Exploration of Novel Neuromorphic Methodologies for Materials Applications
arxiv(2024)
摘要
Many of today's most interesting questions involve understanding and
interpreting complex relationships within graph-based structures. For instance,
in materials science, predicting material properties often relies on analyzing
the intricate network of atomic interactions. Graph neural networks (GNNs) have
emerged as a popular approach for these tasks; however, they suffer from
limitations such as inefficient hardware utilization and over-smoothing. Recent
advancements in neuromorphic computing offer promising solutions to these
challenges. In this work, we evaluate two such neuromorphic strategies known as
reservoir computing and hyperdimensional computing. We compare the performance
of both approaches for bandgap classification and regression using a subset of
the Materials Project dataset. Our results indicate recent advances in
hyperdimensional computing can be applied effectively to better represent
molecular graphs.
更多查看译文
AI 理解论文
溯源树
样例
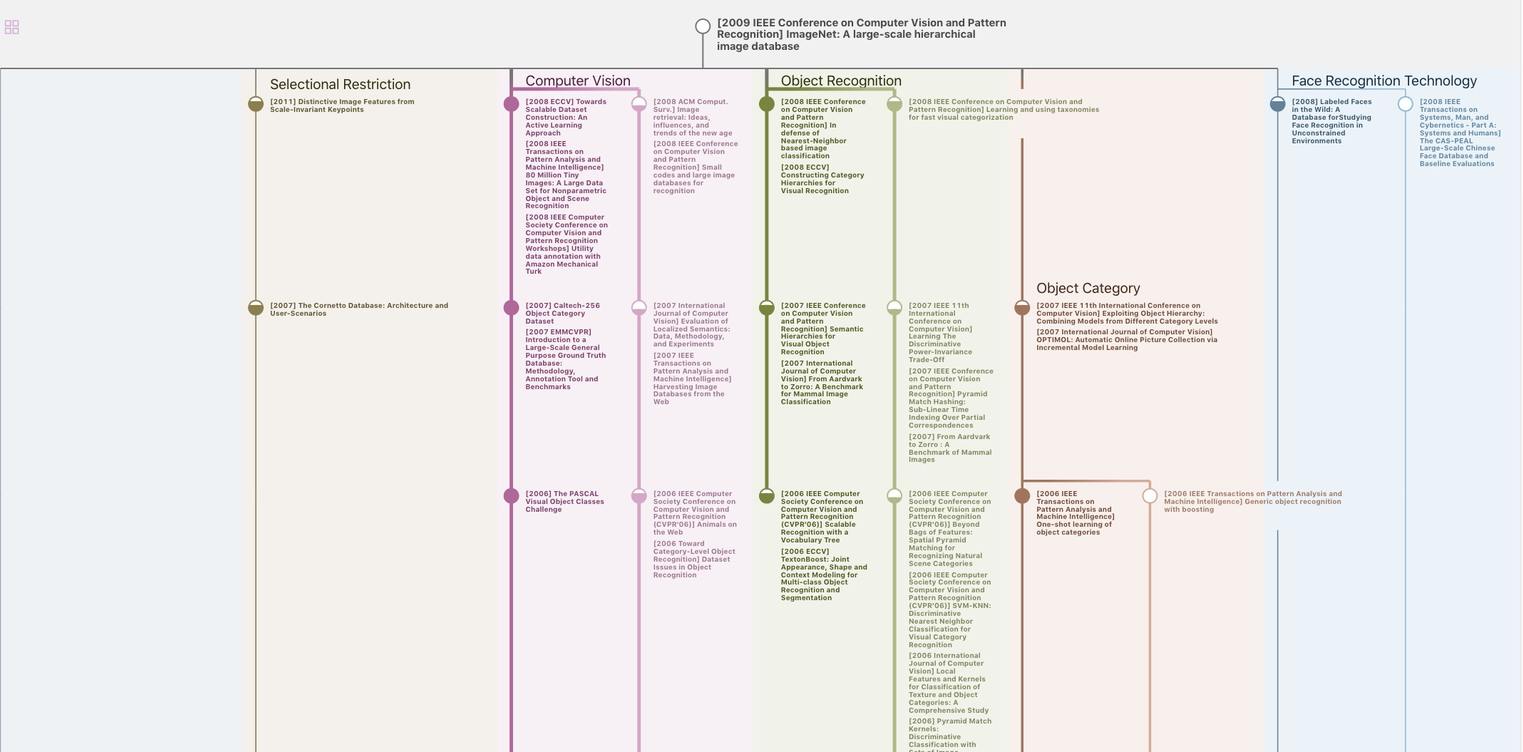
生成溯源树,研究论文发展脉络
Chat Paper
正在生成论文摘要