A Neuromorphic FTJ Model-Driven Design of Charge-Domain Synaptic Circuits and Spiking Neural Networks
2024 8th IEEE Electron Devices Technology & Manufacturing Conference (EDTM)(2024)
摘要
Spike Neural Networks (SNNs) operate in an event-driven manner and have excellent hardware compatibility, resulting in lower power consumption compared to Artificial Neural Networks (ANNs) that use pure software algorithms. In this work, the Spike Timing Dependent Plasticity (STDP) characteristics of Ferroelectric Tunnel Junctions (FTJs) were simulated. We used FTJ as an electronic synapse and proposed an SNN circuit that does not directly read the tunneling current, thereby avoiding the influence of FTJ’s self-capacitance on the conduction current. The functionality was verified in a small-scale array. Using the STDP data, the SNN algorithm was applied for image classification on the MNIST dataset, achieving an accuracy rate of 89.63%. This provides a promising solution for the full hardware implementation of SNNs based on FTJs.
更多查看译文
关键词
SNN,Synapse,FTJ,STDP,Digit recognition
AI 理解论文
溯源树
样例
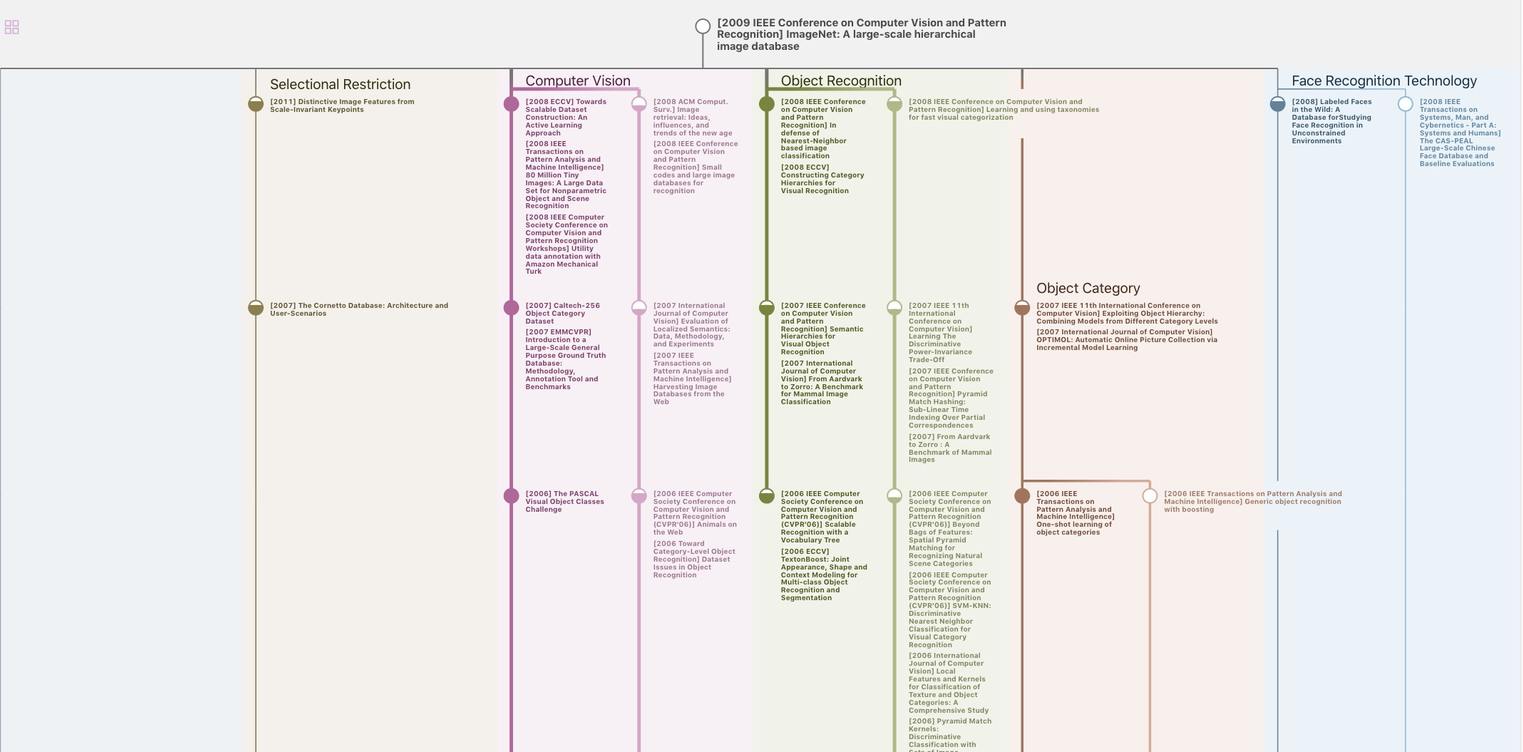
生成溯源树,研究论文发展脉络
Chat Paper
正在生成论文摘要