Refined STACK-CNN for Meteor and Space Debris Detection in Highly Variable Backgrounds
IEEE Journal of Selected Topics in Applied Earth Observations and Remote Sensing(2024)
摘要
In this work we present cutting-edge machine learning based techniques for the detection and reconstruction of meteors and space debris in the Mini-EUSO experiment, a detector installed on board of the International Space Station (ISS), and pointing towards the Earth. We base our approach on a recent technique, the Stack-CNN, originally developed as an online trigger in a orbiting remediation system to detect space debris. Our proposed method, the Refined Stack-CNN (R-Stack- CNN), makes the STACK-CNN more robust thanks to a Random Forest (RF) that learns the temporal development of these events in the camera. We prove the flexibility of our method by showing that it is sensitive to any space object that moves linearly in the field of view. First, we search small space debris, never observed by Mini-EUSO. Due to the limiting statistics, also in this case no debris were found. However, since meteors produce signals similar to space debris but they are much more frequent, the R-Stack-CNN is adapted to identify such events while avoiding the numerous false positives of the Stack-CNN. Results from real data show that the R-Stack-CNN is able to find more meteors than a classical thresholding method and a new method of two neural networks. We also show that the method is also able to accurately reconstruct speed and direction of meteors with simulated data.
更多查看译文
关键词
Neural network applications,space technology
AI 理解论文
溯源树
样例
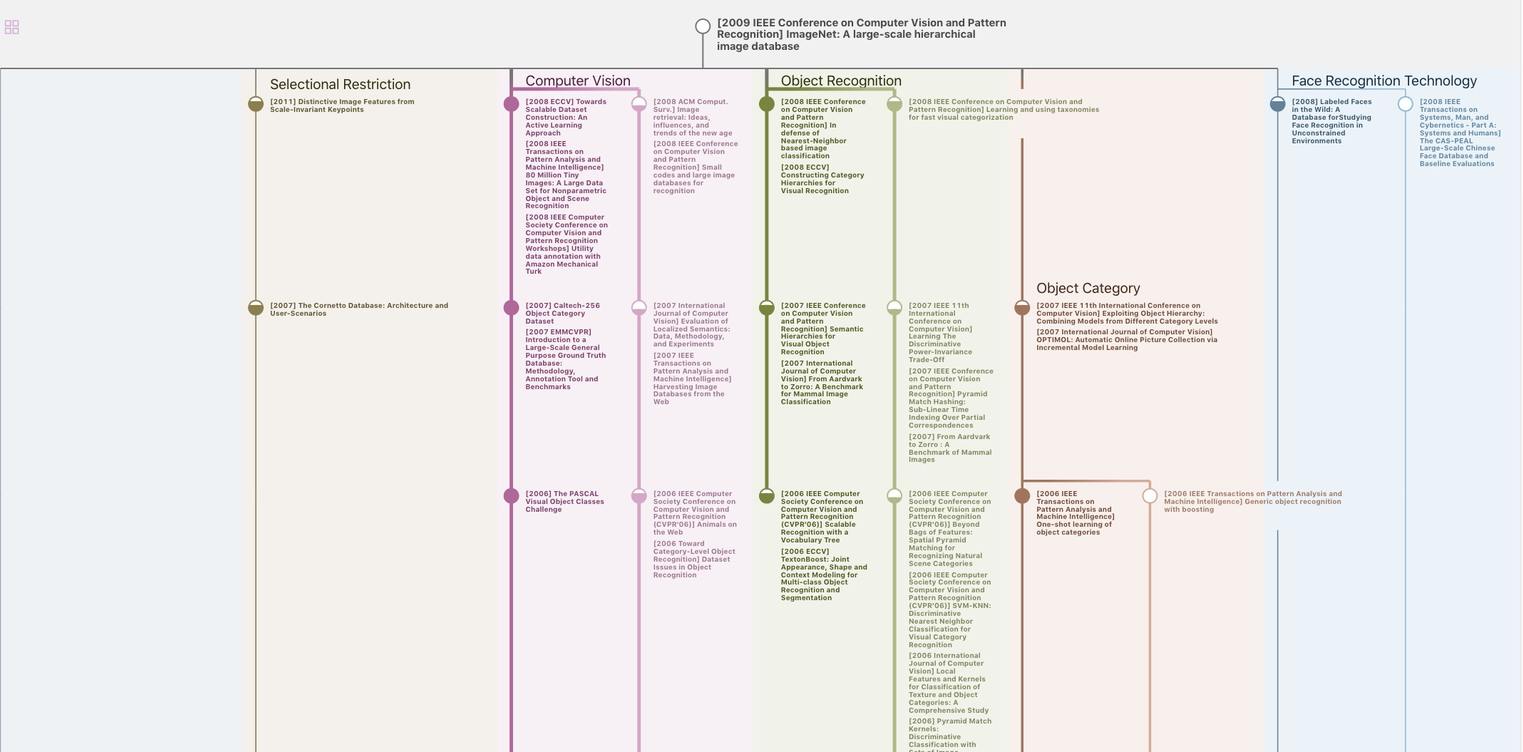
生成溯源树,研究论文发展脉络
Chat Paper
正在生成论文摘要