A Geometric Algebra-based machine learning method for Typhoon intensity forecasting
crossref(2024)
摘要
Abstract Typhoons, as highly destructive natural disasters, significantly impact society and economy, making accurate prediction of their intensity crucial. Artificial intelligence technologies, such as machine learning, have been extensively applied in the meteorological domain due to their advantages in processing large-scale datasets and learning complex nonlinear relationships. However, existing machine learning models for typhoon intensity prediction face issues like high complexity and parameter count, increasing computational demands and risking overfitting. This study leverages the advantages of geometric algebra for the unified expression and computation of multi-dimensional variables, introduces a geometric algebra-based convolutional neural network with spatial attention to enhance typhoon intensity predictions. This study employed typhoon data from the Northwest Pacific from 2000 to 2018. The results indicate that, compared to the traditional forecasting methods of the China Meteorological Administration (CMA), the proposed geometric algebra neural network model improved prediction accuracy by approximately 9% and reduced the root mean square error by about 5% compared to real-valued neural network models. Most importantly, while maintaining predictive performance, the geometric algebra neural network significantly reduced the number of model parameters, achieving up to a fourfold decrease compared to real-valued neural networks. This study offers new insights and technical advancements for using Geometric Algebra in analyzing to handle long-term, large-scale meteorological data.
更多查看译文
AI 理解论文
溯源树
样例
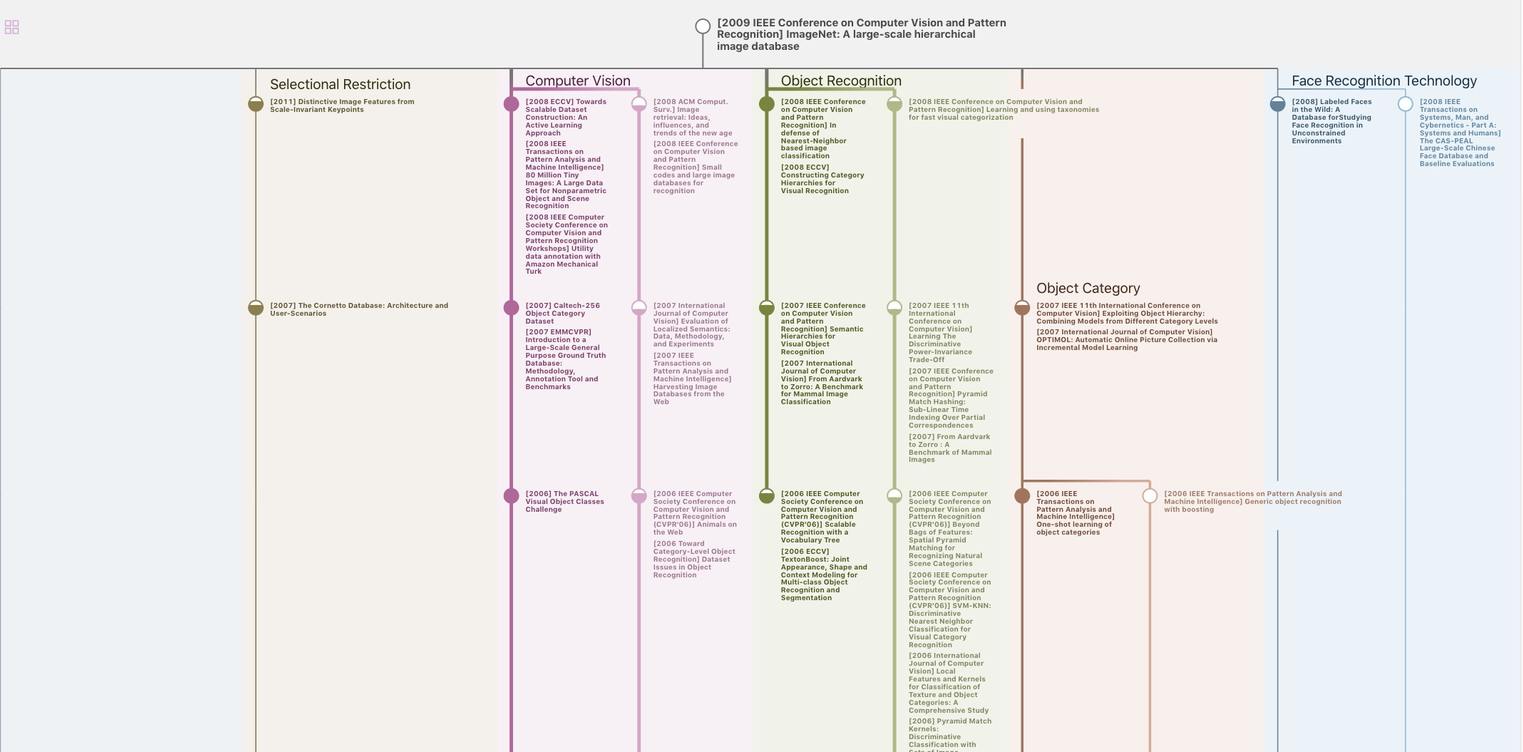
生成溯源树,研究论文发展脉络
Chat Paper
正在生成论文摘要