Leveraging small-scale datasets for additive manufacturing process modeling and part certification: Current practice and remaining gaps
Journal of Manufacturing Systems(2024)
摘要
Additive manufacturing (AM) provides a data-rich environment for collecting a variety of process data. These crucial data can be used to develop effective machine learning (ML) models for anomaly detection and process monitoring. These models are important for users to understand and identify process variations within the AM process, which are directly related to the formation of defects. However, many ML models are data hungry and require copious amounts of data for model training. Because of this, small to medium-sized manufacturers can be at a disadvantage, as collecting, processing, and analyzing large amounts of data can be cost prohibitive, leading to limited available samples. Despite this limitation, there are several commonly used approaches for improving the usability and effectiveness of small-scale data sets, including feature extraction, data augmentation, and transfer learning approaches. These approaches allow for either dimension reduction of the data through feature extraction, increase in training diversity and size via augmentation techniques, or the transfer and alignment of knowledge from one or more sources of data to improve a target model’s performance. This paper aims to explore these three popular techniques for small-scale dataset enhancement, provide insights into their use in AM, and discuss potential limitations and future research directions related to knowledge fusion and advanced learning techniques.
更多查看译文
关键词
Additive manufacturing,Data augmentation,Feature extraction,Machine learning,Process monitoring,Small-scale data,Transfer learning
AI 理解论文
溯源树
样例
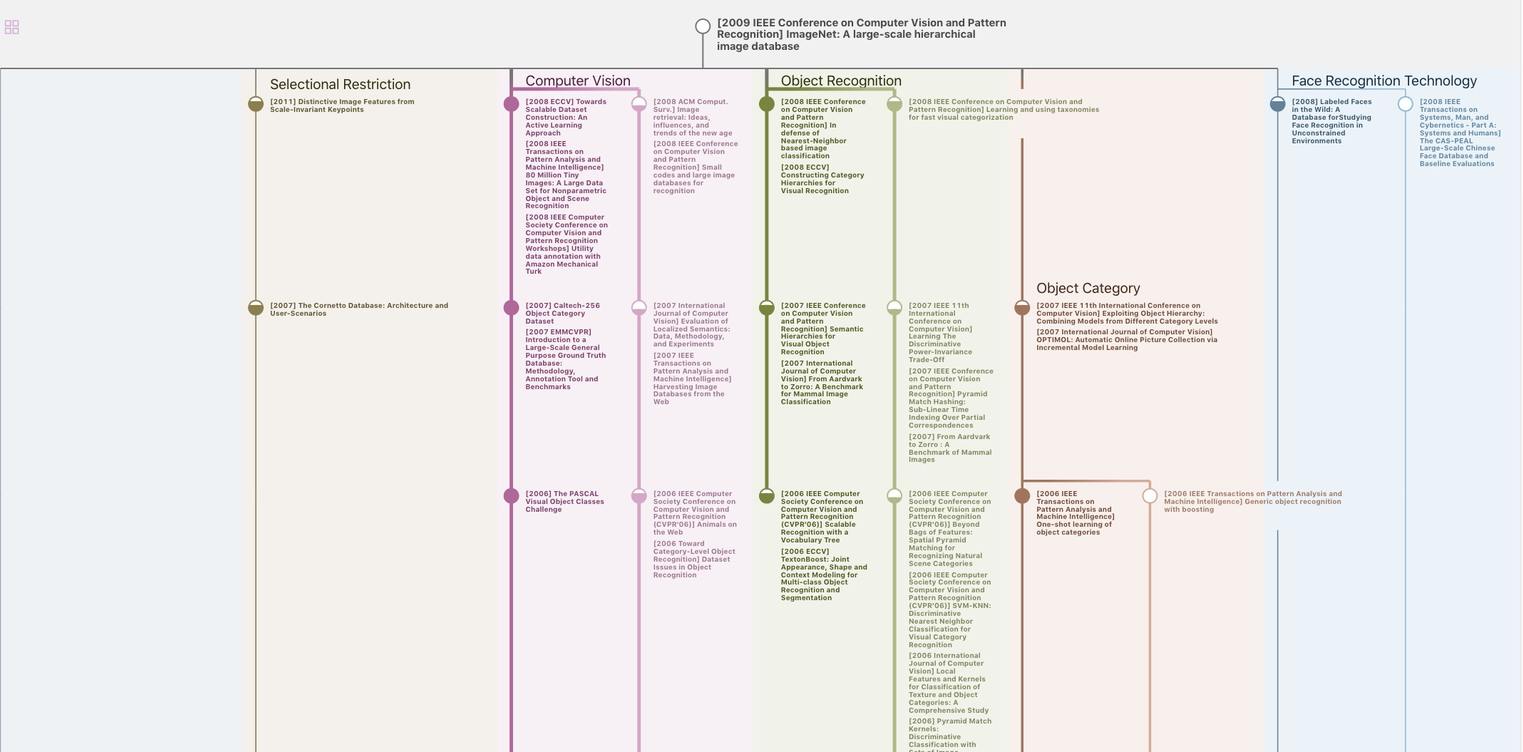
生成溯源树,研究论文发展脉络
Chat Paper
正在生成论文摘要