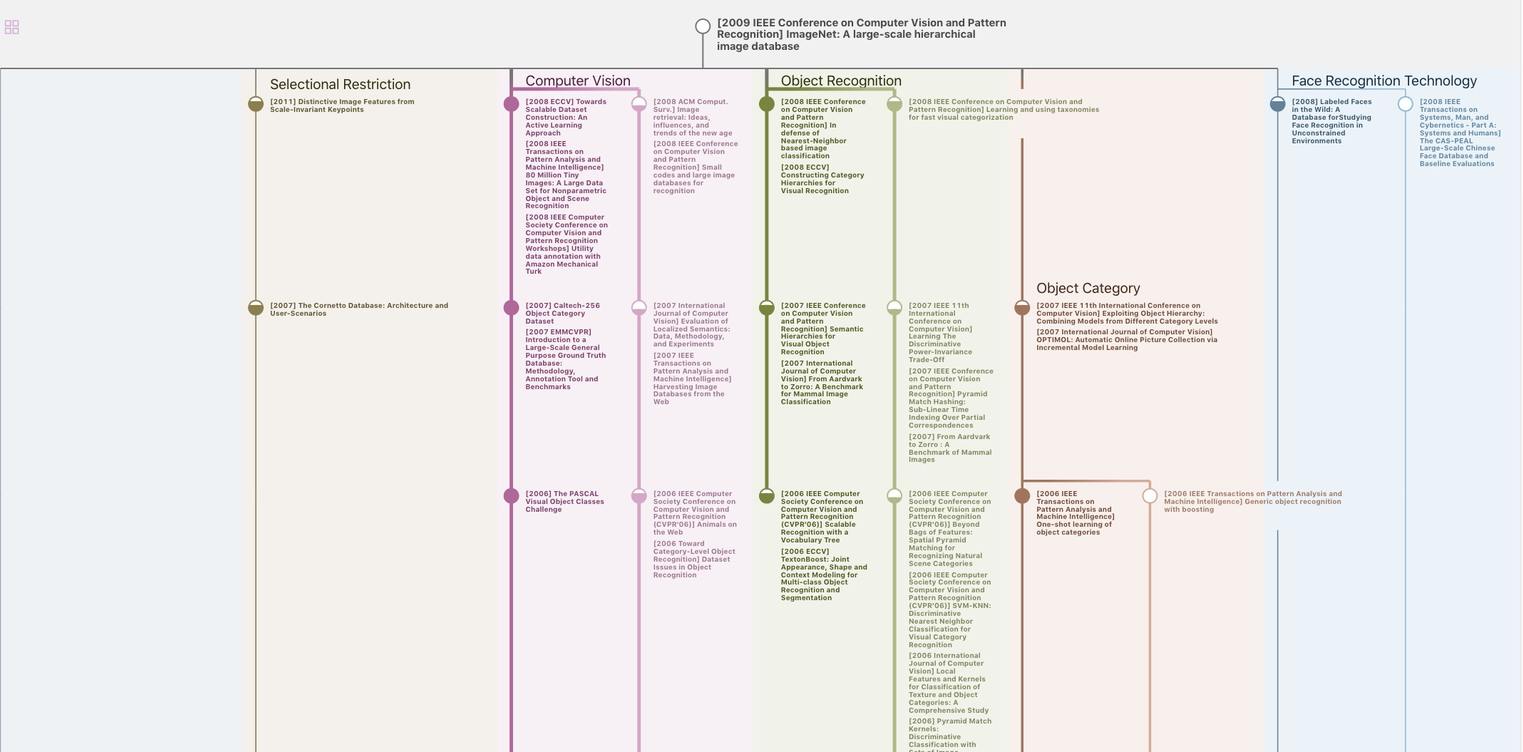
生成溯源树,研究论文发展脉络
Chat Paper
正在生成论文摘要